Predix at GE: Machine Learning in Industrial IoT
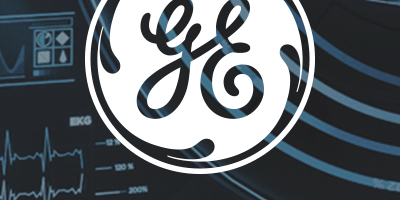
Predix is GE's attempt to harvest data from the industrial 'internet of things' and employ machine learning algorithms to optimize industrial systems.
Overview
For over 120 years, General Electric has specialized in the manufacture of heavy industrial equipment such as power turbines, jet engines, and medical imaging devices. Consequently, when Jeffrey Immelt, former chief executive of GE, centralized digital components of the company’s business lines into a new division – contending that software would be GE’s driver of growth in the future – it represented a departure from the organization’s core business and a modernization of the firm. [1]
At the center of this strategy was an application called Predix: a union of GE’s software products intended to serve as an operating system for heavy industry. The idea was to collect information from the industrial ‘internet of things’ and employ machine learning to optimize maintenance and usage of industrial systems.
GE had several applications used to monitor physical assets. For example, sensors on turbines might communicate information on wind speed, power output, and motor diagnostic conditions. By centralizing information and building virtual models (‘digital twins’) of equipment, GE could employ algorithms to predict breakdowns, optimize maintenance tasks for specific components, or rebalance an entire system and reduce costs. Predix represented tremendous value for the firm internally, as it would allow GE to improve internal asset usage, and externally, as they intended to sell Predix as a data-centric platform for process improvement to industrial customers. [2]
The Value of Predix [3]
Growth Challenges
At launch, Immelt envisioned Predix as a foundational pillar for GE’s growth, contending that the system would push GE to become a leading software company by 2020. [4] Predix would enable even less tech-savvy customers to model physical systems with the ‘software equivalent of Lego blocks’ in an ecosystem backed by GE’s machine learning algorithms for optimization. Spending matched this vision as the company hired over 1500 engineers and spent ~$4B on development in 2016. [5] However, GE Digital was hindered by P&L responsibility as development of Predix represented a huge expense. To achieve profitability, the digital arm of the company began acting as a consulting shop to industrial companies, building specialized programs for factory operations, equipment controls and monitoring that represented short-term digital projects as opposed to new, flexible platforms for modeling and machine learning that would provide long-term value to GE and its clients. [6]
In 2017, John Flannery, the newly minted chief executive, changed the company’s strategy for Predix, slashing the budget for the product and insisting on a more ‘focused approach’, before engaging an investment bank to seek buyers for GE’s digital business. [7] The latest GE CEO, Larry Culp, has continued to discuss asset sales as a method for raising capital, and the future of Predix remains uncertain. The company may divest in its digital arm and move away from industrial applications of machine learning as a primary growth driver. [8]
Future direction
In asset intensive industries, there is a clear value proposition for machine learning. Transportation or energy systems can be optimized to improve utilization and load balancing. Models that pinpoint preventative maintenance of even the smallest component in a single piece of equipment hold the potential to save millions as component failures might otherwise result in system outages. Consequently, it would be a mistake for GE to abandon Predix entirely, but the product does need focus. In the short-term, Predix should be refined for internal use to improve performance of the company’s physical systems as there is some indication that GE divisions (Aviation) struggled with effectively employing Predix. [9] Investment in R&D should ensure that Predix can model ‘digital twins’ across all of GE’s own asset classes. GE should also continue to form partnerships with customers in industry to expand its platform and model systems outside of its core business lines. Working with clients to build specialized analytics applications is an excellent interim strategy if GE can utilize those projects to generalize and improve its existing models. The long-term goal for the company should be to fashion Predix into a truly flexible AI engine that can be fit to any industrial system for optimization.
There are several competitors and eager entrants in industrial IoT. Traditional competitors like Rockwell Automation, tech giants such as Google and Amazon, and start-ups like C3 IoT pose a threat to GE’s Digital business. In some ways, competitors are better positioned both to handle massive amounts of data from industrial systems and to develop generalizable algorithms to improve operations in industry. Moreover, software is at least one step removed from GE’s core competency of product development and manufacture of physical equipment. This set of circumstances raises a few questions. Should GE divest in Predix and concede to competitors in this space? Would the company be better served by focusing on core competencies or does machine learning in industrial IoT truly represent a brighter future for GE?
(797 words)
References
[1] “Creation Of GE Digital”. Businesswire.Com. September 14, 2015. https://www.businesswire.com/news/home/20150914006029/en/Creation-GE-Digital, accessed November 2018.
[2] Lohr, Steve. “G.E., The 124-Year-Old Software Start-Up”. Nytimes.Com. August 28, 2016. https://www.nytimes.com/2016/08/28/technology/ge-the-124-year-old-software-start-up.html?_r=0, accessed November 2018.
[3] “Predix: The Industrial IoT Application Platform.” GE Digital Platform Brief. 2018. https://www.ge.com/digital/iiot-platform , accessed November 2018.
[4] Lohr, Steve. “G.E., The 124-Year-Old Software Start-Up”. Nytimes.Com. August 28, 2016. https://www.nytimes.com/2016/08/28/technology/ge-the-124-year-old-software-start-up.html?_r=0, accessed November 2018.
[5] Lohr, Steve. “G.E. Makes a Sharp ‘Pivot’ on Digital”. Nytimes.com. April 19, 2018. https://www.nytimes.com/2018/04/19/business/ge-digital-ambitions.html, accessed November 2018.
[6] Moazed, Alex. “Why GE Digitial Failed”. Inc.com. January 8, 2018. https://www.inc.com/alex-moazed/why-ge-digital-didnt-make-it-big.html, accessed November 2018.
[7] Cimilluca, Dana; Mattioli, Dana and Gryta, Thomas. The Wall Street Journal. July 30, 2018. https://www.wsj.com/articles/ge-puts-digital-assets-on-the-block-1532972822, accessed November 2018.
[8] Sheetz, Michael. “GE drops below $8 after CEO Culp says he feels the ‘urgency’ and will sell assets to raise cash”. CNBC.com. November 12, 2018. https://www.cnbc.com/2018/11/12/ge-ceo-culp-says-he-will-use-asset-sales-to-raise-cash-and-bring-leverage-down-feels-the-urgency.html, accessed November 2018.
[9] Immelt, Jeffrey. “How I remade GE”. Harvard Business Review. September 2017. https://hbr.org/2017/09/inside-ges-transformation, accessed November 2018.
I do not think that GE should continue investing in Predix for a couple of reasons. GE is facing competition from much more nimble start up companies like C3 IoT and Palantir that have a core competency in software development. These companies are able to develop software at a quicker pace and gain a competitive advantage. Additionally, because the nature of the space, it will be very difficult to create a “one size fits all” solution. Even within one industry, there are serious challenges when looking at data availability, uniformity, and sources. These will vary by customer and therefore require individualized solutions.
IoT seems like such a promising new horizon but has been slow on the uptake. I think the current challenge in IoT is that much of the technology’s potential exists in untapped opportunities for optimization*between* companies in an ecosystem, rather than the low-hanging fruit of optimization within a single company’s operations. It will take a shift in business mindset to apply IoT solutions to larger ecosystem problems. If GE does choose to stick with Predix, they would do well to guide their clients toward broader applications of IoT to expand their market.