Microsoft’s FarmBeats Uses Machine Learning to Increase Crop Yields
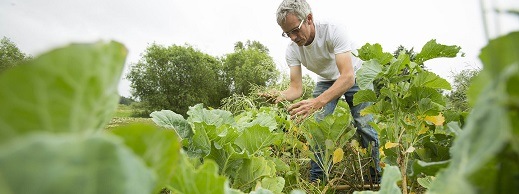
Through weather predictability, soil sensor data, drone imaging, and white space connectivity, Microsoft's FarmBeats seeks to use machine learning to increase crop yields and meet food demand that is projected to double by 2050.
With the projection of global food demand to double by 2050 due to population growth and higher average income, how will we continue to feed the world into the future? [1] Established in 2015, Microsoft’s FarmBeats seeks to answer this question with the application of machine learning through data acquired by soil sensors and drone imaging. Supplemented with weather predictability algorithms and white space connectivity, FarmBeats is designing a low-cost “precision agriculture” technology to best guide farmers on how to increase yields with minimal inputs [2]. See below for a video overview of their implementation at Dancing Crow Farm [7,8]:
Why is this megatrend important to Microsoft’s management of product development?
In 2014, 3.5% of the world’s GDP was related to agriculture, including 24% of developing nations’ GDP [3]. The UN recently forecasted the world population to grow to 9.8 billion people by 2050 and 11.2 billion by 2100, with this coming almost entirely from the developing world (Figure 1) [4]. Despite a growing population, crop yield growth rates have been slowly declining since 1962 (Figure 2) [5]. To address these concerns and to reduce cost inputs, “precision agriculture” arose with the goal of only giving water, pesticides, and fertilizer to the crops that need it. This would involve a precise surveillance of the land that was being used to grow crops, with the understanding that soil composition, acidity, and moisture levels can differ greatly in close proximities. Current techniques of “precision agriculture” cost around $1,000 per sensor, with substantial additional costs being associated with the data transfer from these systems to the farmer [6]. Microsoft’s FarmBeats saw this as an opportunity to use machine learning and cheaper connectivity to tackle this issue.
How is FarmBeats addressing these issues in the short term and medium term?
FarmBeats is currently in the product development and testing phases, with two key farms in Washington and New York as their centers for collecting data. In the short term, FarmBeats is addressing several key issues: the cost of connectivity and data transfer, lack of reliable power, and optimized drone flights to capture visual data. To address the current high costs of connectivity and data transfer, FarmBeats is using the TV “white space” (TVWS), or unused radio and broadcasting frequencies, to transfer live sensor field data (e.g. moisture level, acidity) from their base stations to a central PC (Figure 3). This removes the need for an expensive cellular data plan to send data. The central PC, called the “FarmBeats Gateway”, collects and analyzes this data on site without internet, and compresses the data to be about 1000 times smaller before sending it to a cloud to feed the machine learning program using a standard internet connection. To tackle the lack of reliable power, FarmBeats has installed cheap solar panels that utilize weather predictability algorithms to plan when and how to use limited power to collect and send data. This algorithm has allowed the system to remain online 100% of the time, but at the cost of gathering up to 15 times less data samples on cloudy days when solar power is limited. To further counter power limitations while adding visual data to supplement the field sensors, FarmBeats has designed an optimized drone flight algorithm to use wind to steer them and cover 30% more area before needing to be recharged. In addition to collecting visual and sensor data for the machine learning program to determine the best uses of water, fertilizer, and pesticides, FarmBeats is targeting in the middle term to see how this functionality can fit into other parts of the farming industry, such as livestock monitoring and food refrigeration [2,6].
Recommendations for the short and medium term:
In the short term, FarmBeats should focus on expanding their existing capabilities to other geographies with different soil conditions and weather to ensure that their machine learning algorithm is able to learn from a wide breadth of environments. Rather than expending resources on how to apply this nascent product to other applications, they should emphasize scaling globally and cutting equipment costs below their current level of $220 so that FarmBeats can be implemented more rapidly in the developing world [2]. FarmBeats should also analyze their data acquisition frequency, and if it is sufficient to collect 15 times less data on cloudy days. If not, it may be worth investing further in the implementation of battery technology so that they can achieve a higher data acquisition rate to best guide the farmers on how to improve their crop yield.
Going forward, two challenging issues come to mind:
- After proving their ability to increase yield, what is the best way for Microsoft to scale and implement FarmBeats and “precision agriculture” around the world?
- How can FarmBeats further help reduce the costs to the farmer?
Word Count: 798
Sources:
[1] “Food Production Must Double By 2050 To Meet Demand From World’S Growing Population, Innovative Strategies Needed To Combat Hunger, Experts Tell Second Committee | Meetings Coverage And Press Releases”. 2009. Un.Org. https://www.un.org/press/en/2009/gaef3242.doc.htm.
[2] “Farmbeats: An Iot Platform For Data-Driven Agriculture”. 2017. Usenix.Org. https://www.usenix.org/system/files/conference/nsdi17/nsdi17-vasisht.pdf.
[3] “Agriculture, Forestry, And Fishing, Value Added (% Of GDP) | Data”. 2017. Data.Worldbank.Org. https://data.worldbank.org/indicator/NV.AGR.TOTL.ZS.
[4] “World Population Projected To Reach 9.8 Billion In 2050, And 11.2 Billion In 2100 | UN DESA | United Nations Department Of Economic And Social Affairs”. 2017. UN DESA | United Nations Department Of Economic And Social Affairs. https://www.un.org/development/desa/en/news/population/world-population-prospects-2017.html.
[5] “Cereal Yield (Kg Per Hectare) | Data”. 2018. Data.Worldbank.Org. Accessed November 12. https://data.worldbank.org/indicator/AG.YLD.CREL.KG.
[6] “TV Dinners”. 2016. The Economist. https://www.economist.com/science-and-technology/2016/09/17/tv-dinners.
[7] “Farmbeats Tracks Soil, Moisture Data 24/7”. 2016. Youtube. https://youtu.be/pDgjOHY7sMI.
[8] and Cover Image: “Farmbeats: AI & Iot For Agriculture – Microsoft Research”. 2018. Microsoft Research. Accessed November 12. https://www.microsoft.com/en-us/research/project/farmbeats-iot-agriculture/.
Very interesting read and very socially impactful application of machine learning algorithms. I agree that for this precision agriculture technology to make any meaningful impact in the world it needs to reach developing nations at affordable prices for farmers. Having said that, I will have to disagree with the author in that Microsoft shouldn’t focus their resources on applying these machine learning capabilities into other areas to make an impact in the world’s food problem. The main issues with food around are not only the resources it takes to produce it (which the author very aptly addresses in his publication) but how to make it physically accesible to more people [1]. If food distribution around the world doesn’t improve and yields increase then either people who had access to food before are going to consume more of it or supply will outpace demand leading to spoilage and investments in precision technology will render useless. In short, I see the value in increasing yields with less resources for geographies with great access to food but for this precision technology to make a great impact it need to be paired with improved infrastructure and better supply chain management in developing nations. Therefore, my open question is: can Microsoft apply this machine learning capabilities to help companies predict future supply and demand in areas of the world that have inadequate access to food?
[1] Rijpkema, Marieke. 2018. “How To Solve The Food Problem In The World? – EBF Groningen”. EBF Groningen. https://www.ebfgroningen.nl/journal/how-to-solve-the-food-problem-in-the-world/.
This article is a great analysis of how FarmBeats is leveraging technology in their approach to precision agriculture. I think that research in this area is particularly pressing as we consider the potential destruction and removal of arable lands through climate changes in the future. However, I would echo the concern that Reed Hastings has raised on whether yields are the most pressing issue in this space. I find it an interesting thought experiment to consider the agricultural revolution of the 18th & 19th centuries as a large-scale experiment in crop yield improvements. Fast forward from that period of technological and cultural advancement to today and we have the situation of a world population approaching 8 billion people with massive disparities in access to food. As society explores avenues to activate further increases in crop yields, we also have to ask ourselves how we ensure the next increase does not result in the same disparity. Additionally, we need to examine closely the ways in which increases in crop yields lead population growth (i.e. does a new influx of consumable energy accelerate population growth such that yields are always chasing?) As a company with the reach and resources that Microsoft has, I would urge them to tackle the distribution and waste problems that currently exist within the agriculture industry, while still developing such precision agricultural technologies that will doubtless be useful in a future with a very different climate.