Spotify is better at picking your music than you are, but will they always be the best?
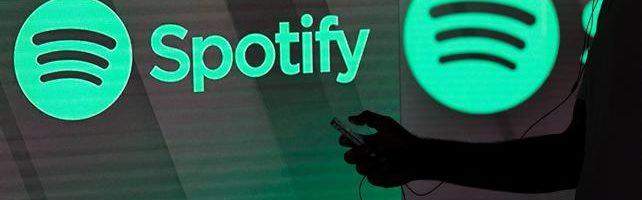
Machine learning at Spotify is a source of product differentiation in the music streaming industry.
Music streaming services today largely rely on a model of providing an extensive catalog of music of roughly 30M tracks for ad-free listening for about $10 monthly.[1] Thus, product differentiation is difficult to achieve, threatening the competitive positioning and pricing power of players in the industry.[2] To combat this, companies are implementing machine learning to add elements of personalization to increase their value proposition to customers and artists. Spotify, launched in 2006 and now the leader in the space with more than 35M+ paid users and 100M active users across 58 countries, has achieved its success thus far through best-of-kind execution of machine learning to develop personalized product features, such as Discover Weekly, that enable the product to deeply connect with its customers.[3] But the key question remains, can this competitive advantage last?
Source: Vidhya Murali, “Music Personalization at Spotify,” Power point presentation, September 15-19, 2016.
Whereas its competitors rely on a singular application of machine learning, Spotify has combined three predominant machine learning strategies in order to create its more satisfying discovery engine that powers the company’s product development (see above graphic).[4][5][6] First, Spotify utilizes collaborative filtering, leveraging implicit feedback, such as the stream counts of tracks, to develop a profile for each of its 100M+ users.[7][8] Next to understand the music component, Spotify relies on Natural Language Processing to pull text from blogs, news articles, et cetera, to understand what words and adjectives are associated with songs and artists.[9] The final component is the utilization of audio models to analyze raw audio tracks, studying aspects such as key, tempo, and volume, enabling Spotify to understand the fundamental similarities between songs.[10] The user-specific data is captured in the rows of Spotify’s vector system, with each row representing a user’s preferences, while these latter two components are captured in columns, representing song-specific information (see graphic below).[11] Through analyzing this user-song vector, Spotify can understand which users are similar to other users, as well as which songs are similar to other songs, and therefore match fitting songs and users across the platform.[12] With this system in place, Spotify can deliver highly effective customized music curation through its Discover Weekly playlist function, which has earned the company overwhelmingly positive acclaim from customers and consequently, driven growth and retention.[13]
Source: Vidhya Murali, “Music Personalization at Spotify,” Power point presentation, September 15-19, 2016.
Though Spotify has been successful thus far, continuous improvement with regards to its employment of machine learning techniques and applications is a priority amongst management, as the company seeks to maintain its competitive edge. One such near-term focus is to improve the quality and variety of content that informs the music-specific component of the analysis, which will further enhance the quality of the personalized playlists. Specifically, Spotify seeks to add analysis of lyrics and album-related images to their song and artist categorization.[14] A longer-term focus is to further ‘personalize’ the model, rather than relying on the existing technique of bucketing by ‘similarities.’[15] The closer Spotify can get to achieving true personalization, the more difficult it will be for competitors to close the product-quality gap. Another medium-term initiative is the expansion of its catalog into shows and podcasts[16]; management sees opportunity to leverage its existing machine learning platform to create similar value in the content curation of additional media forms.
There are further opportunities for management to extract value from its machine learning capabilities to further differentiate its product and maintain its leadership position in the music streaming space. In the near term, Spotify can leverage its deep understanding of its customers’ music preferences to help artists in the content creation process, by way of selling analysis to artists to provide a clearer understanding of the qualities and fundamental components that lead to song success amongst various listener groups. Because Spotify is a network, it is critical to provide value-additive offerings to both sides of the equation, the artists and listeners, in order to strengthen the network effect. Though it would be difficult for Spotify to succeed in the actual content creation on the music side of the business, over the medium-term, I see an opportunity for Spotify to leverage its deep knowledge of customers and their preferences to create its own content on the podcast and radio show front. As we’ve seen from Netflix, the creation of proprietary content is an effective method to increase competitive advantage via product differentiation, and thus reduce customer switching between platforms.
Though Spotify has been successful in its pursuit of differentiation via leveraging machine learning up to this point, the question of whether this advantage is sustainable is a key one. Will there always be opportunities for Spotify to further advance their algorithms and data quality, or is it inevitable that competition eventually catches up?[17] In terms of overall technological disruption, is there a world in which music streaming becomes obsolete or is music streaming driven by machine learning the final frontier for music consumption? (788)
[1] Trefis Team, “Spotify Has Seen A Big Rally, But Still Faces Some Challenges,” Forbes, June 27, 2018, https://www.forbes.com/sites/greatspeculations/2018/06/27/spotify-has-seen-a-big-rally-but-still-faces-some-challenges/#4a38e97d3ffb, accessed November 2018.
[2] Trefis Team, “Spotify Has Seen A Big Rally, But Still Faces Some Challenges,” Forbes, June 27, 2018, https://www.forbes.com/sites/greatspeculations/2018/06/27/spotify-has-seen-a-big-rally-but-still-faces-some-challenges/#4a38e97d3ffb, accessed November 2018.
[3] Vidhya Murali, “Music Personalization at Spotify,” Power point presentation, September 15-19, 2016, https://www.slideshare.net/vidhyamurali/music-personalization-at-spotify, accessed November 2018.
[4] Ying Qin, “A Historical survey of Music Recommendation Systems: Towards Evaluation,” Music Technology Area, Department of Music Research, Schulich School of Music, McGIll University, Montreal, Canada (April 2013). http://digitool.library.mcgill.ca/webclient/StreamGate?folder_id=0&dvs=1542151925966~558, accessed November 2018.
[5] “How does Spotify Know You So Well,” A Medium Corporation, October 10, 2017, https://medium.com/s/story/spotifys-discover-weekly-how-machine-learning-finds-your-new-music-19a41ab76efe, accessed November 2017.
[6] Nicholas Silipo, “Recommended For You: The Use of Machine Learning and Prediction Engines in Music Apps,” CUNY Hunter GSR, (Spring 2018).
[7] Vidhya Murali, “Music Personalization at Spotify,” Power point presentation, September 15-19, 2016, https://www.slideshare.net/vidhyamurali/music-personalization-at-spotify, accessed November 2018.
[8] Nicholas Silipo, “Recommended For You: The Use of Machine Learning and Prediction Engines in Music Apps,” CUNY Hunter GSR, (Spring 2018).
[9] Vidhya Murali, “Music Personalization at Spotify,” Power point presentation, September 15-19, 2016, https://www.slideshare.net/vidhyamurali/music-personalization-at-spotify, accessed November 2018.
[10] Vidhya Murali, “Music Personalization at Spotify,” Power point presentation, September 15-19, 2016, https://www.slideshare.net/vidhyamurali/music-personalization-at-spotify, accessed November 2018.
[11] How does Spotify Know You So Well,” A Medium Corporation, October 10, 2017, https://medium.com/s/story/spotifys-discover-weekly-how-machine-learning-finds-your-new-music-19a41ab76efe, accessed November 2017.
[12] Vidhya Murali, “Music Personalization at Spotify,” Power point presentation, September 15-19, 2016, https://www.slideshare.net/vidhyamurali/music-personalization-at-spotify, accessed November 2018.
[13] Nicholas Silipo, “Recommended For You: The Use of Machine Learning and Prediction Engines in Music Apps,” CUNY Hunter GSR, (Spring 2018).
[14] Vidhya Murali, “Music Personalization at Spotify,” Power point presentation, September 15-19, 2016, https://www.slideshare.net/vidhyamurali/music-personalization-at-spotify, accessed November 2018.
[15] Vidhya Murali, “Music Personalization at Spotify,” Power point presentation, September 15-19, 2016, https://www.slideshare.net/vidhyamurali/music-personalization-at-spotify, accessed November 2018.
[16] Vidhya Murali, “Music Personalization at Spotify,” Power point presentation, September 15-19, 2016, https://www.slideshare.net/vidhyamurali/music-personalization-at-spotify, accessed November 2018.
[17] E. Brynjolfsson and A. McAfee. “What’s driving the machine learning explosion?” Harvard Business Review Digital Articles (Jul 19, 2017.)
Great content! Your suggestion for Spotify to create their own music content based on machine learning insights is very intriguing. My main concern with their model is that their “understanding” of the customer’s music preference is solely based on the music provided by Spotify and thereby their ML models are being trained on a limited set of data which could potentially define a certain aspect of the customers’ music tastes but not the whole music profile of the customer. For example, Spotify’s international music database is still very limited compared to other music streaming platforms around the world which means Spotify’s customers could potentially be into different music genres and songs that Spotify’s ML models have no idea about. This could lead to an inaccurate grouping of the customer’s preference and new content created by Spotify may not be very successful. One solution could be that Spotify partners with international music platforms or expand geographically.
I believe there are absolutely opportunities for Spotify to continue advancing. This piece really drove me to think about machine learning’s intersection with the human psyche and human emotions. I agree with Petra’s point above, that the ‘preferences’ are limited due to the scope of songs the listener are exposed to. I also argue that Spotify seems to provide the sought-after model, and perceives to have a competitive edge, because there is no best alternative. Music is emotional and often creates an emotional connection between the listener and the song. Furthermore, human’s taste song by song is often particular. While they may enjoy a few specific genres, their enjoyed songs per album listed may vary dramatically. As machine learning progresses to better understand emotions and fickle human preferences, I think Spotify will have to adapt and incorporate these to keep any competitive edge.
This is very interesting and clearly depicts a situation where Spotify must stay on their toes to stay ahead of the competition. They have created a competitive advantage but I believe that it will be difficult to sustain for many of the reasons you described. As machine learning is limited to the data provided, I think there are opportunities to try and protect this advantage by international partnerships (to Petra’s point) as well as partnering with alternative music venues such as Soundcloud, that host user-uploaded content and would bring even more varied data to their algorithms.
Great piece Jeannie! I love music and I think that this is an interesting perspective on the future of music. At the core of their application of machine learning is the desire to improve the customer experience, as a result create sticky customers. As a Spotify user, I do appreciate the “Discover Weekly” feature, where I have found songs and artists that the algorithm has matched very well for me, but given the extremely personal and emotional aspect of music, I do wonder about the privacy concerns. One of the things that I have noticed from using “Discover Weekly” is that the algorithm seems to rank/rate recent music listened to highly, thereby recommending songs that are in line with your current emotional state. Having said that, I can imagine situations where the Spotify algorithm is picking up on a user’s emotional distress and amplifying that distress by recommending even more angst-filled songs. Similar to the issue that occurred when Target predicted that a teen girl was pregnant before her family knew – I can imagine among many different outcomes, a situation where Spotify could predict suicide or increase the likely of suicide through its song recommendations. I guess the question that I am trying to get to is how and when will the machine know that its recommendations though performing as expected are having negative outcomes beyond the limits of the application.
Very interesting piece! I am impressed with the advanced and multi-faceted approach that Spotify has taken to date to model and predict customer song preferences. Unfortunately, I wouldn’t be surprised if at this point they will only be able to achieve diminishing returns on efforts to further refine customer song recommendation engines, primarily because human song preferences are complicated and vary pretty wildly on a song-by-song basis in a way that is more art than science.
However, I believe the point you touched on briefly about Spotify beginning to explore applying machine learning to the other side of the market is a critical one. In addition to influencing the content creation process for artists as you mentioned, I think Spotify could also explore things such as recommending the optimal timing and locations for a particular artist to go on tour due to their popularity or lack thereof in certain geographies or demographics.
While I think Spotify is probably nearing the peak of the mountain of consumer music preference recommendations, they’ve only recently left base camp on the mountain of applying machine learning to aid in content production and value creation for record labels and artists.
This is very interesting, Jeannie! Echoing the comments above, I wonder if Spotify will eventually enter the larger media playground in order to remain competitive. From what I’ve read, Spotify has been struggling from a cash perspective for several years now, so I don’t think they have the capacity to invest in their own music content or video. My guess is that they will need to partner up with another firm to do so.
Nice post! I love spotify, so was happy to see that people picked this topic. To answer your first question, I think that the major streaming services (Apple Music and Tidal) will eventually catch up with Spotify’s algorithm for personalized playlists, etc since there aren’t large technological barriers to entry. However, long term Spotify will have the advantage because the company is starting to work closer with up and coming artists to distribute their music. Armed with powerful insights about fans, Spotify has an opportunity to leverage this information to almost become an internet based record label. The next wave of music will definitely be an interesting one!
It’s very interesting to see how Spotify combines several different machine learning strategies in order to produce customized-music playlists for its subscribers, and I think you did a good job at laying out their strategy and future plans in an organized way. One gripe I have had with Spotify is that I tend to listen to music playlists, such as classical and “deep focus,” while I’m reading or writing, in order to enable my concentration. Because of this, Spotify picks up on these habits and curates classical or ambient playlists for me in my Discover Weekly. I do listen to other music (i.e. that I like) with less frequency. I wonder how Spotify can pick up on some of these types of behaviors and filter through the “garbage in” inputs to make its music curation more effective ultimately.
I agree with Kay Holmes’ concerns about users’ expectations of privacy from the Spotify algorithm and potentially negative impact from public perception of Spotify knowing “too much” about one of its users. Spotify’s ad campaign last year featured billboards with machine learning insights, revealing the most-listened to songs of the year. Additionally, Spotify created a personalized “musical year in review” for each user based on their listening habits. Revealing to consumers the extent of the data a company collects about them can be risky business. Users may see the data as “creepy” rather than insightful. Indeed, there was considerable backlash when Netflix released similar tweets about holiday movie watching — while users may subconsciously know that all of their behavior is being tracked and captured into internal data, they don’t necessarily like being reminded of this or have a feeling of being “judged” for what content they are consuming. Our content consumption (whether it be music or TV shows) is a window into our inner feelings, almost a diary of sorts. In this way, Spotify must be very careful about its personalization being useful but being cognizant that users don’t like the feeling of giving up privacy.
Streaming licensed non-exclusive music content is a very low margin business, similar to TV / movie rental on-demand on iTunes or Amazon. Therefore, we would expect that the Spotify’s sole source of profit and competitive advantage in this market would be from its personalization algorithm and convenient UI. This is shaky and unclear how much users are really willing to pay for this — customers may prefer a similar service with all the same music content with a less advanced UI, for a lower price. It’s unclear that Spotify’s current business model is sustainable. As others have pointed out, its profits are slim and there isn’t much of a theoretical case to be made for margin expansion. A better strategy would be for Spotify to create its own original content, perhaps by signing mega hit artists like Taylor Swift or Drake to have their music only available on Spotify. This was similar to Beyonce’s strategy with Tidal, though it’s unclear if she was better off for this or not, since the lack of radio play or other distribution likely dampened the potential media buzz and word of mouth.
Very interesting article! As a frequent user of Spotify’s platform, I have been amazed by their ability to recommend content that I ultimately do end up really liking. That in turn has made me a very loyal customer. However, your question about the potential to sustain this competitive advantage is spot on – this algorithm, while novel now, is likely not difficult to replicate in due time. It is unlikely Spotify will stay one step ahead forever. However, their sustained competitive advantage can come from their collaboration with and insights from content producers, where they have the first mover advantage and thus an upper hand on the competition.
Thanks for an insightful post! I wonder if Spotify would work with artists and provides them information what characters of music would be popular will be popular among users, then it will actually compromise the artists’ own creativity and originality and lead to music styles converge to a mainstream standard just so to gain popularity. On your question about new frontier of music consumption, I wonder if the music consumption experience can be more immersive in the future. For example, combine AR/VR technology with music consumption to feel that the musician is performing in front of the users.
Great post, Jeannie! I absolutely think other streaming services, such as Apple Music, will catch up to Spotify. Currently, Spotify has more users, but Apple is adding new users faster [1]. As the tech advances, I believe Apple will be just as effective at recommending music.
[1] https://www.forbes.com/sites/careypurcell/2018/05/15/with-50-million-subscribers-apple-music-still-lags-behind-spotify-for-now/#3139fb59557f
Awesome article! I will agree with those who have commented above, based on my own experiences using Spotify, that the company has done an good job with their machine learning algorithms to this point. I have generally been impressed by the music recommendations that Spotify serves me. That said, I do not think that the use of machine learning to make these music recommendations is itself a huge edge anymore, as several of Spotify’s key competitors have followed a similar strategy. But, Spotify’s solid understanding of its customers’ behaviors and preferences provides an important foundation from which it can develop other products and services. Customers today place a premium on a personalized experience, and it seems to me that Spotify could be poised to deliver.