NewYork-Presbyterian: Becoming the Hospital of the Future
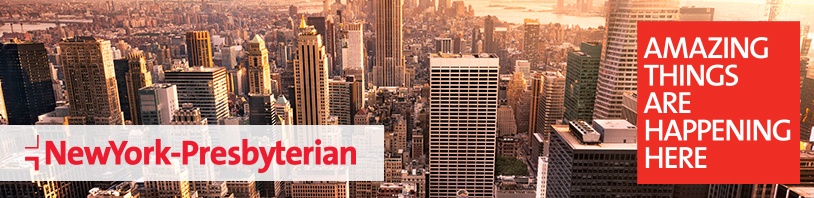
NewYork-Presbyterian hospital is using machine learning technology to streamline hospital operations, free up more time for clinicians, and improve overall quality of care.
How is NewYork-Presbyterian using Machine Learning?
Physicians are required to handle large amounts of data, from macro-level physiology to micro-level lab and imaging studies. The growing amount of patient data and the increasing requirements for doctors to document notes on electronic medical records has made it very difficult for doctors to manage all this information while still providing optimal care for their patients [2]. This has resulted in doctors spending more time on computers than in face-to-face interactions with their patients [3]. At NYP, machine learning is being used to monitor patients and to alert doctors and nurses when data is clinically-relevant, which is allowing doctors to spend more time with patients.
To do this, NYP created the Clinical Operations Center, or CLOC, which is an off-site support building where nurses use machine learning to monitor patients at the hospital. It works by having automated patient monitors which send data in real-time to CLOC. Nurses at CLOC monitor the data and machine learning is used to separate non-priority data from priority data. When an intervention is required, a notification is sent to the hospital staff. NYP has reported multiple benefits of this remote monitoring system, including: 1) doctors can spend more time with their patients, 2) alarms dropped from 30,000 per week to only 100, 3) it reduced the number of redundant tasks, and 4) it reduced the number of staff needed on-site [4].
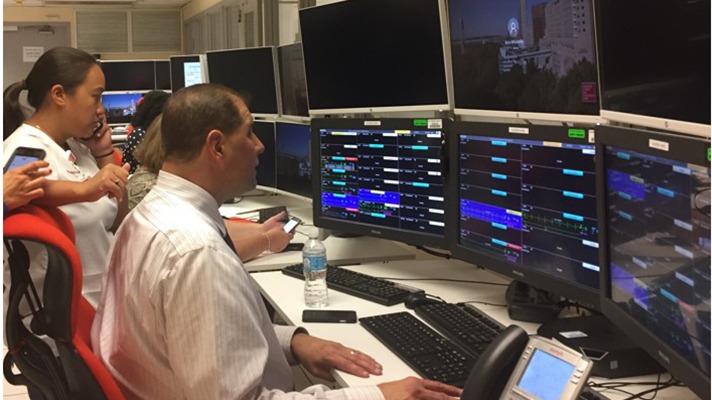
What issues are they trying to solve?
In the short-term, NYP’s goal is to “leverage machine learning at 100% capacity” to continue optimizing clinicians’ workflows. CLOC is already showing the benefits of machine learning in the daily clinical monitoring of patients. However, this monitoring system has been implemented in only 30 medical units at NYP. The hospital leadership has a vision for expanding this system across more hospital units [5].
In the medium-term, NYP is exploring new uses for machine learning beyond remote patient monitoring. Their focus is primarily in the operations space, which is unlike many health care companies that are using machine learning for diagnostics and decision-making tools. To do this, NYP established the “NYP Innovation Center” and hired a team that works on machine learning projects. The overarching goal is to use machine learning to extract insight from hospital data, and work with senior leadership to deliver live, automated, and actionable information to clinicians [6].
As the use of machine-learning grows, NYP will certainly face issues with the technology. First, there is a potential for bias. There is a risk that machine-learning will reflect doctor’s biases since they are inherent to the data we provide them. Second, there is a fiduciary patient-doctor relationship. The introduction of machines will call into question whether machines are also part of that relationship and whether data can truly be kept confidential. Finally, we question the overarching goal of machine-learning: is it to improve health care or to generate more profit? Or both? [7].
Recommendations for Growth
At a high-level, NYP uses machine learning to address operational inefficiencies within the hospital. Their first application is in patient monitoring and alarm systems, but there are certainly other areas where this technology can create efficiency in patient throughput and clinician workflow:
- Surgical scheduling systems: By using machine learning, NYP can create optimal operating schedules such that operating rooms spend less time being idle during patient turnarounds.
- Patient documentation: Currently, clinicians spend a significant amount of time interviewing patients and documenting notes on electronic medical records. Machine learning can be used to not only document notes, but also to recommend next steps in the treatment plan.
- Expand into diagnostics: This will be important as they compete to remain a leader in digital health and innovation. The industry is trending towards big data and improved diagnostics, especially in radiology and pathology.
Unanswered Questions
As machine learning continues to expand, the questions that remain are: 1) Can we maintain the patient-doctor relationship while still upholding confidentiality, 2) Can we effectively remove bias from the decision-making process, and 3) What is the fundamental goal of machine learning in medicine: to maximize profits or to shift clinicians’ time back to the patient?
(Word Count: 780)
Sources
[1] https://lowninstitute.org/news/blog/ehrs-desperately-need-reboot-new-studies-show/
[2] https://www.nejm.org/doi/full/10.1056/NEJMp1606181?url_ver=Z39.88-2003&rfr_id=ori%3Arid%3Acrossref.org&rfr_dat=cr_pub%3Dpubmed
[3] http://annals.org/aim/fullarticle/2546704/allocation-physician-time-ambulatory-practice-time-motion-study-4-specialties
[4] https://www.healthcareitnews.com/news/inside-look-newyork-presbyterians-ai-command-center
[5] https://www.nyp.org/news/NYP-Named-Top-10-Most-Innovative-Company-in-AI-by-Fast-Company
[6] http://www.innovatenyp.org/silvermanfellowship/
[7] https://www.nejm.org/doi/full/10.1056/NEJMp1714229?url_ver=Z39.88-2003&rfr_id=ori:rid:crossref.org&rfr_dat=cr_pub%3dpubmed
Ricardo, I think your essay is very interesting. Now is an interesting time for healthcare IT, where hospital systems have been accumulating data for years, and now we are on the cusp of truly generating value from that data. I think one of the challenges we may continue is that in order for the CLOC to function, it needs to be looking at some patient data. The big question is: what is the source of that data? Of course, some of that data can be pulled from monitors that are hooked up to the patients, removing the need for human intervention. But what about the data that, today, is only put into the electronic medical record by the physician [e.g. like diagnosis, treatment plan, etc]? How can we make that a less human intensive process so that the innovative machine learning solutions like the CLOC are able to function without needing the up front cost of physician data entry?
Great essay. Truly believe that healthcare has a lot to benefit from ML, especially in a context where costs keep rising and medical attention becomes a “luxury” good for people with financial stress. Mike pointed out an interesting aspect in his comment above which is where do we get the data from. Thinking ahead in the future, there will be a great opportunity to capture more relevant data in an automatic way with the widespread of wearables and the increasing sensors added to smartphones. This will add a lot of relevant automated input that could be used by health providers to prevent and/or act faster in emergencies. But I do see a big challenge in terms of privacy (as you also mentioned in your essay) not only on how we are going to keep that info secure but also on how much consumers are willing to share with health providers.
To echo the two comments above, I too am worried about what will happen to the patient-physician relationship when machine learning is used to provide recommendations to patients. I am not a physician, but from experience as a patient there is only so much that our vitals and other data points that can be collected by a “machine” tell a doctor about our condition. We must make sure that doctors are only using this information generated from machine learning to influence the treatment plan for patients, and I think that this will cut down on the amount of time doctors must spend behind the computer. However, my view is that we must continue to have doctors collect the more qualitative information that influences the treatment plan one way or another. To the data privacy issues, I think the use of de-identified data (PHI) is becoming more and more common in the medical field, but I do think that technology is advancing well enough to continue to figure out ways in which to protect this data in the cloud.
This is a thought-provoking essay, Ricardo. It’s exciting to read about a real-life application of machine-learning (ML) to solve real-world problems. Similar to the comments above, I do too worry about what will happen to the patient-physician relationship. The question of privacy laws aside, I wonder what will happen to trust in the relationship between patients and physicians. Will patients trust the ML recommendations and will they see a need for a physician (in the extreme case)? I do believe that ML learning can help decrease throughput time and improve the overall quality of a physician visit. My concern is more about whether patients will trust this new system, and the potential unintended consequence of new machine-learning programs (created by non-hospital supervised companies replicating the technology) giving diagnostic recommendations to former patients. It’ll be interesting to see how the application of ML evolves in the health-care space and how physicians and hospitals relationships with their patients evolves over time.
Great article – many thanks for sharing Ricardo. A recent study suggests that the U.S. spends about twice what other high-income nations do on health care but has the lowest life expectancy and the highest infant mortality rates [1]. I strongly agree with your analysis that AI has tremendous power to bring health care cost down. To many of the above comments and two questions you asked yourself at the end of the essay, I agree this could significantly change the doctor-patient relationship. What you and the other respondents have not talked about, however, is how this could also open opportunities to change the nurse-patient relationship or empower more bridges between nurses, docters and AI. There has always been a very big distinction between doctors and nurses: nurses are essentially not able to prescribe and always report under doctors. Given AI might provide more accuracy in diagnosis as well as an improved flow in operations, potentially nurses (or Nurse Practitionsers [2]) could take over a bigger part of the role as “trusted medical advisor”?
[1] Lisa Rapaport, “U.S. health spending twice other countries’ with worse results”, Reuters, MARCH 13, 2018, https://www.reuters.com/article/us-health-spending/u-s-health-spending-twice-other-countries-with-worse-results-idUSKCN1GP2YN, accessed November 2018
[2] American Association of Nurse Practitioners, “What’s a Nurse Practitioner (NP)?”, https://www.aanp.org/about/all-about-nps/whats-a-nurse-practitioner, accessed November 2018