OrCam: A New Vision for Machine Learning
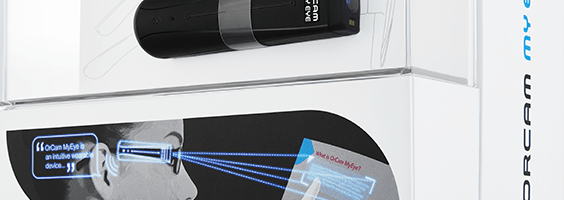
Can machine learning help people see again? OrCam says yes.
In late 2013, Israeli computer scientist Amnon Shashua and entrepreneur Ziv Aviram introduced the OrCam MyEye, a wearable visual assistant “system” – part computer, digital sensor, speaker and machine learning algorithm(s) – aimed at improving the lives of the visually impaired, a population numbering more than 20 million in the U.S.[1] and over 285 million worldwide[2]. These include people afflicted by medical conditions such as macular degeneration, cataract and diabetic retinopathy as well as others who have suffered vision loss in military combat. The device is about the size of your finger, weighs just under an ounce and costs $2,500, roughly the price of a good hearing aid.[3]
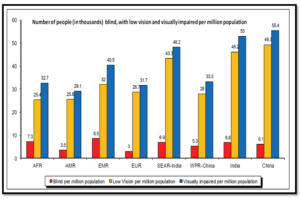
Design Spec(tacle)s:
While wearing the MyEye attached to one’s glasses, a user points at whatever s/he wants the device to read and the device’s camera, upon recognizing the human’s outstretched hand, takes a picture of the text – be it a billboard, food package label or newspaper – and, after running the image through its algorithms, reads the text aloud. Leveraging supervised learning technology, the product is trained on millions of images of text, products, and languages so that it can identify and interpret the proper image when it comes into view.
While OrCam began as a “AI-native” technology, advancements in machine learning have pushed the boundaries of what the MyEye is able to do – and created challenges for Shashua and Aviram as they seek to further develop their product.
Seeing Around Corners:
Existing product improvements: OrCam has capitalized on ML advancements to improve its core product. MyEye benefits from greater image capture capabilities as the underlying software is continuously trained on new formats of text such as new fonts, sizes, surfaces and lighting conditions. It can now identify whether there is sufficient natural light to capture an image and can recognize when an image is upside down and clue the user to flip the item. In addition, MyEye can announce color patterns (useful for dressing oneself in the morning), recognize millions of products and store additional objects like credit cards or grocery items.
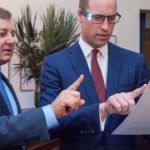
New product features: Beyond text, OrCam has focused on adding facial recognition capabilities, which similarly harness the power of machine learning. Through supervised learning, users can record family members faces in <30 seconds[4] and the device will cycle through its programmed dataset to identify this person the next time s/he comes into view. Further, the device can parse unstructured inputs (e.g., new faces) to give the user clues about bystanders it doesn’t recognize (“it’s a young woman in front of you”).[5]
Challenges for 20/20 and Beyond:
One of the classic tensions with wearable technology surrounds how much intelligence is stored on the device versus in the cloud. On the one hand, the device should be aesthetically light and easy to use (the initial product was burdened by a clunky cable and base unit), but on the other, it should be “big” enough to process, store and compute a lot of data. One option to overcome the constraint of limited built-in memory is using real-time cloud storage, but doing so consumes a large amount of power and drains the battery (today’s battery life is ~2 hours[6]), not to mention raising a host of user privacy issues if the device is synced with other personal, cloud-based apps.
How better to solve this problem than by turning ML capabilities inward to make the product itself function more efficiently? For example, the MyEye can harness machine learning to decide when to process new images (defer processing when in “low battery” mode), which ones to process (prioritize those with sufficient light exposure) and how to regulate its overall database (delete redundant images or re-capture a low-resolution image). [7] In addition, by training its image sensors to re-aim when an object is only in partial view, the MyEye will be able to process information more accurately and, importantly, efficiently, as it no longer wastes time matching incomplete data. [8]
Future Optics:
One key question for OrCam surrounds how far it should expand its target market. The basic product design – which required a user to point to an object to trigger the reading system – was geared for the “low vision” market; in fact, it precluded “fully blind” individuals who wouldn’t know where to point. However, much more potential exists to embed the product into daily life for the visually impaired and beyond. What if OrCam could leverage its accumulated dataset to send corresponding information about an individual – such as name, birthdate, or last time of meeting – once it recognizes that person’s face?[9]. Or, if the device reads McDonald’s labels every Sunday, can it make statistical inferences to recommend coupons associated with these user preferences? Overall, how should OrCam weigh increasing the MyEye’s functionality and mass market appeal against protecting user privacy and maintaining a consumer-friendly, wearable design?
(Word count: 798).
Citations:
[1] Erik Brynjolfsson and Andrew McAfee. “The Dawn of the Age of Artificial Intelligence.” The Atlantic (February 2014).
[2] “Global Data on Visual Impairments.” World Health Organization (2012).
[3] Katharine Schwab. “The $1 Billion Company That’s Building Wearable AI for Blind People.” Fast Company (May 2018).
[4] Alex Lee. “My Eye 2.0 uses AI to help visually impaired people explore the world.” Alphr (February 2018).
[5] Romain Dillet. “The OrCam MyEye helps visually impaired people read and identify things.” TechCrunch (November 2017).
[6] Katharine Schwab. “The $1 Billion Company That’s Building Wearable AI for Blind People.” Fast Company (May 2018).
[7] Yonatan Wexler and Amnon Shashua. “Apparatus for Adjusting Image Capture Settings.” Patent No. US 9,060,127 B2. United States Patent Office (June 2015).
[8] Yonatan Wexler and Amnon Shashua. “Apparatus and Method for Analyzing Images.” Patent No. US 9,911,361 B2. United States Patent Office (March 2018).
[9] For HBO’s Veep fans, think of this as a real-life Gary Walsh to our Selina Meyer!