Partners HealthCare: Leveraging Machine Learning to Predict Heart-Disease
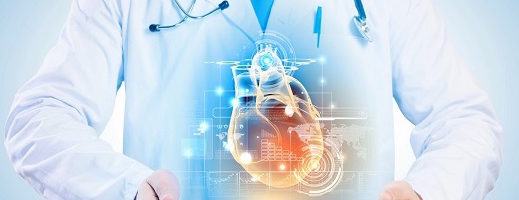
Faced with ongoing U.S healthcare reform that forces hospitals to bear financial risk for patient outcomes, Partners Healthcare should embrace the power of machine learning to cut costs and improve quality of care
Faced with ongoing U.S healthcare reform that forces hospitals to bear financial risk for patient outcomes, Partners Healthcare (Partners) should embrace the power of machine learning to cut costs and improve quality of care. In particular, better analytical tools that integrate multiple health data sources, from electronic health record (EHR) data to fitness tracker output, to accurately predict heart disease complication risk could save Partners tens of millions of dollars per year.
Across the U.S., machine learning techniques hold the potential to significantly reduce the $10+ billion of preventable annual hospitalization costs attributed to heart-disease.[i] The current standard for evaluating risk in heart-disease patients, the Framingham 10-year risk score, is inaccurate in certain populations, especially in women and minority groups.[ii] A more accurate and less biased machine learning-based prediction tool would better identify high-risk patients for heart attack, stroke, and hospitalization, allowing better preventative measures. For example, patients determined high-risk by the prediction tool could be targeted with additional doctor’s appointments, screening tests, and medication adjustments to prevent costly complications requiring hospitalization.
Such a tool has already been built and validated by researchers in Boston using using EHR data.[i] These researchers found that their tool predicted heart-related hospitalizations with an accuracy of 82% compared with 56% for the Framingham 10-year risk score.[iii] However, it is safe to assume that a machine learning algorithm that integrated data sources in addition to EHR data, such as retinal fundus images and fitness tracker data would be even more accurate at predicting hospitalizations. A Google research group recently applied deep learning techniques to retinal fundus images alone and was able to predict 5-year risk for Major Adverse Cardiac Events as accurately as the current risk prediction standard.[iv] The predictive capacity of machine learning to evaluate cardiac risk based on multiple data inputs is immense.
With the ongoing transition from fee-for-service to value-based reimbursement, Partners is under pressure to find novel ways to both control costs and improve outcomes. Leveraging the predictive capacity of machine learning offers a potential solution. The Affordable Care Act encouraged the creation of Accountable Care Organizations (ACOs), networks of doctors and hospitals that share medical and financial responsibility for providing coordinated patient care. The ultimate goal of ACOs is to increase efficiency and quality of care through financial incentives for not duplicating services and preventing medical errors.[v] Since 2012, Partners, the largest healthcare provider network in New England, has entered ACO contracts with all major insurers, including Medicare, commercial payers, and MassHealth. Ultimately, Partner’s ACO contracts rewards it for avoiding unnecessary hospitalizations and preventing progression of chronic diseases, like heart disease. With preventable heart-related hospitalizations accounting for over 30% of total preventable hospitalizations, leveraging a machine learning prediction tool to avert these unnecessary heart-related hospitalizations would generate for Partners significant financial rewards in its ACO contracts.[i]
To adjust to the the new ACO reimbursement framework, Partners has expanded its population health management programs, namely care coordination, behavioral health, and substance use disorder programs.[vi] These initiatives allow Partners to better address the social determinants of health, such as housing and education, that play a large role in health outcomes. In the first five years of its Medicare ACO, Partners saved $38 million and achieved a quality score of 94.5%, among the highest for ACOs in the country.[vii] However, despite these early successes, Partners has increasing incentives to further improve given the rising risk burden of its ACO contracts, especially its MassHealth ACO contract, and the uncertain future of federal healthcare legislation.
So how would machine-learning prediction tools be integrated with Partners’ population health management programs for heart-disease? First, every patient in the Partners system would be screened for heart-disease progression and hospitalization risk using an EHR-data derived machine learning algorithm. Second, those screened to be high-risk would be monitored with regular retina fundus images and a fitness tracker. Finally these additional data sources along with the original EHR data would be used as inputs for an even more accurate machine learning-based prediction tool. With better predictions of disease progression risk, Partners’ population health management initiatives could be better targeted and streamlined. The end result would be higher financial rewards for Partners and better care for patients.
Providing the best quality healthcare to patients at the lowest cost to society must be the mission of large healthcare systems like Partners, especially as financial incentives are aligning to drive this mission. By leveraging machine learning techniques to prevent and reverse the progression of heart-disease, Partners has the potential to not only to save considerable money, but also radically improve the trajectory of its patients’ heart disease.
(763)
[i]Dai, Wuyang, et al. “Prediction of Hospitalization Due to Heart Diseases by Supervised Learning Methods.” International Journal of Medical Informatics, vol. 84, no. 3, 2015.
[ii]Ioannis et al. “Machine Learning Outperforms ACC/AHA CVD Risk Calculator in MESA.” Journal of the American Heart Association. 2018.
[iii] Paschalidis, Yannis. “How Machine Learning Is Helping Us Predict Heart Disease and Diabetes.” Harvard Business Review, 30 May 2017, hbr.org/2017/05/how-machine-learning-is-helping-us-predict-heart-disease-and-diabetes.
[iv] Poplin et al. “Prediction of cardiovascular risk factors from retinal fundus photographs via deep learning.” Nature Biomedical Engineering volume 2, pages158–164. 2018.
[v] Gold, Jenny. “Accountable Care Organizations, Explained.” Kaiser Health News. 2015
[vi] “Partners to participate in innovative Medicaid program to improve patient care, reduce costs” Partners Patient Care. August 2017. https://www.partners.org/Newsroom/Press-Releases/Partners-Participates-MassHealth-ACO-Medicaid-Program.aspx.
[vii]“Accountable Care Organization Overview” Partners. 2018. https://www.partners.org/for-patients/ACO/ACO-Overview.aspx.
I think you did a great job framing the challenge of transitioning to value-based care and the opportunities for machine-based learning in this space. Our ability to gather immense amounts of patient data is quickly outstripping our ability/time to meaningfully process and leverage this data. New technologies tout the ability to almost continually monitor heart rate, blood pressure, glucose, and any number of other physiologic metrics. Currently, however, this begs the question of: so what? Ten thousand heart rate measurements are meaningless unless they can be placed in the context of a patient’s baseline physiology and then used as a warning sign for impending medical disease or deterioration when the patient strays from that baseline. Few physicians have time in their clinical work lives to process such immense amounts of data, but machines can.
This is a really interesting idea. A few questions/comments for you:
1) Do you think that this machine learning algorithm would lead to a large shift in the way that physicians actually practice medicine? As you mentioned in your write up, the medical community currently uses the Framingham risk score, which is flawed, but there is nevertheless a large amount of study data that shows that this risk score is useful in identifying which patients are at risk for heart disease (and therefore which patients should be placed on heart medications like statins). How much of an incremental improvement do you think this algorithm will actually have on identifying patients at risk for heart disease?
2) Given that the Framingham risk score is already so entrenched in medical practice, it would be a great deal of work for clinicians (both at Partners and elsewhere) to switch their practice to a machine learning algorithm. How would you go about convincing physicians (many of whom have been using the Framingham risk score for years) to switch to a new machine learning algorithm? Additionally, how would you drive buy-in from physicians outside of the Partners network?
This is an amazing idea that will probably turn around the entire healthcare industry. Thinking of the way it is implemented I see a possibility the people may be changing their behavior knowing what data is used for making predictions on their health conditions which in turn may influence their insurance costs. Such behavior changes may be both positive (like running more to have better data on one’s fitness tracker) and negative (like actually refusing to use fitness tracker at all so that the system has less information on a person).
This was an interesting read about one the many potential applications of machine learning in healthcare. Your article touched on a couple of key challenges that I would like to press on further as I believe they meaningfully stall the potential application of the above tool.
As Sam noted above, the Framingham risk score is part of clinical practice today. In medicine, it typically takes about 8-10 years for clinical guidance published in literature (e.g., academic journals) to be widely adopted by physicians in their practice. This would make it very hard for the above program to gain traction and lead to behaviour change in the short term. This is further exacerbated, by two other factors you discussed: reimbursements, and tools needed to implement the program.
On reimbursements: While Partner’s has been moving meaningfully to risk-based contracts, MA is on the forefront of these types of contracts nation-wide. Large swathes of the country do not have the incentive structures in place for this program of early detection and monitoring to be financially viable.
On tools: Partner’s is one of the largest academic medical centers in the country, with many resources at its disposal. Its EHR has been customized to contain more data than typical home-grown or smaller scale EHRs at hospitals with fewer resources. I worry about the potential applicability of these programs to community-based hospitals throughout most of the country. Would they ever be able to implement this?