Role of AI in lending – can machine learning bridge the gap between irresponsible lending and financial inclusion?
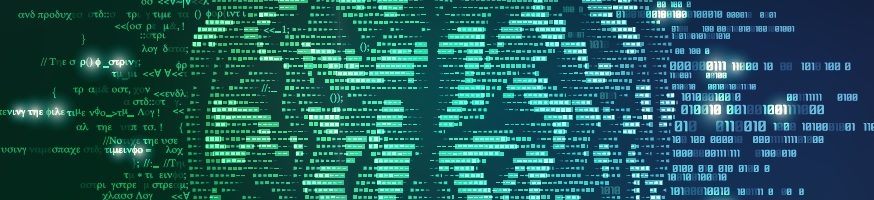
According to the PwC’s Financial services technology 2020 and beyond report, financial services is expected to be disrupted the most by modern technologies and banking is the industry with the highest share of leaders concerned about the speed of technological change [1].
Up until now high costs of compliance and numerous barriers of entry created by well-established players have kept fintech startups at bay, preventing them from making substantial headway into the market. However, fintech has found relatively fertile ground for development in developing economies were drive for innovation is complemented with high share of under-banked population. Notable examples include:
- Tinkoff Bank – online bank with no branches serving over 7 million customers in Russia [2]
- 4Finance – consumer lender with loans issued online exceeding EUR 6 billion across 13 counties in Europe [3]
- MyBucks – Africa-focused fintech operating 4 banks and 8 microfinance institutions providing wide range of financial services with a vision to become a fully digitized bank. MyBucks has issued EUR 519 million worth of loans to over 1.5 million customers [4]
- Mintos – Latvian startup turned into global online marketplace that has given retail investors the opportunity to gain exposure to alternative lending. Mintos has already funded over EUR 1.2 billion loans through its platform [5].
Examining digitalization of lending shows that the operating model has heavily skewed fintech products towards unsecured, small-size consumer loans, installments and credit cards. Fintech lenders differentiate themselves from traditional financial institutions by eliminating the red tape and offering a convenient, high-speed service to their customers. At the same time, online lending commands premium pricing compared to traditional lending channels, often leading to exorbitantly high effective interest rates. Lenders justify high rates by high-risk nature of their products, but the regulators are not always convinced and terms like ‘predatory lender’ and ‘loan shark’ are often used to describe fintechs. Growth of online lending has led to imposition of effective interest rate caps in a lot of developing countries – Baltics, Georgia, etc. In response, a lot of the fintechs started positioning themselves as responsible lenders and claiming social impact mantra.
There is clear value to fintech business model, since online lenders foster financial inclusion through providing access to capital for the parts of the population that have been previously excluded from the financial system. However, hidden fees and bullet repayments designed to maximize revenue do lead to a clear tension with responsible lending practices and create legitimate over-indebtedness concerns. This is where AI and machine learning algorithms have the potential to become game changers by resolving that tension through dramatically improving risk management capabilities and enabling companies to price their products fairly.
MyBucks has been at the forefront of developing AI in fintech and incorporating machine learning algorithms in their operations. The company views AI as instrumental to achieving their goal of reaching over 2 billion people that are excluded from the financial system today. Most of the fintechs employ some variation of proprietary credit scoring models that assess creditworthiness of their loan applicants, but these models are usually static and have to be adjusted or updated manually. In contrast, MyBucks has developed a credit scoring AI, “Jessie”, that is able to incorporate large swaths of relevant internal and external data. The algorithm learns to better predict probability of default through updating automatically to include newly available data and recalibrating in real time. The company has also implemented AI to proactively prevent fraud through monitoring for anomalous client behavior (“Dexter”). True to its digital vision MyBucks is in the process of piloting a number of other intelligent technologies to prevent client churn (“Charlie”), to proactively manage on-time repayment and maximize collections (“Maica”), to communicate with clients in advance based on intelligent predictions (“Donte”) and to evaluate affordability based on automatic income and expense calculator (“AMIE”) [4].
A lot of these cutting-edge technologies are still in pilot mode and their effectiveness remains to be evaluated. Analyzing high-level financials of the company indicates that despite 72% average loan book yield, operating expenses have dragged down profitability for the last three years [4]. MyBucks’ management is correct to focus on growth and developing scalable technologies in order to decrease operating costs, but the company also needs to strongly establish itself as a responsible lender. Piloting AMIE should be recognized as a step in the right direction, but the management needs to stress affordability more and make it a cornerstone of the operating model. Incorporating responsible lending practices into its AI will enable MyBucks to build upon a foundation suited for sustainable development in the long term.
What role will regulations play in terms of implementing AI based lending? Is AI based lending scalable to larger/corporate loans? (777 words)
References:
[1] PwC (2016). Financial Services Technology 2020 and Beyond: Embracing disruption. [online] Available at: https://www.pwc.com/gx/en/financial-services/assets/pdf/technology2020-and-beyond.pdf [Accessed 10 Nov. 2018].
[2] Tinkoff Bank (2018). Key Facts. [online] Available at: https://www.tinkoff.ru/eng/about-bank/key-facts/ [Accessed 10 Nov. 2018].
[3] 4Finance (2018). 4finance passes €6 billion online lending milestone. [online] Available at: https://www.4finance.com/press_release/4finance-passes-e6-billion-online-lending-milestone/ [Accessed 10 Nov. 2018].
[4] MyBucks (2018). Annual Integrated Report 2018. [online] Available at: https://corporate.mybucks.com/docs/MyBucksAnnualReport2018.pdf [Accessed 11 Nov. 2018].
[5] Mintos Marketplace (2018). Statistics. [online] Available at: https://www.mintos.com/en/statistics/ [Accessed 10 Nov. 2018].
Hey Giorgi, nice read! The topic is extremely interesting as many fintech startups are truly revolutionizing the traditional financial industry, making many of the commercial banks behemoths change their business model.
I would like to build on your essay, adding one example from Latin America. Prior to HBS, I worked in the biggest ecommerce and payments platform in the region. MercadoPago’s goal is to democratize the access of money in Latin American economies. As you pointed out in your essay, the main target for MercadoPago is people who are outside the traditional commercial banking scope because of their high-risk profiles. The company is charging extremely high interest rates while claiming to be inclusive with a huge social component on its mission at the same time. Even though I see your point of this paradox, I believe that the company is delivering truly positive results to the Latin American society. It started lending credits to both merchants and consumers, helping sellers boost sales and consumers get access to buy whatever they need in the ecommerce platform. The company is also launching its first money market fund to allow people to invest on the MercadoPago account and generate profits. It is also providing customer with lots of benefits and discounts in gasoline, restaurants and travel.
Having said that, I definitely believe that AI is not only the future of lending money, but also the future of assess risk of fraud. Alipay (Alibaba payments platform) is extensively using AI and facial recognition to determine identification and decide whether that person is the owner of the account to allow transactions. I think the main hurdle is the huge investment that AI may imply for a small and midsize companies such as MercadoPago in Latin America. However, the future is promising and an investment in AI can probably lead to huge cost savings in fraud and operations.