Predictive Policing: Promoting Peace or Perpetuating Prejudice?
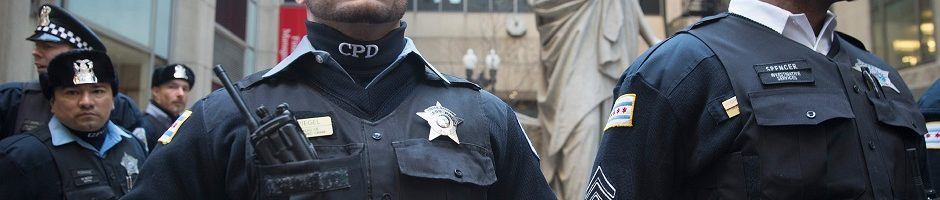
The Chicago Police Department's embrace of machine learning raises critical questions about the balance between community safety, civil liberties, and systemic bias.
What if you could stop a crime before it happened? That’s the promise of “predictive policing,” which uses algorithms to analyze data about past criminal activity in order to illuminate patterns and establish hypotheses about future wrongdoing. This approach allows law enforcement to be proactive rather than reactive, ideally lowering crime rates and enhancing community safety.[1]
The city of Chicago has the highest number of homicides per year across all U.S. cities.[2] This epidemic of violence presents a challenge to the Chicago Police Department (CPD), which must efficiently deploy human and financial resources to keep residents safe. By incorporating machine learning into its policing process, CPD can focus its efforts on communities and individuals that are supposedly most likely to be involved in criminal activity.
In 2013, CPD partnered with researchers at the Illinois Institute of Technology to create an algorithm that identifies Chicagoans most at risk of being victims or perpetrators of violent crimes.[3] This “Strategic Subject List” (SSL) assigns a score of 1 to 500 based on an individual’s probability of being involved with a shooting or murder. The score is generated based on factors including a person’s history of arrests and connections to past criminal activity.[4]
The SSL is used to facilitate proactive interventions through CPD’s “Custom Notification Program.” Police officers and social workers visit the homes of people with high risk scores to warn them that they will likely be involved in a shooting as the victim or perpetrator, and to offer resources such as drug treatment programs, housing and job training, and support for leaving gangs.[5] Police personnel also inform them that the highest possible criminal charges will be pursued if they are found to be perpetrators of any further criminal activity.[6]
In the coming years, CPD will need to demonstrate that the SSL program is effective. A 2016 study by the RAND corporation found that people on the so-called “heat list” were not more or less likely to be involved in a shooting or homicide than the control group.[7] CPD must also figure out how to maximize the utility of the SSL algorithm. According to a RAND researcher, officers are given vague directives on how to use the SSL, and thus the predictive data is not leveraged in an impactful and actionable way.[8]
CPD intends to increase its reliance on machine learning in the next decade. In October 2018, the mayor announced an expansion of the city’s “smart policing strategy,” building on a pilot program that uses technology to complement existing policing practices. [9] For example, CPD will use past crime data (and other inputs including phases of the moon and schedules of sports games)[10] to predict where criminal activity might occur, and provide officers with real-time analysis on mobile apps to inform their patrols. Initial data suggests that these technological tools have contributed to a citywide decline in violence over the past year. [11]
As CPD continues to incorporate machine learning tools such as SSL into its policing process, the algorithms must be more transparent. Predictive policing programs can undermine a person’s right to the presumption of innocence and other civil liberties.[12] The use of opaque algorithms might also exacerbate the tense relationship between community members and law enforcement. In a statement on the use of predictive policing by CPD, a representative from the ACLU of Illinois wrote, “We are at a crisis point in Chicago regarding community and police relations. Transparency is critical to restore faith in the system.”[13]
CPD must also ensure that the SSL system does not reinforce biases against communities of color. According to Chicago Magazine’s analysis, 56% of Black men ages 20-29 in Chicago have an SSL score.[14] This is potentially the result of a negative “feedback loop” given that the algorithm considers a person’s past arrests. Studies have shown that Black people are arrested for drug possession, for example, at disproportionate rates,[15] so any program that uses historical arrests to predict future criminal activity relies on biased inputs.
Finally, two questions warrant consideration. First, should police departments employ predictive policing technologies? Such algorithms could theoretically eliminate the need to rely on unjust proxies such as race to drive policing practices. However, predictive policing also has the potential to undermine civil liberties, perpetuate structural bias, and lead to unnecessary surveillance.
The second question invites imagination — are there other opportunities for police departments to leverage machine learning to increase community safety? For example, a team of data scientists at the University of Chicago created an algorithm to identify police officers with a propensity for excessive force.[16] Or perhaps CPD could wield its predictive technology to take a holistic, preventative approach to community safety, proactively connecting service providers with individuals most impacted by the root causes of violence, such as poverty and mental health issues.[17]
[795 words]
[1] Leslie A. Gordon, “Predictive policing may help bag burglars—but it may also be a constitutional problem,” ABA Journal 99(9) (September 2013): 1.
[2] Cheryl Corley, “Chicago Battles Its Image As Murder Capital Of The Nation,”NPR, August 10, 2018, https://www.npr.org/2018/08/10/637410426/chicago-battles-its-image-as-murder-capital-of-the-nation, accessed November 12.
[3] Jeff Asher and Rob Arthur, “Inside the Algorithm That Tries to Predict Gun Violence in Chicago,” The New York Times, June 13, 2017, https://www.nytimes.com/2017/06/13/upshot/what-an-algorithm-reveals-about-life-on-chicagos-high-risk-list.html, accessed November 12.
[4] Chicago Police Department, “Special Order S09-11: Strategic Subject List (SSL) Dashboard,” July 14, 2016, http://directives.chicagopolice.org/directives/data/a7a57b85-155e9f4b-50c15-5e9f-7742e3ac8b0ab2d3.html, accessed November 12.
[5] Monica Davey, “Chicago Police Try to Predict Who May Shoot or Be Shot,” The New York Times, May 23, 2016, https://www.nytimes.com/2016/05/24/us/armed-with-data-chicago-police-try-to-predict-who-may-shoot-or-be-shot.html, accessed November 12.
[6] Chicago Police Department, “Special Order S09-11: Strategic Subject List (SSL) Dashboard,” July 14, 2016, http://directives.chicagopolice.org/directives/data/a7a57b85-155e9f4b-50c15-5e9f-7742e3ac8b0ab2d3.html, accessed November 12.
[7] Jessica Saunders, Priscillia Hunt, and John S. Hollywood, “Predictions put into practice: a quasi-experimental evaluation of Chicago’s predictive policing pilot,” Journal of Experimental Criminology 12(3) (September 2016): 347-371.
[8] John S. Hollywood, “CPD’s ‘heat list’ and the dilemma of predictive policing,” Crain’s Chicago Business, September 19, 2016, https://www.chicagobusiness.com/article/20160919/OPINION/160919856/chicago-police-s-heat-list-and-what-to-do-with-predictive-policing, accessed November 12.
[9] “Mayor Emanuel Announces Expansion Of Smart Policing Strategy Supporting Nearly Two Years Of Consecutive Declines In Crime,”
press release, October 10, 2018, on City of Chicago Office of the Mayor website, https://www.cityofchicago.org/city/en/depts/mayor/press_room/press_releases/2018/october/101018_ExpansionSmartPolicingStrategy.html, accessed November 12.
[10] “Violent crime is down in Chicago,” The Economist, May 5, 2018, https://www.economist.com/united-states/2018/05/05/violent-crime-is-down-in-chicago, accessed November 12.
[11] “Mayor Emanuel Announces Expansion Of Smart Policing Strategy Supporting Nearly Two Years Of Consecutive Declines In Crime,”
press release, October 10, 2018, on City of Chicago Office of the Mayor website, https://www.cityofchicago.org/city/en/depts/mayor/press_room/press_releases/2018/october/101018_ExpansionSmartPolicingStrategy.html, accessed November 12.
[12] ACLU, “Statement of Concern About Predictive Policing by ACLU and 16 Civil Rights Privacy, Racial Justice, and Technology Organizations,” August 31, 2016, https://www.aclu.org/other/statement-concern-about-predictive-policing-aclu-and-16-civil-rights-privacy-racial-justice, accessed November 12.
[13] Karen Sheley, “Statement on Predictive Policing in Chicago,” June 7, 2016, https://www.aclu-il.org/en/press-releases/statement-predictive-policing-chicago, accessed November 12.
[14] Yana Kunichoff and Patrick Sier, “The Contradictions of Chicago Police’s Secretive List,” Chicago Magazine, August 21, 2017, https://www.chicagomag.com/city-life/August-2017/Chicago-Police-Strategic-Subject-List/, accessed November 12.
[15] “Violent crime is down in Chicago,” The Economist, May 5, 2018, https://www.economist.com/united-states/2018/05/05/violent-crime-is-down-in-chicago, accessed November 12.
[16] Rob Mitchum, “Using data science to confront policing challenges,” UChicago News, August 25, 2016, https://news.uchicago.edu/story/using-data-science-confront-policing-challenges, accessed November 12.
[17] Andrew V. Papachristos, “CPD’s crucial choice: Treat its list as offenders or as potential victims?” Chicago Tribune, July 29, 2016, https://www.chicagotribune.com/news/opinion/commentary/ct-gun-violence-list-chicago-police-murder-perspec-0801-jm-20160729-story.html, accessed November 12.
Thank you for this very important essay. I think you are absolutely correct in highlighting the many potential pitfalls with machine learning. Unfortunately, these issues are not getting enough air time and could lead to catastrophic results. I fundamentally challenge the notion that machine learning can be unbiased in its prediction of crime. As you point out, it relies on too much biased data and has an unchecked feedback loop. Because of these issues, I wholeheartedly agree that it would be more powerful to repurpose ML to help prevent the use of excessive force or to connect people with needed resources. There is very little downside in these cases, whereas the downside in the current applications is enormous and has a generational impact. I also wonder if there needs to be government intervention. Perhaps laws need to be created to prevent certain machine learning/artificial intelligence applications because of the implications and severity of biased data.
Thanks for this article. This strikes at the heart of something that’s been concerning me with machine learning for quite some time which has to do with the objectiveness and quality of the inputs to the algorithm. I don’t see any way that historical inputs do not perpetuate structural and societal biases or even more so confirm biases in people when they see a high SSL score. I think there is an inherent danger in the unchecked feedback loop within the ML system as well as an unchecked feedback loop from the SSL score to the individual interpreting the score. I think this could be a good tool in the toolkit if it yields responses of increased support and resources to mitigate potential crimes rather than reinforce societal expectations. I would focus on training the user of the data to understand what the output means and what actions they should take based on that output, which I fear will not happen considering significant resource constraints as well as a low prioritization.
Thank you, Holly for this article! I completely agree with the risk of using machine learning to assess resident risk profiles, as emphasized in the previous two comments. However, I also would like to bring attention to two other threats that Holly mentioned: 1) that there is limited faith in the integrity of the system and 2) that members of the “heat list” are no more or less likely to be involved in a crime. These threats indicate to me that the CPD has a more pressing issue than implementing machine learning to predict criminal propensity: they have a trust problem.
Without trust, no level of machine learning insights will suffice to enable CPD to operationally and proactively reduce crime outcomes. It would therefore make sense to me for the CPD to run a resident (not academic) competition on trust. Conduct deep interviews of South- and West-side, crime-prone neighborhoods. Get to know why residents mistrust the police and figure out how to rectify that relationship. Once residents view the police as an ally, rather than a threat, then the police and residents will be able to proactively partner together towards a safer community. Maybe then, the #1 source of information will come directly from responsible neighbors, rather than an expensive machine learning algorithm with information asymmetry challenges.
Holly, thank you very much for such an interesting essay! In my opinion, the algorithm intends to solve very important problems in society and is likely to have a great future ahead. Nevertheless, like you, I struggle to understand the ethical implications of the project. Past crimes, race or place of living is not a 100% predictor of future crimes and I think approaching people at “high risk” category may be unfair and frustrating. People are labeled because of certain criteria that algorithm decides important, which have nothing to do with an individual’s intention of committing a crime in the future. As you noted in the essay, it is a direct violation of liberty and presumption of innocence. In order to make algorithm less inclined to “label” innocent people, I would suggest developers alter the algorithm in the way it focuses only on people who are at risk of being victims, not on potential perpetrators. Although it may limit the effectiveness of the program, it will not have undesired side effects that may hinder liberty.
Holly, thanks for the great article. I agree with you and other commenters that it is very hard to trust machine learning using inputs that have been proven to be biased in the past.
I also wanted to touch on the point of transparency which I think hits on this tension the world (especially in the USA and since 9/11) faces between privacy and (national) security. If, and this is a big if, this could remove bias and actually be predictive, would there still be a concern using machine learning? Transparency could allow criminals or others to game the system and thus defeat the purpose but I agree the trust needs to be there first anyways. This is more of a philosophical or thought exercise as I believe we are a long way from removing bias from the system and actually being able to predict crimes using statistics or algorithms but believe it needs to be considered. I unfortunately feel we are moving this way even before we have proven results or understand the ethics and tradeoffs but do think the considerations should continue to be raised!
This technology poses a huge risk for racial profiling and can exacerbate mistrust in communities. It could have profoundly damaging psychological effects and possibly physical safety risks for those who are inappropriately targeted based on their characteristics. If we want to be proactive about ending crime, we need to invest in schools, rehabilitation programs, and social services. To me, the problem is less about identifying individuals struggling with mental illness, drug addiction, and poverty, and way more about the lack of resources available to help these individuals. If you enter any hospital or emergency department for example, you’ll be able to identify individuals struggling with these issues, and you’ll also find providers and social workers with minimal resources with which to create lasting change.
I would suggest that those implementing predictive policing technologies think critically about the type and magnitude of crime they are trying to prevent using this technology. How they implement this technology to identify human trafficking and search for trafficking victims, for example, may be very different from how they would deploy the technology in an attempt to prevent other crimes.
For those interested in learning more about building trust between police and communities, My90 is a start-up that amplifies voices in the community by allowing people to anonymously share feedback about their experience with law enforcement: http://www.textmy90.com/
Thanks for the interesting article! I agree with you and above comments regarding the risks of using historical data for predictive purposes, especially in analyzing criminal records. It might be helpful to understand the common traits among criminals using data analytics, and that can help to define preventive actions; however in my opinion labeling people even before they commit a crime as potentially guilty is not going to work. This is a very sensitive topic, on which even human thinking is sometimes biased.
Nevertheless, I believe security enforcement can benefit from other areas of technological developments and AI. For example, there are many companies emerging that use machine learning to analyze visuals from surveillance cameras to detect “suspicious activity”. Using machine learning will then much less resources to monitor and analyze real-time videos and interfere if needed. Data privacy concerns will be still there, but I believe this will improve day-to-day security operations in a positive way if used well.