Partners HealthCare: Machine Learning to Improve the Patient-Doctor Experience
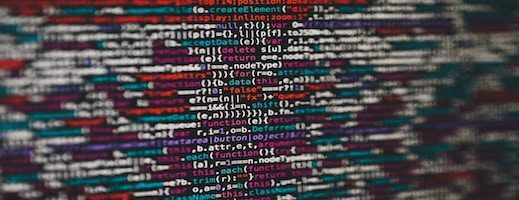
Many U.S. physicians feel that health care digitization has created serious headaches with limited benefits for patients. Will machine learning soon change their minds? Partners HealthCare, a health care system that oversees two world-class Boston hospitals, certainly thinks so.
Picture this. You visit your doctor, who is 20 minutes behind schedule. You eventually see them for 15 minutes, of which they spend 50% of the time typing notes, constantly clicking their mouse, and staring at their computer screen. The doctor then performs a lightning-fast exam, rapidly relays essential information, and sends you for a test. Before you know it, the visit is over and you leave the clinic feeling confused and unsatisfied. Hours later, you receive a phone call about your test result.
Unfortunately, the above situation is far too common, leaving patients and physicians feeling increasingly frustrated. The culprit? Poor design and implementation of the last wave of digital health technology driven by the electronic medical record (EMR).
In a recent New Yorker piece entitled “Why Doctors Hate Their Computers,” Atul Gawande, a world-renowned surgeon and CEO of Amazon’s healthcare venture, wrote, “A system [EMR] that promised to increase my mastery over my work has, instead, increased my work’s mastery over me.”[1] These unfavorable dynamics have driven physician burnout to an all-time high, with over 50% of physicians experiencing symptoms of burnout.[2]
EMR technology has left a bad taste in physicians’ mouths by costing them unnecessary time and mental energy. Partners HealthCare, which includes Massachusetts General Hospital (MGH) and Brigham and Women’s Hospital (BWH), is developing the next wave of health care technology to reverse this trend and empower its physicians. How? By using machine learning.
Partners emphasized its commitment to machine learning by establishing the MGH and BWH Center for Clinical and Data Science (CCDS) in 2016.[3] Partners has since positioned itself for long-term success by creating partnerships with key industry leaders. In 2017, the CCDS announced a 10-year collaboration with General Electric to “integrate deep learning technology into every aspect of the patient journey.”[4] More recently, CCDS has partnered with Nuance Communications, a company that specializes in open-platform artificial intelligence development. Through this agreement, CCDS will focus on translating machine learning technology into solutions for radiologists who interpret medical images.[5] Multi-disciplinary teams consisting of physicians, data scientists, and engineers will greatly enhance these collaborations’ chances of success.
While Partners clearly recognizes the long-term potential of machine learning, how quickly can we expect this technology to impact patients? Remarkably, MGH researchers have already produced exciting results. In a 2018 study published in Nature, a preeminent scientific journal, researchers enhanced medical images through a machine learning technology called AUTOMAP.[6] In the clinical setting, this technology could allow for faster, more accurate interpretations by radiologists. Now, imagine that the test performed in the earlier scenario is a medical image that is vital for your doctor to establish a diagnosis. Instead of anxiously waiting hours for the results, AUTOMAP could allow your doctor to more quickly and confidently reveal the results to you in minutes, leaving more time for discussion of your questions and concerns.
Another encouraging MGH study showed that a machine learning algorithm could accurately predict which emergency department patients with bloodstream infections would need potentially life-saving medication.[7] With this algorithm, instead of reacting to complications that arise, doctors could proactively administer the important medication and prevent complications.
These discoveries could improve the speed and quality of diagnoses and treatment plans. This would create more time for physicians to counsel patients and answer questions, ultimately enhancing trust and satisfaction with the patient-doctor experience.
As Partners strives to unleash the full potential of machine learning, it should consider the following recommendations:
- Emphasize machine learning as being complementary to physicians as opposed to a substitute for them to encourage enthusiastic buy-in. This notion has been supported by commentary in academic journals.[8],[9]
- Establish an open-access manual of machine learning best practices based on current projects. It is important to hasten the innovation process in a field like health care that is inherently slow to adopt new technology due to patient safety concerns.
- Ensure that operational efficiency gained through machine learning is allocated to improving the patient-doctor experience and not to increasing patient volume. This could be accomplished by measuring physician and patient satisfaction after implementing new technology.
As health care organizations grapple with this new technology, the following questions are interesting to consider:
- Who is at fault if a machine learning-driven decision results in a medical error?
- How can bias be minimized in health care machine learning algorithms?
While many details must be worked out, Partners HealthCare is at the cutting-edge of using machine learning to improve the patient-doctor experience and health care more broadly. The innovations discussed here are just the tip of the iceberg.
(798)
[1] Gawande, A. (2018, November 06). Why Doctors Hate Their Computers. Retrieved from https://www.newyorker.com/magazine/2018/11/12/why-doctors-hate-their-computers
[2] Shanafelt, T. D., Hasan, O., Dyrbye, L. N., Sinsky, C., Satele, D., Sloan, J., & West, C. P. (2015). Changes in Burnout and Satisfaction With Work-Life Balance in Physicians and the General US Working Population Between 2011 and 2014. Mayo Clinic Proceedings, 90(12), 1600–1613. https://doi.org/https://doi.org/10.1016/j.mayocp.2015.08.023
[3] Mass General News. (2017, April 7). Bringing the latest advances in artificial intelligence to patient care. Retrieved from https://www.massgeneral.org/News/newsarticle.aspx?id=6264
[4] Copp, R., & Sousa, K. (2017, May 17). Partners HealthCare and GE Healthcare launch 10-year collaboration to integrate Artificial Intelligence into every aspect of the patient journey. Retrieved from https://www.partners.org/Newsroom/Press-Releases/Partners-GE-Healthcare-Collaboration.aspx
[5] Nuance Communications, Inc. (2018, March 05). Nuance and Partners HealthCare Collaborate to Accelerate Widespread Development, Deployment and Adoption of AI Applications for Diagnostic Imaging. Retrieved from https://globenewswire.com/news-release/2018/03/05/1415228/0/en/Nuance-and-Partners-HealthCare-Collaborate-to-Accelerate-Widespread-Development-Deployment-and-Adoption-of-AI-Applications-for-Diagnostic-Imaging.html
[6] Zhu, B., Liu, J. Z., Cauley, S. F., Rosen, B. R., & Rosen, M. S. (2018). Image reconstruction by domain-transform manifold learning. Nature, 555, 487. Retrieved from https://doi.org/10.1038/nature25988
[7] Matheson, R., & MIT News Office. (2018, November 07). Machine-learning system could aid critical decisions in sepsis care. Retrieved from http://news.mit.edu/2018/machine-learning-sepsis-care-1107?fbclid=IwAR24dS96H6t6_7GIbH4JVv0OMyW0dzh-nCHLk1TrVoq77gXgGuCJVErvnnM
[8] Fogel, A. L., & Kvedar, J. C. (2018). Artificial intelligence powers digital medicine. Npj Digital Medicine, 1(1), 5. https://doi.org/10.1038/s41746-017-0012-2
[9] Verghese A, Shah NH, Harrington RA. What This Computer Needs Is a Physician: Humanism and Artificial Intelligence. JAMA. 2018;319(1):19–20. doi:10.1001/jama.2017.19198
[10] Featured Photo by Markus Spiske on Unsplash
The Atul Gawande quote was very powerful and encapsulates the challenge at hand very well. It’s great that machine learning could accelerate how quickly patients get results. The risk of a machine medical error is scary, and would likely be received critically by the general public. I wonder if machine learning could also expose human bias rather than replicating it.
I believe that training machine learning algorithms using high quality, representative datasets would minimize bias. I would be concerned about bias if the algorithm is trained on a database enriched for certain characteristics. For example, if the data set includes a high number women and high number of cardiovascular events, then the algorithm may learn to associate cardiovascular events with women. However, I believe a great benefit of machine learning algorithm is that the process reduces human bias that naturally occurs during an in-person physician-patient appointment.