Payment Fraud: The Hidden Cost of Doing Business and How First Data is Using Machine Learning to Protect Merchants from Fraud Losses
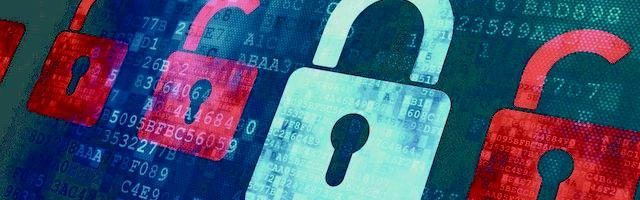
As the threat of payment fraud grows in the digital era, First Data has developed its own machine learning capabilities to improve the process of threat detection.
This year, it is estimated that fraud will cost merchants 1.8% of their total revenue−up 3.5x from 0.51% in 2013.[i] Increasing rates of fraud have been compounded by the proliferation of e-commerce and card-not-present transactions,[ii] which have become subject to rising threats as the rollout of EMV chips and secure readers have made in-store fraud more difficult to commit.[iii]
In June 2017, First Data, a global leader in merchant-oriented payment processing technologies, launched Fraud Detect, a comprehensive fraud scoring solution that utilizes machine learning to measure levels of fraud risk across its customers’ payment transactions.[iv] As online threats have become increasingly complex and virulent, the use of machine learning to improve fraud detection has taken on immense importance for payment processors like First Data.
Process Improvement through Machine Learning
Unlike traditional threat detection measures, which typically rely on manual rules-based engines, machine learning allows for the dynamic detection of new, potentially unknown threats. While rule sets are excellent at detecting known threats based on past bad behaviors (see Exhibit 1), most rules-based engines are inherently manual, reactive, and static−leaving merchants exposed to risk as sophisticated fraudsters inevitably learn to evade these rules.
Exhibit 1: Traditional Rules-Based Engines in Online Fraud Detection
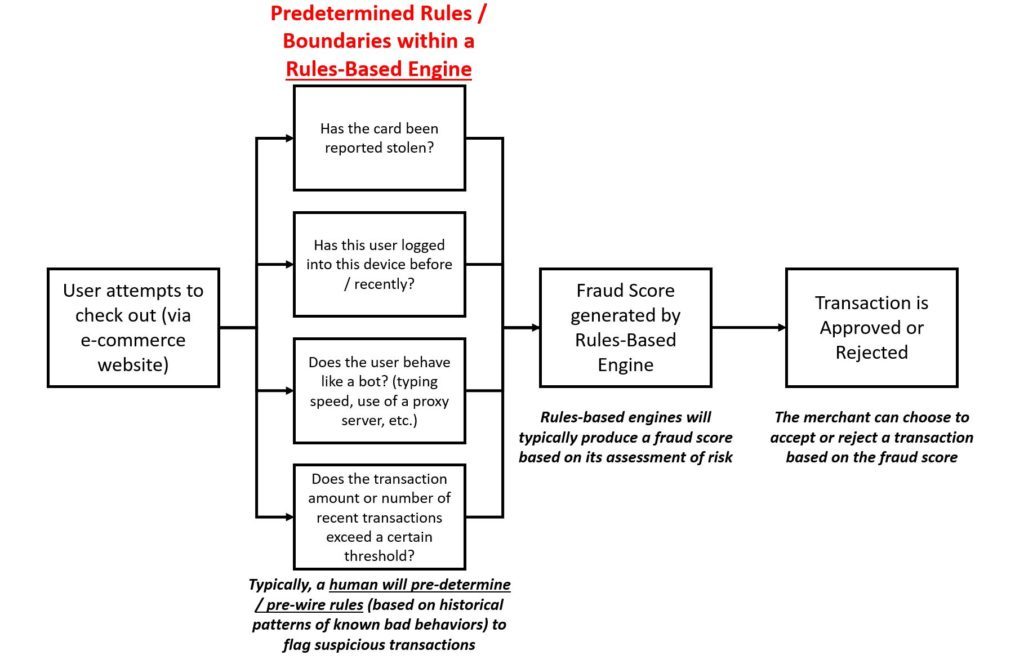
First Data believes that machine learning offers significant advantages in the arms race against fraud. Rather than requiring constant and manual setting of new rules or boundaries in response to new threats, machine learning can automatically and proactively detect more detailed and accurate relationships in massive data sets by searching for anomalous behaviors, as opposed to a pre-defined set of bad activities (see Exhibit 2).
Exhibit 2: Machine Learning’s New Approach: Anomaly Detection

First Data’s Strategy
Since the launch of Fraud Detect, First Data has been focused on deploying its solution across its existing customer base of over 6 million merchant locations.[v] By signing up more merchants onto the platform, First Data will not only establish a competitive moat around its fraud solutions, but it will also improve the quality of its algorithms, as precision improves with more transaction data. Processing over 93 billion transactions annually, First Data has the potential to better discern patterns and predictive measures through rigorous analysis than competitors who have more limited access to data and merchants.[vi]
In this near-term expansion plan, speed-to-market will be critical. First Data should focus first on signing up its largest merchants (possibly even at steep price discounts), who represent a disproportionate share of transaction volume, to get a critical mass of data quickly. In conjunction, First Data should target merchants across a variety of verticals and regions to understand the potential nuances in fraud risk across industries and geographies. Most importantly, the merchants that First Data does sign up for its Fraud Detect platform should all be contractually required (or financially incentivized) to report back actual instances of fraud to help close the feedback loop on First Data’s algorithms and improve their predictive power.
Longer-term, First Data is setting the foundation to grow beyond a global payment processing company and become a “market leader in security and fraud.”[vii] As we see with the launch of Fraud Detect, First Data is looking to develop homegrown competencies in machine learning to leverage its massive amount of payment data and establish broader capabilities in big data.
To achieve this vision, First Data should focus on how Fraud Detect can be complemented with other fraud detection methods over time. As the industry adage goes, there is no “silver bullet” for beating fraud−fraudsters have always found ways around past detection measures. While threats will continue to evolve, perhaps even with machine learning-driven countermeasures from fraudsters, remaining focused on exploring new frontiers for combating fraud will be key to First Data’s long-term success.
Ultimately, machine learning will continue to be a critical component in First Data’s product development road map, especially within its emerging suite of security and fraud solutions, but key questions remain:
- Given that fraud constantly evolves and finds ways to circumvent new detection measures, how will fraudsters potentially adapt to machine learning techniques?
- How much fraud can be avoided, practically? How much will fraud just be a cost of doing business in the digital era?
(796 words)
References:
[i] LexisNexis Risk Solutions. 2018 The True Cost of Fraud. Accessed 13 Nov 2018.
[ii] Card-not-present transactions typically refer to online transactions, as opposed to card-present transactions, which generally take place in-store or offline, where merchants can verify the details and existence of a card and / or cardholder.
[iii] Groenfeldt, Tom. “As EMV Chips Make In-Store Fraud Harder, Fraudsters Move Online”. Forbes, 2018, https://www.forbes.com/sites/tomgroenfeldt/2017/02/28/as-emv-chips-make-in-store-fraud-harder-fraudsters-move-online/#75ffbef6493a. Accessed November 2018.
[iv] “First Data Launches New Merchant Solution to Prevent Fraud Across All Commerce Channels”. First Data, 2017, https://www.firstdata.com/en_us/about-first-data/media/press-releases/06_01_17.html. Accessed November 2018.
[v] First Data Corporation, October 29, 2018, Form 8-K, p. 6, www.sec.gov/Archives/edgar/data/883980/000088398018000043/a9302018newexhibit991.htm. Accessed November 2018
[vi] First Data Corporation, June 12, 2018, Analyst/Investor Day Transcript, via CapitalIQ. Accessed November 2018.
[vii] First Data Corporation, June 12, 2018, Analyst/Investor Day Presentation, via CapitalIQ. Accessed November 2018.
Mike, great commentary on First Data. I think it is really important for a company like First Data to keep its data set and metrics secure otherwise “fraudsters” will just copy the behaviors to get around being detected as fraud. For example, in the simple rules-based approach, all a fraudulent purchaser would have to do is follow those steps to get around being detected. Going forward, while machine learning will have a multitude of behaviors to look for, since its comes down to statistics and certainty, all the fraudster would have to do is be really “human” in a few areas to trick the machine learning algorithm.
Mike, very informative and intuitive description of how machine learning is being utilized by First Data to try and get ahead of the move to more complex fraudulent behavior in the digital marketplace. I fully agree with your short for First Data – getting to market quickly and targeting your largest data source will allow your machine learning processes to build it’s data set to base it’s decisions on. Long term, I am curious how practical it is for a payments company to migrate into the big data industry in an age of “internet of things” with companies becoming more inundated with copious amounts of data.
I do wonder how much the 3.5x increase in fraud from 2013 is due to fraudsters already employing machine learning or other advanced tactics. My read of this increase of fraud is that either (or both) 1) fraudsters are already becoming increasingly more technologically advanced or 2) payments companies have been lagging behind in keeping up to speed with it’s fraud detection practices. If it turns out to be the second case, then there is the potential for a steady state of fraud to be reduced below its current level and to return to the 2013 levels or lower with the incorporation of machine learning to course correct. However, if First Data is simply catching up with the fraudsters, then this reactionary movement could lead to First Data constantly being behind the fraudsters.
Fantastic article – as others have mentioned this is a really great way to frame up the security challenges in payments and the role of big data analysis in security. I think payments companies are highly advantaged in providing/partnering with others to provide dynamic fraud detection solutions given their large repository to historical transaction data. Payments processors have been utilities historically and players of scale have held an oligopoly in the space among large merchants, as the space becomes more competitive the ability for payment processors to aid in fraud detection and provide other KYC capabilities to their merchants may be a powerful differentiator. I agree with your concern that fraudsters are also innovators that will adapt to machine learning solutions. This is why in the long term I think it’s important for the broader payment processing industry to partner with one another as well as other financial institution players to share a data depository to improve the accuracy of big data solutions combating fraud. This will make it increasingly difficult innovative fraudsters.
Mike, thank you for sharing this very important piece! It’s great to learn how machine learning is being used in the financial services industry, an industry that is highly susceptible to fraud activities. The focus of highlighting First Data use of technology to protect merchants from fraud losses was very interesting. A great example of a company having an access to such a large data set and was able to make use of its 6 million company customer data set. I’m curious to hear about your thoughts of other industries or even specific companies that would benefit from First Data’s capability around machine learning and big data–even beyond the usage of combating fraud. Thanks again for sharing!