Project Maven: Machine Learning in the Military Target Selection Process
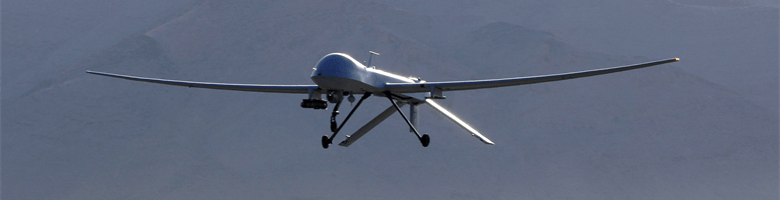
Is using Artificial Intelligence in military applications evil? Are programs like Project Maven necessary to decrease civilian casualties and reduce error in an era with "smart" drones and other advanced weaponry?
In May of 2018, controversy erupted on the Google campus with a signed petition by over 4000 employees not to continue its contract with the US Department of Defense (DOD) on Project Maven, a cooperative project between Google and the DOD intended to apply machine learning to analyze drone footage. Employees cited Google’s “Don’t be Evil” policy in their memorandum to Google executives.[1] Yet the role of Artificial Intelligence (AI) in Project Maven was to differentiate legitimate targets from possible collateral damage. As a former US Army Officer responsible for selecting targets from drone footage, I experienced the enormous problem of separating legitimate targets from non-combatants which Project Maven is supposed to solve.
We often hear about “drone warfare” in the news media but how does the process actually work? Current legacy drones are not smart. Drones such as the Air Force’s MQ-1 Predator go up in the air and provide video feed to a command center with no analytical processes involved. It is up to the intelligence assets inside the command center to “identify friendly or foe before approval to fire is given.”[2] Having served as both an intelligence officer and a targeting officer, I can attest that these intelligence assets are just people with the proper training. However, training does not mitigate the difficulty of determining whether a grainy video from 5 kilometers away is a goatherder with a staff, or a militant with a rifle. Since this process is so imperfect, the military has many layers of bureaucratic control to prevent attacking the wrong target. This process, referred to as the Target Selection Process can often take from 24-36 hours.[3] Even with the layers of control, accidents still happen such as the airstrike in Syria that mistakenly killed 18 allied fighters rather than its intended ISIS targets.[4]

This is a problem that machine learning can fix. Project Maven is the “DoD’s integration of AI, big data, and machine learning across operations to maintain advantages over increasingly capable adversaries.”[5] Adaptive programs can recognize patterns based on previous data, images, and behavioral characteristics that people cannot. The first step was to differentiate between different objects on the battlefield such as vehicles, personnel, and weapons. According to Google engineer Reza Ghanadan, “the results showed several cases that with 90+% confidence the model detected vehicles which were missed by expert labelers.”[6] If this machine learning continues to be successful, it has the potential to decrease collateral damage and drastically increase lethality by shortening processing time in the targeting process.
The military’s main goal in the next few years will be to further capabilities such as those in Maven, with the intention to better differentiate friend from foe and also to use AI to identify targets from other intelligence sources. Over a longer horizon of the next 10 years or so, the military is using machine learning is to shorten the Targeting Selection Process as a whole. As previously mentioned, once targets are identified, a lengthy bureaucratic process begins that compares the possibility of collateral damage, civil and legal considerations, and probability for success. A massively time-consuming process, the Target Selection Process, is “limited by the number of targeteers that Western armed forces, particularly NATO, have now, and it is almost impossible to perform.”[7] For now, the military is relying on individuals but AI is well-suited to solve this problem.

While the military is actively pursuing machine learning applications in the Targeting Process, funding levels have not reflected this priority. R&D spending in the Defense industry is only 15% of the Information and Communication space.[8] A key problem in the industry is a limited number of qualified engineers that have strong hardware and software expertise. There is fierce competition for these engineers from Silicon Valley startups and therefore the “aerospace and defence sector, where funding lags behind, is less appealing to the most able personnel.”[9] One way to signal real change is to put more dollars towards R&D in this sector. Additionally, the DOD should consider education funding for promising engineering students in return for a work commitment on DOD related projects.
To conclude, machine learning has many applications in the military context but AI in the targeting sphere is not evil and represents real opportunities to increase effectiveness of current assets while decreasing collateral damage. In this article, all applications of AI implied human control on weapons release criteria. Another point of consideration are the ramifications of allowing AI weapons release criteria, i.e. take human life. (745)
[1] Khari Johnson, “AI Weekly: Google should listen to its employees and stay out of the business of war,” Venture Beat, April 6, 2018, https://venturebeat.com/2018/04/06/ai-weekly-google-should-listen-to-its-employees-and-stay-out-of-the-business-of-war/, accessed November 2018.
[2] US Army Field Artillery School, “Army Technical Publication 3-60: Targeting” (May 2015), p. 2-5.
[3] Ibid, p. 4-6.
[4] Eric Schmidt and Anjali Singhvi, “Why American Airstrikes Go Wrong,” The New York Times, April 14, 2017, https://www.nytimes.com/interactive/2017/04/14/world/middleeast/why-american-airstrikes-go-wrong.html, accessed November 2018.
[5] Merel Ekelhof, “LIFTING THE FOG OF TARGETING: ‘Autonomous Weapons’ and Human Control through the lens of Military Targeting,” Naval War College Review, (July 2018), https://global-factiva-com.prd2.ezproxy-prod.hbs.edu/ha/default.aspx#./!?&_suid=1542042310566043656795788349134, accessed November 2018.
[6] Kate Conger, “Google Plans not to Renew Its Contract for Project Maven,” Gizmodo, June 1, 2018, https://gizmodo.com/google-plans-not-to-renew-its-contract-for-project-mave-1826488620, accessed November 2018.
[7] Merel Ekelhof, “LIFTING THE FOG OF TARGETING: ‘Autonomous Weapons’ and Human Control through the lens of Military Targeting,” Naval War College Review, (July 2018), https://global-factiva-com.prd2.ezproxy-prod.hbs.edu/ha/default.aspx#./!?&_suid=1542042310566043656795788349134, accessed November 2018.
[8] M.L Cummings, “Artificial Intelligence and the Future of Warfare,” Chatham House, https://www.chathamhouse.org/sites/default/files/publications/research/2017-01-26-artificial-intelligence-future-warfare-cummings-final.pdf, accessed November 2018.
[9] Ibid.
The 90% statistic makes it clear how advantageous it is to use AI to inform and expedite target identification. One question that arises for me (outside of the internal talent and funding issues) is the vulnerability of AI and electronic messaging to hacking by opposing forces, who could alter the output and/or inner workings of the tool. It is therefore still critical to involve the human element and continue to train on target identification. However, in the future, I imagine training will also have to include how to weigh AI data with the human element and make those critical decisions.
Great writeup! You mention that lack of funding is a primary reason for the lack of talent acquisition in this field. I was reading an article which spoke about the current political uncertainty and how it impacts this. Precisely, I would quote from the article ” The current uncertainties are influencing the initial and midcareer employment decisions of R&D professionals who typically would have been interested in defense-sector careers but, today, have diverse options in other areas” https://physicstoday.scitation.org/doi/10.1063/1.1372115
Since you are from the same background, I would be interested in knowing your opinion.
Very fascinating article. One interesting thought that occurred to me while I was reading this was who should get the blame when the AI makes a “mistake” and the human acts based on that mistake? It might be easy to say that the human should, because they’re the one that made the ultimate decision, but if there is a gradual transition from human control to AI control, at what point would the blame shift? Is it always going to be “who” presses the final button? And if it is the AIs “fault”, are the developers the ones to blame? Or simply the AI itself? It seems like a hard question to answer, but a seemingly important one when the stakes seem so high.