Rent the Runway Wants You to Clean Out Your Closet…Permanently
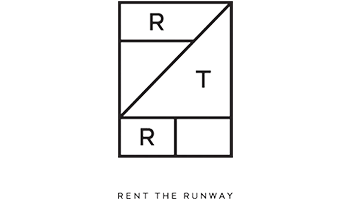
Rent the Runway aspires to be a “closet in the cloud,” and it is using data to make it happen.
If the meteoric rise of ecommerce ushered in the death of traditional retail, Rent the Runway is poised to be the nail in the coffin. The company’s value proposition of variety and affordability is perfectly aligned with societal shifts towards a more experiential, social media-driven, and sharing-focused economy. An emphasis on data and machine learning has driven RTR’s success thus far; however, the company has a grand vision for the future and needs to advance these capabilities to capitalize on the opportunity ahead. According to RTR internal research, the average American woman buys 68 new pieces of clothing a year, 80% of which are rarely worn [1]. RTR plans to solve this problem, envisioning a future in which closets contain the bare necessities and are supplemented with its “Unlimited” subscription model. The company would effectively become the “Netflix of fashion” [2]. To achieve this lofty goal, it must double down on its data capabilities.
The use of data and machine learning is important to RTR, because the company’s success is based on its understanding of two complex concepts – logistics processes and consumer preferences – and its ability to respond quickly. First, the profitability of the business is based on the unit economics of its inventory and how many times a dress “turns over” [3]. If a dress is sitting idle, it is not making money. Therefore, the company must continuously be iterating on its processes of shipping, dry cleaning, and inventory management to ensure low turnaround time [4]. In fact, its dry-cleaning warehouse is the world’s largest, processing 2,000 items per hour [5]. With each dress rental, RTR’s data systems get smarter, understanding what is coming in and out of the warehouse, predicting dry cleaning and repair time, prioritizing the order of events, and understanding when inventory is likely to go out of service [6]. Regarding the consumer, RTR must understand and appeal to changing preferences. Fashion is particularly fickle, with major trends shifting every few months. The ability to analyze its customer base, predict fashion trends, and manage inventory is key to acquiring and retaining customers.
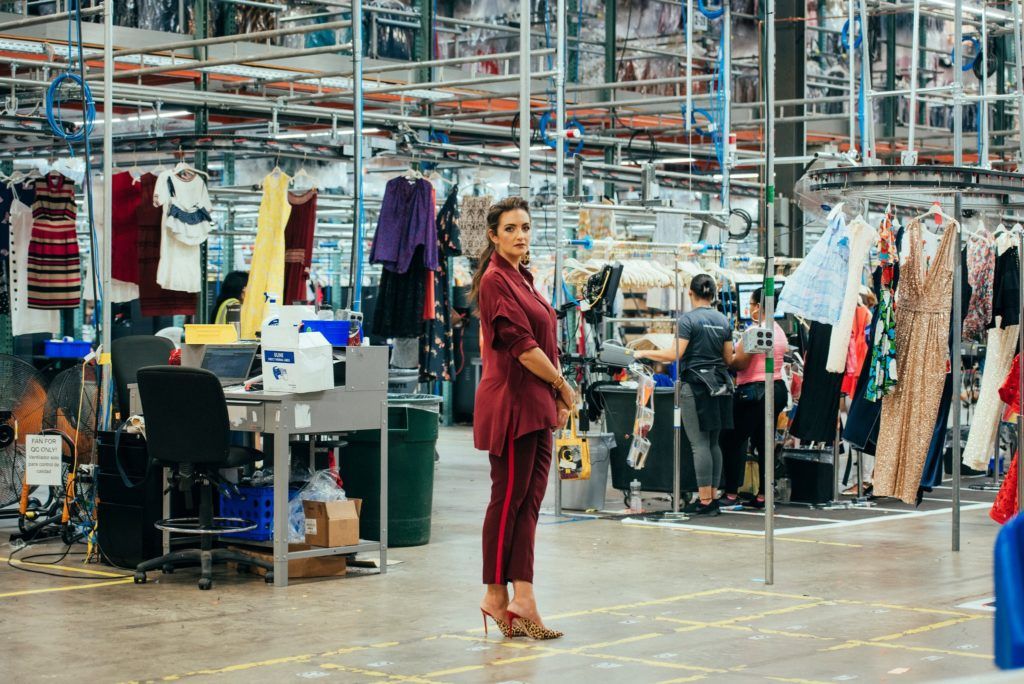
According to its founders, RTR is a “fashion company with a technology soul.” As the business has scaled, it has collected a wealth of information from its consumers and embraced data as a key tenet of the business [7]. First, RTR has access to what their consumers are searching for, filtering by, and favoriting. They then use this data to personalize the online or in-app experience, increasing the likelihood of a rental. The business “continually tweaks algorithms to identify pieces that customers might like and highlight them on the app’s home screen, similar to recommendations offered by Amazon and Netflix” [8]. Additionally, over 50% of consumers provide reviews after each rental, commenting on satisfaction, fit, and experience [8]. RTR applies these customer reviews to its inventory categorization, using an algorithm to crawl the text and understand which dresses are favored for specific events. Most importantly, RTR uses this data to inform its available inventory. Based on consumer searches, it preemptively understands when styles are changing and provides what is “in style.” In summary, CEO Jennifer Hyman states, “We’re collecting hundreds of thousands of data points each week: style, fit of the garment, what occasion you wore it to, what else is going on in your life, who are you? We’re creating one-to-one style personas for every user we have, which is helping us create an individual home page for you” [8]. Chief Analytics Officer Vijay Subramanian foresees taking this capability further in the future. Instead of scrolling through the app and seeing a few items you may like, he wants to target the consumer so effectively that the first two personalized suggestions will likely convert to rentals [7].
I agree, and I believe that RTR must further enhance its personalization to become a “closet in the cloud” [1]. RTR has created a new category and needs to convince consumers to forgo their purchases in favor of rentals. To be successful, it must find a way to limit the overwhelming choice available to users and reduce the time spent browsing the expansive inventory. With enhanced personalization, RTR may be able to mirror Stitch Fix’s capabilities, sending personalized packages on a bi-weekly basis based on the company’s knowledge of an individual’s fashion profile. Additionally, I foresee RTR becoming fully ingrained in the consumer’s life, accessing personal calendars, viewing work and social events, and providing specific items tailored to the consumer’s schedule. Data could become the new personal stylist.

What is the future of subscription fashion? Will the RTR Unlimited subscription see widespread adoption? Can fashion be data-driven, or will it always need a personal touch? (775 words)
[1] Alexandra Schwartz, “Rent the Runway Wants to Lend You Your Look,” The New Yorker, October 22, 2018, https://www.newyorker.com/magazine/2018/10/22/rent-the-runway-wants-to-lend-you-your-look, accessed November 2018.
[2] Kyle Jones, “How this entrepreneur launched the ‘Netflix of fashion,’ a company now worth nearly $1 billion,” Women in the World, January 5, 2018, https://womenintheworld.com/2018/01/05/how-this-entrepreneur-launched-the-netflix-of-fashion-a-company-now-worth-nearly-1-billion/, accessed November 2018.
[3] Steven Bertoni, “The Secret Mojo Behind Rent the Runway’s Rental Machine,” Forbes, August 26, 2014, https://www.forbes.com/sites/stevenbertoni/2014/08/26/the-secret-mojo-behind-rent-the-runways-rental-machine/#567ed4aa38bf, accessed November 2018.
[4] Sam Hollis, “How Rent the Runway built an $800 million business in a mom-and-pop industry,” September 17, 2018, https://jilt.com/upsell/rent-the-runway-logistics/, accessed November 2018.
[5] The Economist, “Rent the Runway is taking clothes-sharing mainstream.” June 7, 2018, https://www.economist.com/business/2018/06/07/rent-the-runway-is-taking-clothes-sharing-mainstream, accessed November 2018.
[6] Renee Boucher Ferguson, “Rent the Runway: Organizing Around Analytics,” MIT Sloan Management Review, March 24, 2014, https://sloanreview.mit.edu/article/rent-the-runway-organizing-around-analytics/, accessed November 2018.
[7] Deciding by Data, “Never Do Laundry Again: How Rent the Runway is Creating a “Closet in the Cloud” That Lets Data Choose Your Outfit,” June 1, 2018, podcast, https://decidingbydata.com/2018/06/01/rent-clothes-rent-the-runway-chief-analytics-officer-vijay-subramanian/, accessed November 2018.
[8] Sheila Marikar, “The Transformational Bliss of Borrowing Your Office Clothes.” The New York Times, October 12, 2018, https://www.nytimes.com/2018/10/12/business/rent-the-runway-office-clothes.html, accessed November 2018.
As a personal RTR user, I see there are three fashion paradoxes that leave open opportunities or might not be solved by the RTR model.
1. Although everyone wants to wear luxury designer clothes at a low price, no one wants others to know that they wear used clothes. Therefore, the social embarrassment of admitting wearing used clothes is the natural obstacle for users to recommend RTR to their friends.
2. The purchase pattern for fashion is that you may want to try something new, for example, a new style for a date, new dresses for an important party, etc. This fashion purchase behavior brings the divergence in consumer preference even for the same individual. However, machine learning is about improving convergence by predicting the preference of individual user with more historical data. There is a natural tension then.
3. Individual users want to get access to unlimited selections when they sign up the service. However, RTR has to increase the inventory turnover rate as much as possible. Therefore, a very common user experience is that when you finally selected your clothes, it’s already run out and was sent to someone else. The increase in inventory turnover rate is actually harming the user experience. This is because the fundamental network effect is not that obvious for the rental of a limited number of physical objects (clothes). In traditional tech companies, the network effect means that the marginal cost for serving one more customer is really low, because the digital content or infrastructure can be easily shared and used by many users at the same time. However, when it comes to physical stuff, one more user means decreasing probability of resource availability to other users.
Among the 3 issues I listed here, I think #3 is the most critical one. It challenges the fundamental hypothesis of the RTR shared economy model.
Thanks for such a thought-provoking article. I absolutely love the idea of having the tie-ins with a calendar so the company can proactively provide you a new wardrobe. Wedding coming up? You’re set! Not in the fashion space, I wonder if this will also spur even more creativity so that no two people have the same dress. You could essentially guarantee “you are the only one in Chicago getting this dress this year” (for a price).
I also think their retail approach is interesting. In cities like Chicago, there are showrooms, so that the physical brand component still exists. Of course, the machine learning may further reduce the need, but completing the online / AI portions with good old fashioned retail may strengthen their position.