Predictive Maintenance at NRG
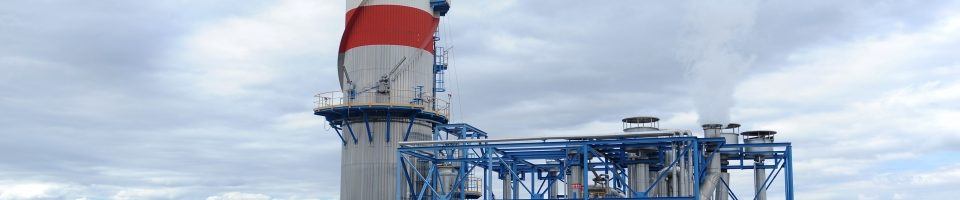
How will predictive maintenance impact NRG's competitive positioning?
NRG operates over 26,000 megawatts (MW) of electricity generation capacity as well as transmission and distribution infrastructure serving over 3 million end customers.[1] Machine learning, particularly through predictive maintenance and the Internet of Things (IoT), is poised to fundamentally transform the way that NRG operates power plants.
Old Model: Scheduled Maintenance
The old model of power plant maintenance used maintenance schedules informed by the running hours of a power plant. For example, General Electric’s 6B.03 43 MW capacity gas turbine must be stopped either every 24,000 hours of operation or after 1,200 starts for a hot gas path inspection.[2] These maintenance schedules are determined by the original equipment manufacturer (OEM), in this case, GE. When a turbine reaches the threshold, the operations team performs the scheduled maintenance. The risk of scheduled maintenance is that the actual performance of the turbines may not match the performance of the average turbine that is used to calculate scheduled maintenance guidance from the OEM. A turbine may run under worse atmospheric conditions than expected or may have a different frequency of cold, warm, and hot starts. Following scheduled maintenance guidelines results in performing maintenance too early (leading to unnecessary outages and cost) or too late (potentially resulting in higher maintenance costs or forced outages).
New Model: Predictive Maintenance
Power plants have always collected an enormous quantity of data. Even plants operating with legacy analog control rooms record power plant operations manually by hand many times per hour. Now with computer technology the data generated by power plants is accessible to support improved operations decisions. Further, IoT devices allow new data to be collected in a more granular fashion or in more remote locations.[3] With machine learning, this data can be used to improve the maintenance cycles from scheduled maintenance to predictive maintenance.[4] Data analysis can identify under what patterns of operating conditions equipment is most likely to break down.
Impact of Predictive Maintenance
In competitive markets, thermal power plants typically make money based on the spark spread. The spark spread is the price per megawatt-hour (MWh) of electricity sold in the spot market less the cost of fuel per MWh and less the cost of variable operations costs per MWh. Predictive maintenance can help generators reduce their operations and maintenance costs and increase their spark spread margin.[5][6] Plants with a higher spark spread will have a more competitive position in the merit order dispatch curve, allowing them to increase their dispatch frequency and better cover their fixed costs. Finally, forced (unplanned) outages leave generators exposed to purchasing replacement energy in the spot market to satisfy contractual obligations.
NRG Implementation of Predictive Maintenance Technology
NRG spent approximately $1.4bn on operations and maintenance expenses in 2017.[7] It’s reported results noted significant variations of this cost category due to the timing and occurrence of planned and forced outages. NRG signed a predictive maintenance agreement with Azima DLI covering its Ivanpah concentrated solar thermal plant in California.[8] Azima’s technology uses vibration analysis and oil testing to detect potential problems before they arise. In the longer term, the industry is moving towards using predictive maintenance in place of preventative maintenance and NRG is on the leading edge of this trend.
Strategic Positioning for NRG
NRG should continue to implement predictive maintenance across their fleet of generation assets to improve performance and competitive positioning. A broader strategic question for NRG is how they should roll-out this technology – through external partnerships with specialized providers or through exclusive partnership with providers and internal efforts. If all generators lower their variable costs through predictive maintenance, then in a competitive market they will bid down the spark spread back to the original margin. That is, the value created will not be captured by the generators but will be passed to the end user.
Machine learning and predictive maintenance technologies improve as they process more data. The second and third-movers get incrementally more accurate results than the first-mover. If NRG implements predictive maintenance with external providers it may lose the exclusivity of data created by its 26,000 MW of generation capacity. As one of the largest US independent power producers NRG could benefit from exclusive partnerships or even acquiring a technology provider and internalizing the technology. Such a strategy could allow NRG to maintain a competitive advantage on variable costs and key operating metrics.
How can NRG best implement advances in machine learning technology for predictive maintenance? Can NRG capture its share of the value created?
(741 words)
[1] NRG, 2017 Annual Report.
[2] Heavy-Duty Gas Turbine Operating and Maintenance Conditions, Justin Eggart, Christopher E. Thompson, Jerry Sasser, and Mardy Merine. (Atlanta: GE Power, October 2017), https://www.ge.com/content/dam/gepower-pgdp/global/en_US/documents/technical/ger/ger-3620n-heavy-duty-gas-turbine-operationg-maintenance-considerations.pdf
[3] IIoT Predictive Maintenance—Redesigning the Maintenance Repair and Overhaul (MRO) Approach, 2018, Frost & Sullivan, accessed November 2018.
[4] Erik Brynjolfsson and Andrew McAfee, “What’s Driving The Machine Learning Explosion?” Harvard Business Review, July 2017: 12-13.
[5] “Moving from Preventive to Predictive Maintenance with Advanced Analytics,” Power, https://www.powermag.com/moving-from-preventive-to-predictive-maintenance-with-advanced-analytics/, accessed November 2018.
[6] “Facilitating Predictive Maintenance of Electrical Assets with Pervasive Sensing Strategies,” Power, https://www.powermag.com/facilitating-predictive-maintenance-of-electrical-assets-with-pervasive-sensing-strategies/, accessed November 2018.
[7] NRG, 2017 Annual Report.
[8] “Azima DLI, NRG Energy Announce Predictive Maintenance Agreement,” Azima DLI Press Release, https://www.reliableplant.com/Read/29311/azima-nrg-agreement, accessed November 2018.
CBH, thank you for sharing this perspective on NRG’s predictive maintence implementation. As you pointed out, the value that machine learning can bring, is tremendously increased when the model is fed a large volume of data to train the system. The OEM’s like GE have suggested maintenance schedules, and in some cases these maintenance guidelines must be followed to be compliant with regulatory guidelines. Thus, there are probably situations in which the predictive maintenance model would propose waiting longer for a maintenance activity than is advised by the OEM. Additionally, I imagine nuclear plants have more stringent guidelines than those of a combined cycle plant, because the implications of a failure are more significant. As such, I’m curious about how OEMs and regulatory agencies are approaching this trend. Operators across the industry are certainly looking for ways to minimize cost, though I’m sure the OEMs and agencies may push back on it.
Thanks for the article! The trade-off between the need to have more data to improve machine learning as quick as possible, and the loss of the competitive edge is super interesting. However, I think there could be a scenario where the competitive advantage is in the development of the machine learning algorithm to predict maintenance, and not necessarily in the data itself. Alternatively, one could think about setting up a subsidiary company that is in change of developing these algorithms and collecting the data through partnerships. By doing so, this “subsidiary” could offer the power plant operators an outsourced service so that so that the operators can keep doing what they do best and not worry about developing new internal capabilities!
This is a fascinating article. I find it particularly interesting in this sector where maintenance equates to large scale efficiencies in the system. I’d be curious to see how this could be implemented in a user friendly interface. In so many cases, humans need to have the ability to dig deeper into the data in order to understand the proper remedial action. I’d be fascinated to see how power plant operators could implement this technology at a variety of scales!