Smarter Cities: How Machine Learning can Improve Municipal Services in Chicago
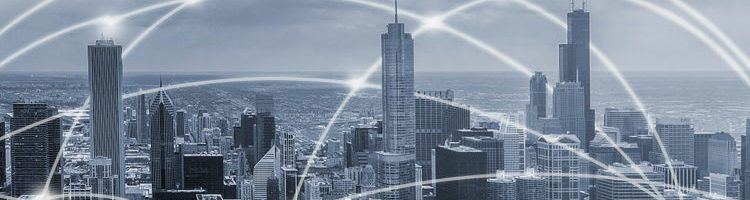
While machine learning is typically discussed in the context of major technology companies, city governments, institutions rarely thought of as innovators have begun to use machine learning to better understand the data they collect and use those insights to improve their processes.
Given their regulatory mandate, city governments collect an extraordinary amount of data ranging from real estate transactions to crime and education statistics. Unlike higher levels of government, which require legislation for most actions, local officials are well positioned to act quickly on the insights derived from their data via the many direct services that they provide.
Cities typically use data to inform their delivery of services, but machine learning provides an opportunity to go a step further than simple trend analysis as these algorithms can identify connections between seemingly independent variables among their massive datasets. These variables can then be used to make more accurate predictions about future citizen behavior, which drives smarter policy decision making.
Chicago leading the charge
Chicago’s local government has had success employing machine learning to improve its processes in areas of critical service delivery and in ways that more efficiently use City employees.
Earlier this year, Chicago’s Mayor, Rahm Emanuel, announced that gun violence was down 25% compared to the previous year, and down by over 55% in Englewood, a neighborhood that has historically struggled with violent crimes. A primary source of this improvement was the use of machine learning in predicting where and when violent crimes are likely to be committed. Mayor Emanuel summed up the strategy saying, “what you can predict, you can prevent”. Developed in conjunction with The University of Chicago’s Crime Lab, the algorithm makes predictions based on a wide variety of factors including gang activity, gun sales, time of day and even the weather. These predictions are then made available to each precinct so they can strategically deploy officers to high risk areas[1]. Critically, the City recognized that the algorithm would only be as successful as the data behind it, so they simultaneously deployed a technology called ShotSpotter, which collects a vast amount of information on every gunshot it detects instead of relying on when an officer is present or when a gunshot is reported by a civilian. Currently, ShotSpotter covers over 100 square miles across Chicago[2].
Chicago has also established a framework and governance structure designed to determine when machine learning should be employed to improve processes more broadly. In 2014, Tom Schenk was appointed Chief Data Officer to oversee data collection and predictive analytics efforts. In this role, Schenk created a 10-step “Applied Analytics Guide” and an accompanying set of criteria to identify and prioritize efforts given the time and resource intensity required to develop machine learning algorithms (Table 1). The City piloted this approach by creating a model that identifies restaurants likely to have a critical violation in random health & safety inspections, identifying 15% more violations than they would have otherwise[3],[4]. Chicago also launched its Open Data Portal initiative, which provides citizens with access to over 1,000 datasets, allowing individuals to create their own applications/algorithms and either sell those back to the government or launch them as their own independent companies[5].
New frontiers
City officials should continue to use machine learning to improve daily services, but they should also consider areas how predictive analytics could help enact more proactive policies. For example, if Chicago could to identify the neighborhoods most likely to gentrify, local officials could increase housing supply by facilitating the construction of affordable housing and enacting zoning changes that allow greater density, which would limit price increases and displacement. Given the lead time required in construction, officials are not currently able to act fast enough to mitigate these issues once an area begins to gentrify. Many data scientists are already looking into this opportunity and offer an opportunity for partnership. Ken Steif, a professor at the University of Pennsylvania and Alan Mallach from the Center for Community Progress have created “endogenous gentrification” projections for a number of smaller Midwest cities that predict neighborhood gentrification over 10 years before it occurs[6]. Private investors are also using AI to influence real estate investment decisions. Skyline AI is able to predict commercial real estate price trends across the country with an algorithm trained on over 100 datasets spanning 50 years[7].
Open Questions
While machine learning has its merits, any algorithm developed is subject to bias based on the data it is trained on (unless manually corrected). Policing is one area where this could manifest. If officers are biased to focus policing efforts on a given racial group, missing instances where other groups commit crimes, the algorithm built off of that policing data could reinforce that stereotype by selecting race as a predictor of crime. If that prediction drives greater police presence neighborhoods with specific racial demographics, the algorithm would become even more biased over time. The predictive factors used in these algorithms would also need to be kept private, as public knowledge could lead to “gaming” predictions (799 words).
[1] Greg Heinz. (April 10, 2018). Emanuel, Johnson laud drop in violent crime here. Crain’s Chicago Business [online] Available at: https://www.chicagobusiness.com/article/20180410/BLOGS02/180419986/emanuel-johnson-laud-drop-in-violent-crime-here [Accessed on 12 Nov. 2018].
[2] Shot Spotter website. (May 3, 2018). Chicago Expands Shotspotter coverage area to more than 100 square miles. [online] Available at: https://www.shotspotter.com/press-releases/chicago-expands-shotspotter-coverage-area-to-more-than-100-square-miles [Accessed on 12 Nov. 2018].
[3] Jessica Glover. (July 13, 2018). Analytics in City Government: How the Civic Analytics Network Cities Are Using Data to Support Public Safety, Housing, Public Health, and Transportation. Harvard Kennedy School – Ash Center for Democratic Governance and Innovation [online] Available at: https://datasmart.ash.harvard.edu/news/article/analytics-city-government [Accessed on 12 Nov. 2018]
[4] Harvard Kennedy School – Ash Center for Democratic Governance and Innovation website (February 7, 2018). Webinar Recording – Predictive Analytics: A 10-Step Guide. [online] Available at https://datasmart.ash.harvard.edu/news/article/webinar-recording-predictive-analytics-a-ten-step-guide-1220 [Accessed on 12 Nov. 2018]
[5] Achal Bassamboo and Tom Schenk. (June 20, 2016). How Open Data is Changing Chicago. Kellogg Insight [online] https://insight.kellogg.northwestern.edu/article/ow-open-data-is-changing-chicago [Accessed on 12 Nov. 2018]
[6] Tanvi Misra. (February, 17, 2017). Using Algorithms to Predict Gentrification. CityLab [online] Available at: https://www.citylab.com/solutions/2017/02/algorithms-that-predict-gentrification/516945/ [Accessed on 12 Nov. 2018]
[7] Catherine Shu. (July 31, 2018). Skyline AI raises $18M Series A for its machine learning-based real estate investment tech. Tech Crunch [online] Available at https://techcrunch.com/2018/07/31/skyline-ai-raises-18m-series-a-for-its-machine-learning-based-real-estate-investment-tech/ [Accessed on 12 Nov. 2018]
Wow, this seems like a very loaded topic but a highly critical one. Reducing gun violence by 25% is a very impressive achievement that creates huge amounts of value for the residents. By utilizing these methods though, I’m wondering how bias can really be ethically be kept out. If allowing a biased machine to run saves someone life, is it worth it? I’m also heavily reminded of the movie ‘Minority Report’, and how this appears to be trending in that direction.