Risk management on the path to the machine learning prize at Workday
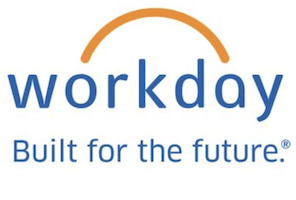
While machine learning creates a large opportunity for cloud software-as-a-service provider Workday to build solutions beyond their core enterprise human capital management and financial forecasting software, the prospect of designing and implementing complex algorithms presents significant risks that must be managed before an unsupervised implementation. This paper digs into the opportunity and risk mitigation tactics that Workday management should take over the next 3-5 years as they launch and scale these products.
According to Synergy Research Group, “spending on SaaS [software as a service] remains relatively small compared to on-premise software, meaning that SaaS growth will remain buoyant for many years.” [1] With this shift, Workday, Inc., which specializes in SaaS systems for human resources and financial management, will continue to accumulate large amounts of customer data on its platform. The rapid introduction of machine learning (ML) techniques, though, presents both large opportunities and significant risks for Workday that must be actively managed as Workday develops its future product roadmap.
First, the opportunity: Workday is poised to leverage ML to provide value to customers in new ways because of the company’s position as a cloud SaaS provider – it can train and test algorithms using data from its full customer base, a substantially larger dataset than a single on-site standalone customer. Workday, therefore, has the opportunity to build and offer ML-based products to its customers as add-ons to its human capital management (HCM) and finance platforms at a time when companies are looking to “tap into the technologies they need right away, and pay only for what they use,” according to a Deloitte study. [2] These product offerings would help customers predict root causes of key people issues like employee retention, talent management, and diversity, and make more accurate financial forecasts. [3]
However, the development of these products does present substantial risk for Workday, especially given their strong position in human capital-focused products. “With the spread of artificial intelligence to employment functions such as recruitment… bad inputs can mean biased outputs,” according to Wendy Hall, who led a review into artificial intelligence on behalf of the UK government. [4] Beyond bias, though, Workday will have to grapple with other challenges unique to ML. On the April 2018 earnings call, CEO Aneel Shusri pointed to customer data quality, stating that “many customers have plans to use [ML] in the next 12 to 18 months, once they get their data… in order. And a big part of that is getting clean data going forward.” [5] Given the importance of data quality to algorithmic predictions, Shusri’s comments suggest immediate adoption would risk output accuracy. Data “breadth” is also important for Workday to consider as it develops ML products. “These methods are great at determining how certain features of the data are related to the outcomes you are interested in. What these methods cannot do is access any knowledge outside of the data you provide,” according to Anastassia Fedyk. [6] Given Workday’s relatively narrow position as primarily an HCM provider, failure to properly scope ML algorithms could drive extraneous results in a real-world customer application.
Recent announcements by Workday indicate that management is beginning to execute on a strategy to integrate ML into the company’s core product. The company recently announced Workday People Analytics, which the company states will incorporate ML to drive pattern recognition in organizations and underlie an “augmented analytics” interface. Workday’s Prism Analytics product aims to be a “hub” for finance and HR data, both from inside and outside the organization (the latter through application programming interfaces with other cloud services), which helps solve for some of the data “breadth” concern related to deploying ML on a focused platform. Over time, Workday is aiming to deploy similar ML applications to augment decision making outside of the HCM product line. [7] Acquisitions this year like SkipFlag [8] and RallyTeam [9] have bolstered the engineering team and IP portfolio for a broader expansion into these ML applications.
For Workday management to successfully lead the way as a ML innovator among focused SaaS companies, management should also think about how to maximize customer transparency into the outputs of the algorithms that they deploy. While unsupervised deployment of complex algorithms would increase rollout speed and lower cost, they could have “limited visibility and [increased] risk of the ‘machine running wild.’” [10] Management should roll out these algorithms with a few key customers and maintain a tight interface between Workday data scientists and the customer team using the algorithms to inform decisions. Management should also leverage ML techniques on their platform to proactively flag and clean data on behalf of customers. Further, I would caution Workday against positioning their machine learning products as a definitive source of truth (e.g. “Workday People Analytics tells you what you need to know” [11]), and instead suggestion positioning them as a best in class tools to augment decision making.
But while Workday can take steps to prevent its ML products from being deployed appropriately in companies, it is ultimately up to their customers to either work with Workday to understand their algorithmic products, or use the “black box” appropriately (read: without blind faith). What further steps can Workday take in product development to ensure that their algorithms are used to their capability?
(799 words)
Endnotes
1 Synergy Research Group. “Microsoft Leads in SaaS Market; Salesforce, Adobe, Oracle and SAP Follow.” https://www.srgresearch.com/articles/microsoft-leads-saas- market-salesforce-adobe-oracle-and-sap-follow, accessed November 2018.
2 Deloitte.Insights. “State of AI in the Enterprise, 2nd Edition.” https://www2.deloitte.com/insights/us/en/focus/cognitive-technologies/state-of-ai-and-intelligent-automation-in-business-survey.html, accessed November 2018.
3 Schlampp, Pete. “Announcing Workday People Analytics: Leveraging the Strength of AI, Machine Learning, and Augmented Analytics.” Forbes.com, October 18, 2018. https://www.forbes.com/sites/workday/2018/10/18/announcing-workday-people-analytics-leveraging-the-strength-of-ai-machine-learning-and-augmented- analytics/#3373c2a977dd, accessed November 2018.
4 Ram, Aliya. “AI risks replicating tech’s ethnic minority bias across business.” FT.com, May 30, 2018. https://www.ft.com/content/d61e8ff2-48a1-11e8-8c77-ff51caedcde6, accessed November 2018.
5 The Motley Fool. “Workday, Inc. (WDAY) Q1 2019 Earnings Conference Call Transcript” https://www.fool.com/earnings/call-transcripts/2018/05/31/workday-inc-wday-q1-2019-earnings-conference-call.aspx, accessed November 2018.
6 Fedyk, Anastassia. “How to Tell If Machine Learning Can Solve Your Business Problem.” Harvard Business Review Digital Articles, November 15, 2016. ABI/INFORM via ProQuest, accessed October 2018.
7 Schlampp, Pete. Workday. “Announcing Workday People Analytics: Leveraging the Strength of AI, Machine Learning, and Augmented Analytics.” https://blogs.workday.com/announcing-workday-people-analytics-leveraging-the- strength-of-ai-machine-learning-and-augmented-analytics/, accessed November 2018.
8 Gagliordi, Natalie. “Workday buys SkipFlag to bolster machine learning capabilities.” ZDNet.com, Jan 16, 2018. https://www.zdnet.com/article/workday-buys-skipflag-to-bolster-machine-learning-capabilities/, accessed November 2018.
9 Miller, Ron. “Workday acquires Rallyteam to fuel machine learning efforts.” Techcrunch.com, Jun 6, 2018. https://techcrunch.com/2018/06/08/workday-acquires-rallyteam-to-fuel-machine-learning-efforts/, accessed November 2018.
10 Fedyk, Anastassia. “The Risk of Machine-Learning Bias (and How to Prevent It).” MIT Sloan Management Review Digital Articles, March 26, 2018, https://sloanreview.mit.edu/article/the-risk-of-machine-learning-bias-and-how-to-prevent-it/, accessed November 2018.
11 Schlampp, Pete. Workday. “Announcing Workday People Analytics: Leveraging the Strength of AI, Machine Learning, and Augmented Analytics.” https://blogs.workday.com/announcing-workday-people-analytics-leveraging-the- strength-of-ai-machine-learning-and-augmented-analytics/, accessed November 2018.