Pinterest Needs to Improve Revenue Per User Ahead of IPO
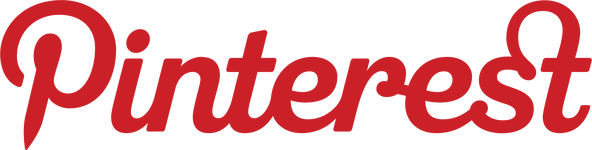
Pinterest's userbase is growing faster than its publicly-traded peers, but the company lags in revenue per user. I exam their existing machine learning infrastructure and recommend how to use it improve revenue growth ahead of their 2019 IPO.
Machine learning is imperative to Pinterest’s business model and is an important area of focus as the company prepares to go public next year at a valuation of up to $15 billion1. This valuation reflects strong user growth of almost 15 percent last year, which is significantly higher than that of Twitter and Facebook who each had 9% over the same period2,3. While the Pinterest’s user growth is impressive, their revenue per user lags that of their peers at just $5, compared to $8 for Twitter and $25 for Facebook4,5,6.
It is imperative for Pinterest to grow revenue per user given their relatively narrow target user base of predominately women aged 25 – 54, 60% of whom are mothers7. Further, revenue per user growth is one of the main valuation and performance metrics used by technology investors8, making it even more important as Pinterest looks to attract public shareholders. In this essay, I will propose new ways to use Pinterest’s existing machine learning infrastructure to increase revenue per user.
How Pinterest Works
Pinterest has a vast userbase of 200+ million unique users9 and processes 150 million image searches per month10. Pinterest users curate “pins” by selecting images from across the web and saving them into different collections, called “boards”. These pins maintain their original website links when they are “repined” by different users, effectively allowing users to share images and idea sources with one another11. Pinterest earns revenue from retailers who pay to have their pins prioritized on a user’s stream through “promoted pins”12.
Exhibit 1: How A Link is Saved Through Pinterest13
How Pinterest Uses Machine Learning
Pinterest uses GPUs (Graphic Processing Units) to analyze and draw inferences from information within pinned images, including image characteristics (color, quality, etc.) as well as metrics about the source website and the profiles of the Pinterest users interacting with the pin. These relationships are used to segment users by interests, frequency of actions and sequence of actions, and ultimately to predict user behavior. Given the amount of data that Pinterest has, they can now predict if a user can will interact with a new pin with 77% – 85% accuracy14, giving them a differentiated advantage in recommending new content for their userbase.
Currently, Pinterest excels at recommending pins similar to ones previously saved by each user but hopes to improve the ability to identify complementary information related to user searches15. Recently, Pinterest developed Pinterest Lens, which allows users to take a photo with their smartphone and then uses machine learning to attempt to source related items16. This is how Pinterest uses machine learning to differentiate themselves and improve their product: by learning about a user’s interests and then predicting helpful and relevant information before the user even knows what they are looking for.
How Pinterest Can Use Machine Learning to Improve Revenue Per User
As described above, Pinterest uses machine learning to both segment users into target groups and predict their behavior with a high level of accuracy. However, the company is not using this information to optimize their clients’ promoted pins and resulting revenue. Currently, the majority of Pinterest purchases are originally discovered through the boards of strangers or friends, versus just 7% sourced through retailer’s promoted pins. Further, only 19% of Pinterest-driven purchases involved a sale or promotion sourced through Pinterest, compared to 37% of Facebook and 32% of Twitter-driven purchases17.
These shortcomings highlight a significant revenue growth opportunity if Pinterest can use their existing machine learning infrastructure to better match promoted pins to target markets. Specifically, I believe that Pinterest should segment users into two main categories: frugal, price-conscious purchasers and trend-conscious buyers who are willing to pay full price. Below is a table describing these two representative buyers, with suggested promoted pin strategies for each:
The key to this strategy in predicting the buying behavior of each user, profiling them based on how they make purchases, rather than what they purchase. This allows retailers to get the most revenue from each segment. They will close more deals with price-conscious buyers by alerting them to sales, without unnecessarily giving up revenue by showing discounts to consumers who are willing to buy premium or newer items at higher prices. If Pinterest can successfully segment and target these two groups, they will achieve stronger revenue per user growth to amplify their already impressive userbase statistics and business model.
Key Questions:
How specific should Pinterest be with segmenting? Is there a threat to retailers by offering a price comparison tool within the app as suggested above?
Word Count: 711
References
- Castillo, M. (2018). Pinterest nears $1 billion in ad sales and valuation rises as it looks to go public in mid-2019. CNBC. Retrieved from http://www.cnbc.com.
- Twitter, Inc. (NYSE: TWTR) (2018). Twitter Third Quarter 2018 Results. Twitter, Inc. Retrieved from EDGAR.com
- Facebook, Inc. (NASDAQ: FB) (2018). Facebook Third Quarter 2018 Results. Facebook, Inc. Retrieved from EDGAR.com
- Laughlin, L. (2018). Breakingviews – Pinterest shapes up for anti-millennial IPO. Reuters. August 8, 2018
- Twitter, Inc. (NYSE: TWTR) (2018). Twitter Third Quarter 2018 Results. Twitter, Inc. Retrieved from EDGAR.com
- Facebook, Inc. (NASDAQ: FB) (2018). Facebook Third Quarter 2018 Results. Facebook, Inc. Retrieved from EDGAR.com
- Laughlin, L. (2018). Breakingviews – Pinterest shapes up for anti-millennial IPO. Reuters. August 8, 2018
- Louis, T. (2013). How Much Is A User Worth?. Forbes. Retrieved from http://www.Forbes.com.
- Castillo, M. (2018). Pinterest nears $1 billion in ad sales and valuation rises as it looks to go public in mid-2019. CNBC. Retrieved from http://www.cnbc.com.
- Melendez, S. (2016). How Pinterest Uses Machine Learning To Keep Its Users Pinned. Fast Company. December 2016 / January 2017, p.p. 40 – 41.
- Zhong, C., Karamshuk, D., & Sastry, N. (2015). Predicting Pinterest: Automating a Distributed Human Computation. Proceedings of the 24th International Conference on World Wide Web,1417-1426.
- Wagner, K. (2017). Pinterest expects to make more than $500 million in revenue this year. Recode.Retrieved from http://www.recode.com
- Text and graphics modified. Original source: shopify.com. How Pinterest Drives Online Commerce.Retrieved from http://www.shopify.com
- Martin, J. (2014). Behavioral Segmentation of Pinterest Users. Proceedings of the 3rd Workshop on Data-driven User Behavioral Modeling and Mining from Social Media, 2014 (November), 13-14.
- Lee, N. (2018). Pinterest rolls out product recommendations for easier shopping. Engadget. Retrieved from http://www.engadget.com.
- Wagner, K. (2017). Pinterest expects to make more than $500 million in revenue this year. Recode.Retrieved from http://www.recode.com
- Sevitt, D., & Samuel, A. (2013). How Pinterest Puts People in Stores. Harvard Business Review, 91(7/8), p.p. 26–27.
I really appreciate this analysis of Pinterest’s ability to provide value-add to retailers and consumers looking to make purchases (versus users that want Pinterest only for brainstorming or aesthetic purposes). In response to your second question, I don’t think a potential Pinterest price comparison tool presents too much of a marginal threat to retailers. The prevalence of the Internet already makes comparison shopping incredibly easy, regardless of whether a shopper is either online or in-store.
For segmentation, I am curious if Pinterest can tell whether certain demographics are already significantly more likely to make purchases through the site or not — the company may not even need to do much work to segment its customer base!
I agree Pinterest should continue to use machine learning to provide its users with better recommendations as well as matching retailers with users that are likely to acquire specific products. But I do not agree that the focus Pinterest should have is growing its revenue per user. While the metric can be additive when looking at businesses for valuation purposes, it does not give us insight into the value that they are delivering and the potential for the business to really scale. Look at Yelp for example. The company has historically struggled to communicate its value proposition, but the revenue per user (in this case, small businesses) is north of $300. I think the #1 focus for Pinterest would be to create an environment that matches (potential) buyers and sellers in the most effective and efficient way.
A useful and well-written study of the current use of machine learning at Pinterest, and further opportunities for its application. As someone who is not very familiar with Pinterest, I enjoyed learning more about how Pinterest works and found this article informative yet easy to understand. It appears that machine learning opportunities in this space are similar to those that Amazon and other online retailers have, most importantly the ability to profile customers minutely through their purchasing behavior and anticipate their upcoming needs. Another interesting way in which Pinterest is exploring machine learning is through the introduction of an early version AI Pinterest bot in 2017, to address process improvement (available at: https://medium.com/@Pinterest_Engineering/introducing-the-pinterest-chat-extension-and-bot-for-messenger-a88ff9d77041).
The customer segmentation and marketing strategy framework presented in the article is highly useful. The classification of two distinct customer groups, price-conscious and trend-conscious, will prevent Pinterest from pushing products to price-sensitive customers simply because they are profiled for a specific product. This lens, when combined with the current product profiling method, will better enable sales to both customer groups. In response to the question posed at the end of the article, I think that customer segmentation should be on a matrix, to capture both buying behavior/price sensitivity (as proposed in the article) and preferences (current approach). Segmentation could also be at least one level deeper. As an example, for price-conscious customers, segmentation could also capture triggers of buying behavior, such as likelihood to purchase from a discount versus buy-one-get-one free product bundling. Whether this level of detail can be achieved in customer segmentation will be highly dependent on the level of machine learning capability at Pinterest.
In response to the other question posed, I think that there is a threat to retailers. If customers are able to see other brand options, this means that they are able to shop for better prices more conveniently. Despite this, the benefits of higher traffic and more exposure to brands outweighs this risk, especially as price sensitive customers are likely to shop around either way.
Thank you for sharing this piece on an interesting application of machine-learning! Your approach to profiling users into “how” they make purchases rather than “what” they purchase resonates a lot with me as particularly millennials are thought to place significant value on experiences. As a consequence, the shopping experience is gaining more and more importance relative to the materialistic value of the purchase.
That being said, I believe that there is still some value in Pinterest’s original segmentation into interests, frequency of action and sequence of actions, particularly as these “hard” segmentation parameters can be tracked reliably while assessing “price-consciousness” and “brand awareness” is much more challenging. I would be curious what segmentation accuracy level you believe machine learning could achieve and in what time span. With that in mind, rather than just inferring the softer from the harder segmentation, what would you think about a matrix segmentation that combines both segmentation approaches but retains their respective information value?
The threat posed by a price comparison tool will depend on the quality of the user segmentation, which is why I believe a matrix segmentation will be critical. If the segmentation quality is high, retailers will be able to employ near perfect discrimination and receive significant benefits from Pinterest’s value add. This will in turn drive revenue per user and hence valuation ahead of the company’s IPO.