Quantopian: the innovation funnel meets hedge funds

Boston-based fintech company Quantopian lets users test and create equity trading strategies, and deploys capital against crowd-sourced algorithms.
Quantopian: the hedge fund from the crowd
The world of hedge funds and proprietary algorithmic stock trading can seem murky, complex, and even dangerous to those unfamiliar with the inner workings of firms engaging in these activities. One Boston-based firm, Quantopian, has built a business model on the idea that trading algorithms can be sourced from anyone, not just highly-paid traders, and is betting on a crowd-sourced model to disrupt equity trading.
Operating Model
Quantopian was founded five years ago by financial-technology (“fintech”) entrepreneur John Fawcett, who previously sold a fintech software company in 2008 for $28M in cash and stock.[1] Heralding the Wall Street Journal’s reporting that “DIY’s newest frontier is algorithmic trading,” the company’s core value proposition is to link investor demand in the form of capital with algorithm supply in the form of crowd-sourced strategies. [2]
Quantopian’s community of 100,000 aspiring quantitative traders are equipped with the below tools to test market theses:
- Software tools leveraging the coding language Python
- Historical data from U.S. equity markets back to 2002
- Backtesting features to simulate how a given strategic would have performed in real market conditions
- Research tools to validate the strength of a given algorithm, and
- Access to capital to trade.
Quantopian publishes its selection criteria and author upside on its website. Successful quants will create strategies with five key attributes[3]:
- Low exposure, with a market beta of between -0.3 and 0.3
- Consistent returns given risk profiles of a Sharpe ratio consistently over 1.0
- Active portfolios with a target turnover rate of 12 and 500 times a year
- Low correlation to other selected authors
- Strategic intent based on market trends and economic drivers
At the top of the innovation funnel, Quantopian offers incentives and competitions for users to submit ideas. Any author can tie their code to their bank account and trade their own money. The company then acts as a gatekeeper by evaluating certain algorithms for six months, much like Threadless does with user submissions. If backtesting and live simulations prove successful, the company may deploy capital to trade the company’s or its limited partners’ (currently, Point72 Ventures) capital, in which case the author will receive a portion of all returns.[4] To be clear, authors own their algorithm’s IP.
The operating model relies strongly on providing powerful software and relevant datasets in order to attract coders, which drastically lowers the cost to attract hedge fund-level talent to create profitable trading strategies. Andreessen Horowitz investor Alex Rampell, a Quantopian board member, thinks of Quantopian “as the next-generation Blackrock.”[5] While the two firms have slightly divergent amounts of assets under management, Quantopian may have more favorable tailwinds.
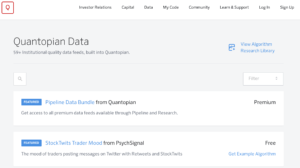
Strategy & Business Model
The typical hedge fund business model relies on highly-paid and intelligent analysts to create a limited set of strategies and algorithms with which to trade capital raised from limited partners. Hedge funds typically charge a 2% management fee and 20% of the positive returns.
Quantopian disrupts this model through crowdsourced strategies, very low labor costs (nearly zero) with a different fee structure, and an open innovation funnel.
In the HBR article Strategy and the Internet, Michael Porter argues that the structural attractiveness of an industry relies on five forces, including intensity of rivals, barriers to entry, and threats of substitute products (see below figure). In this industry, Quantopian’s model proves to drastically lower the barriers to test and create trading models by democratizing access to anyone with Internet access and basic coding skills. In addition, trading offerings are more difficult to keep proprietary, as anyone can build similar or identical trading models using the same available data to pressure test market theses. The variable cost of experimenting with a new algorithm is close to zero under this model; at a traditional hedge fund, the firm may need to invest significantly in a trading strategy, and demonstrate its success, before it has the ability to raise capital from LPs. Porter ultimately argues that “Internet technology provides better opportunities for companies to establish distinctive strategic positionings than did previous generations of information technology.”[6]
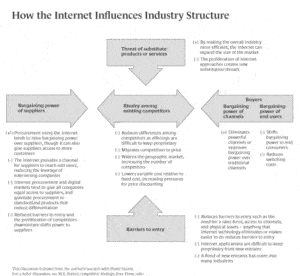
Additional Steps & Conclusion
Quantopian is well-positioned to expand its offerings. The company can further democratize capital raising, or invest training and development to its top producing authors. Finally, the company can act as a recruiting tool for hedge funds.
The classic hedge fund model is under siege, as investors question current fee structures and withdraw money from highly paid firms that struggle to beat market indexes.[7] Quantopian developed a distinctive operating model that engages a broad community through the Internet to compete with hedge fund traders, and thus has the impact to fundamentally change the economic drivers within finance and technology. (797 words)
[1] Advent Completes Acquisition of Tamale Software. 2016. Advent Completes Acquisition of Tamale Software. [ONLINE] Available at: https://www.advent.com/about-us/newsroom/pressreleases/advent-completes-acquisition-of-tamale-software. [Accessed 18 November 2016].
[2] Austen Hufford. 2016. Algorithmic Trading: The Play-at-Home Version – WSJ . [ONLINE] Available at: http://www.wsj.com/articles/an-algo-and-a-dream-for-day-traders-1439160100. [Accessed 18 November 2016].
[3] Quantopian . 2016. Quantopian . [ONLINE] Available at: https://www.quantopian.com/fund. [Accessed 18 November 2016].
[4] Bloomberg.com. 2016. Andreessen Horowitz, Point72 Invest in Crowd-Sourced Quantopian – Bloomberg. [ONLINE] Available at: http://www.bloomberg.com/news/articles/2016-11-14/andreessen-horowitz-point72-invest-in-crowd-sourced-quantopian. [Accessed 18 November 2016].
[5] Ibid.
[6] Harvard Business Review. 2016. Strategy and the Internet. [ONLINE] Available at: https://hbr.org/2001/03/strategy-and-the-internet. [Accessed 18 November 2016].
[7] Ft.com. 2016. Investment: Rise of the DIY algo traders. [ONLINE] available at: https://www.ft.com/content/0a706330-5f28-11e6-ae3f-77baadeb1c93 [Accessed 16 November 2016].
Really interesting idea. If the amateur quants’ IP is protected and truly their own, I wonder how Quantopian will be able to prevent top ideas/coders from being poached from their platform by established funds. In this case, Quantopian would be providing the infrastructure for talent discovery and development for hedge funds, effectively becoming their outsourced R&D and recruiting arm, with little compensation in exchange for top performers (with only middling ideas who are not poached left on the platform). This would only be a problem if the platform was very successful, and if potential LPs discovered and actively poached amateur quants (which I’d imagine could be a violation of the terms of partnerships between potential LPs and Quantopian), or if the amateur quants actively marketed their success (which maybe Quantopian has assessed to be less of a risk).
Wow! This sounds like it could be a disruption if it were easy to come up with investment strategies that worked better than the paid experts. They say that the wisdom of crowds is greater than a few experts, but I’m not sure it applies in this case. I would be interested to know how many in the crowd can create better algorithms than the investment gurus. What is the fee structure? Is that taken into account in ranking the algorithms? Does the ranking prioritize those algorithms that make the platform company more money?
Great post (and a little bit scary for the financial analysts among us)! I agree that more sophisticated and automated data analysis can certainly improve the odds of “beating the market,” but I think human diligence and investment judgement will also continue to be important. For example, analysts can seek out additional data points that are not currently feeding the algorithm (e.g. they can have dozens of customer calls to determine the desirability of a product launch or other events that are important to a stock). Also, there are many examples of startups that don’t have historical comparables that the algorithm can compare the start up to. In these cases, investors have to go with what their gut tells them when projecting how well consumers will accept the new product.
MM 2018 raises a very interesting point about the threat of amateur quants being poached by other hedge funds. But “Keith” points out in his conclusion that Quantopia could have a future as a recruiting tool, not just as a fund. And, as “Keith” points out elsewhere, Quantopia’s business model as it stands also includes providing “access to capital to trade” to amateur quants.
Those details hint at three possible business models for Quantopia: As a fund that invests money based on algorithms licensed from amateurs who use the service; as a recruiting tool for other funds; and as a connection mechanism between amateur traders and capital sources. Do you think those three potential models can work together simultaneously? Or does Quantopia have to choose one in particular if it’s going to succeed?