Netflix & Machine Learning: To what extent should Machine Learning influence a strategic decision-making process?
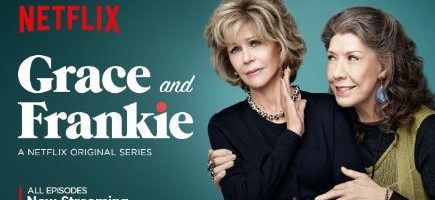
Netflix has been relying on its state-of-the-art movies recommendation engine to propel it to various new heights. Should it keep utmost faith on the engine that took the company to where it is today or is this the time to hit the break and bring back human judgement?
Netflix’s affection with machine learning and its current dilemma
Machine learning has been at the heart of Netflix’s business strategy and catalyst for growth since its inception in 1997. From the earliest form of its business model as a physical DVD-by-mail rental service, Netflix has started analyzing vast amount of user rating and behavior data to identify the type of movies its customers enjoyed watching in the past and developing proprietary algorithm to predict what movies the same customers will likely enjoy watching in the future. Netflix then utilized the result to recommend each individual customer a personalized playlist of movies & TV shows [1]. In addition, it leveraged the aggregate result to make an important business decision: determining which are the most popular contents it will need to buy from the DVD distribution companies and place in the rental library to anticipate future customers demand [2].
Riding the tailwind of internet proliferation phenomenon, Netflix began to convert its core business model into an online streaming service in 2007 [3]. The transformation allowed Netflix to deliver movies and TV shows instantaneously, reach new customers all around the globe, and significantly boost the number of subscribers. This new business model is a boon for its movie recommendation engine – the vast amount of fresh data helps to refine the algorithm and improve the prediction accuracy [4].
The increased confidence on the ability to predict customer’s taste led Netflix to a new venture – acquiring and creating its own original contents (movies and TV shows) [5]. While past decision on which DVD titles to be bought for its library is purely transactional, selecting and dropping casts have more risk and complex relationship effects. In one example, Netflix’s machine learning engine indicated that more users clicked on “Grace and Frankie” show when the image does not contain Jane Fonda. Netflix is struggling on whether to drop her from the marketing image at the risk of irritating the actress [6]. As experts think that similar tensions will occur more often, it’s decision today may set an important precedent on how the company approach similar types of problem going forward [7].
Current solution to balance the machine learning insight
The issue was stirring up internal debate within Netflix’s production and technology team. Production team “don’t believe numbers as much as people in Silicon Valley”; while technology team argued the data should not be ignored [7].
To resolve this impasse, the management team took on two-steps action plan. First, it cultivated the culture of open communication across different teams in voicing out the benefits and concerns of each options. Secondly, it encouraged the teams to also consider the “intangible impacts” that are not yet captured in the machine learning’s algorithm, such as the potential risk of alienating the actress, inability to partner with the actress for any future production titles featuring her, and repercussion of violating the contract agreement [7].
Under this framework, the two teams were able to “reach common ground”. Human judgement is introduced into the decision-making process – Netflix decided to keep the promotional image that also featured Jane Fonda [7].
Additional step to consider
In high level, I agree with the management’s approach to incorporate human judgement to overcome machine learning’s limitation in considering crucial “intangible impacts” that are out of scope for the algorithm. Augmented intelligence should serve as an input in the decision-making process, helping to suggest rather than dictate the final decision.
However, I think Netflix still can generate deeper insight to drive its business decision by conducting more rigorous analysis to separate the signal from the noise [8]. Currently the algorithm captures in one specific instance, there is negative correlation between Jane Fonda’s appearance in the image and the number of click. It does not tell convincingly whether it is truly because of Jane Fonda or other factors in the images such as color tone, background settings, etc. Having more result from other tests in a controlled environment will help to separate consistent relationship from the random correlation – it might even predict causality whether Jane Fonda is actually less desirable than her co-star, Lily Tomlin and drive the business decision in the future.
Open Questions
Netflix has been good at providing its customers new contents based on machine learning result of what they like in the past. However, in media and entertainment industry there is a saturation point where people are bored and weary watching the similar things again and again. What should Netflix do to avoid this situation from happening? Also, how should Netflix orient its business model to ensure it can capitalize on new trends – something that did not exist in the past hence not showing up in the machine learning result, but once introduced the customers will like in the future?
Word Count: 793
References:
[1] Netflix, Inc., “Company Timeline,” https://signup.netflix.com/MediaCenter/Timeline, accessed November 2018.
[2] Willy Shih, Stephen Kaufman, and David Spinola, “Netflix,” HBS No. 9-607-138 (Boston: Harvard Business School Publishing, 2007).
[3] Miguel Helft. “Netflix to Deliver Movies to the PC.” The New York Times, January 16, 2017, https://www.nytimes.com/2007/01/16/technology/16netflix.html, accessed November 2018
[4] Francois Brochet, Suraj Srinivasan, Michael Norris, “Netflix: Valuing a New Business Model”, HBS No. 9-113-018 (Boston: Harvard Business School Publishing, 2017)
[5] Gina Keating (2012). Netflixed: The Epic Battle for America’s Eyeballs. Portfolio/ Penguin.
[6] Travis Clark, “Netflix reportedly had an internal debate after its testing favored ‘Grace and Frankie’ promos without Jane Fonda.” Business Insider, November 11, 2018, https://nordic.businessinsider.com/netflix-tests-liked-grace-and-frankie-without-jane-fonda-wsj-2018-11, accessed November 2018
[7] Shalini Ramachandran and Joe Flint, “At Netflix, Who Wins When It’s Hollywood vs. the Algorithm?” The Wall Street Journal, November 10, 2018, https://www.wsj.com/articles/at-netflix-who-wins-when-its-hollywood-vs-the-algorithm-1541826015, accessed November 2018
[8] Mike Yeomans (2015) ‘What Every Manager Should Know About Machine Learning’, Harvard Business Review Digital Articles, pp. 2–6. Available at: http://ezp-prod1.hul.harvard.edu/login?url=http://search.ebscohost.com/login.aspx?direct=true&db=bth&AN=118667151&site=ehost-live&scope=site (Accessed: 12 November 2018).
What I found really interesting to consider in your article is your question regarding how Netflix can approach and predict new trends where the algorithm has no existing data to learn from. The very question as to how machine learning can introduce true innovation with no precedent data overall is an open question to me. From various discussions I have come to the belief/understanding though that advanced machine learning could possibly “solve for” innovation by looking at a vast majority of previous trends within an industry, e.g. film (or more specifically films at Netflix), and using this data to understand how new trends have emerged over time. Through such analysis, it could potentially come up with an algorithm that can predict what the next trend might be, even though it will seem to us very unexpected.
For now, it may be necessary for Netflix to use human judgment on top of machine learning if it is not sophisticated enough to distinguish “noise” from “signal”. However, if one were to use machine learning to predict innovation, one should be very careful to use human judgment on top of such findings. Humans tend to think of the future in terms of what they have already experienced and thus generally be bad at predicting trends that have not yet existed, hence us being “surprised” at “unexpected” trends. Applying our limited thinking to alter the findings of the algorithms can in such case have detrimental effects in predicting new trends. Thus, I agree with you that using human judgment on top of machine learning can be useful, but “not trusting data” because it does not seem “expected” could potentially fail to predict innovation.
I completely agree with your argument that the human element needs to be considered along with the data in making critical decisions. We have learned in class that machine learning is imperfect, and especially prone to bias. I actually think that companies like Netflix should also employ some traditional customer research methods such as surveys and focus groups to complement the data.
I am also intrigued by your question around how can Netflix capitalize on new trends, or “innovate” on its content, instead of creating more and more similar shows based on existing viewership data. I think two concepts can help here: 1) prototyping, and 2) open innovation. Netflix can figure out a prototype equivalent for content, for example, an animated trailer that illustrates the concept of the show. The goal is to have relatively cheap way of building the prototype, and allow customer reactions to test its commercial value. Another idea is that Netflix can leverage open innovation to allow its creative views to submit content ideas for the rest of the community to vote on.
This is an interesting paper for an interesting topic – two points called my attention:
1) correlation does not mean causation – the example on Jane Fonda presence is spot on, as sometimes data may propose ambiguous and sub-optimal results. It would be interesting to understand though whether in this specific case big data actually present this risk. In fact, this seems a problem of dynamic creative optimization (quite common in the advertising space), where with millions of consumers’ feedback it is easy to run experiments (A/B tests) by changing different parts of the creative (including the presence of Jane Fonda).
2) the past is not always a good predictor of the future – in the media and entertainment industry, like in many others, trends can abruptly change and machine learning (which focus on the past) might not be a good tool to help predict such changes, hence pushing companies (Netflix in this case) in a “follower” position. On the other side, as machine learning gets more sophisticated, it can also learn about specific patterns which are an early predictor of an upcoming change (similar to earthquake forecast of the dragon king theory in finance). Still, predicting a disruption, may not help in the case of the media and entertainment industry, where results are not binary (earthquake yes/no, financial crisis yes/no). Open question.