MetLife: A Case Study in Customer Segmentation
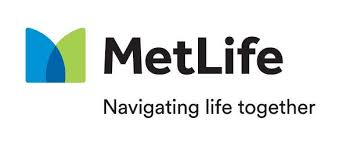
In 2015, MetLife began a year-long brand discovery process that centered around using data and machine learning to develop a more refined view of their customer segments and enable a more nuanced go to market strategy. By better understanding their customers' needs, attitudes, and behaviors, MetLife hoped to gain a competitive advantage in targeting and better serving an increasingly demanding set of customers.
In 2015, MetLife began a year-long brand discovery process that resulted in what they would later call “the most significant change to their brand in over 30 years”.[i] At the core of this strategic refresh, was a fundamentally data driven approach, enabled by advances in machine learning, that revealed to MetLife that the insurance landscape around them was changing: Technological innovations such as the proliferation of internet connections and increased penetration of mobile devices changed the way business was done.[ii] Disruptive newcomers, such as Lemonade, were redefining the market place with their simplified approaches to underwriting. And despite that, customer and shareholder expectations were higher than ever.[iii] In the months that followed, MetLife interviewed and surveyed more than 50,000 customers and with the help of big data clustering techniques used the information to better understand and segment their customers and subsequently redesign their go to market approach.[iii] As an employee of Bain and Company, working with the MetLife team, I had the privilege to see the beginnings of the transformation firsthand.
Rethinking customer segmentation
Traditionally, insurance organizations tried to glean directional insights about their customers’ needs, attitudes, and behaviors through demographics.[iv] In the case of retail customers, age tended to be an important demographic that proxied attainment of certain life stages and thus the sophistication of the individual customer. In the case of corporate customers, the number of employees tended to be an important demographic that proxied sophistication of the organization. Armed with these types of rudimentary insights, insurers would use their best judgement in deciding the bundle of products to offer customers. However, using only demographics, insurers had at best only a rough outline of who their customers were let alone what they wanted or how to target them.
To better understand their customers, MetLife strove to “move from basic demographics and life stages to a view based on mindsets and attitudes.”[v] They collected data on their customers through in-depth surveys designed to extract a combination of demographic, firmographic, attitudinal, and need-type information. Using advanced segmentation tools, survey respondents were clustered into distinct groups based on their individual survey responses resulting in, for the first time in the company’s history, a refined picture of who their customers were. These groups (or segments) provided a new way to think about allocating resources against the pursuit of the “right” customers. Publicly available results of one such clustering (dates back to 2013 corresponding to some earlier work with segmentation), and the strategic targeting implications, are shown in the images below.[vi]
The path forward
As part of their brand refresh, MetLife committed to a data-driven approach “focused on identifying the right customers and creating truly differentiated customer value propositions.”[iii] They committed to an $800 million net annual savings target which they expect to be at full run rate by 2020.[iii] MetLife management stated that realizing the savings would require an estimated $1 billion in investments, a significant portion of which was in technology aimed at getting better data to fuel their increasingly robust data analytics capabilities.[iii]
Further, a core aspect of the customer segmentation work that MetLife engaged in was predicated on the idea that ideal customer segments needed to be “strategic and tactical in nature.”[vii] As part of the of the customer segmentation work, members of the sales force were made aware of the customer segments and given tools to help them effectively engage with target customers.
MetLife took its segmentation practices one step further and began educating its corporate customers, encouraging them to think about their employees through a combination of demographic and psychographic data.[v] MetLife’s business offerings now include “helping HR leaders select their benefits and adjust current programs to suit their diverse employees.”[v]
In many ways, MetLife’s data-driven strategic refresh was significant moment for the company and the broader insurance industry. It applied machine learning towards sales generation when most traditional insurance companies were focused on applying machine learning solely from a risk and improved underwriting perspective.
Going forward, MetLife should continue to embed machine learning deeper within their organization. A 2017 McKinsey article outlined four broad areas where machine learning could create value for an organization: projecting (forecasting), producing (operations), promoting (sales and marketing) and providing (enhanced user experiences).[viii]
MetLife’s efforts in this strategic refresh focused on promoting. Going forward, management should be cognizant not to neglect other areas in which machine learning can add value to the organization.
Ultimately, are sequential improvements in the way MetLife uses machine learning enough to give them a competitive advantage over disruptive newcomers, or is some form of transformational improvement necessary for them to remain relevant?
(768 words)
[i] Stout, Craig. 2016. “The Power Of A Customer Centered Approach – The Metlife Rebrand”. Brand And Marketing Consultancy | Prophet. https://www.prophet.com/2016/10/power-customer-centered-approach-metlife-rebrand/.
[ii] OECD (2017), Technology and innovation in the insurance sector, accessed November 2018
[iii] Metlife inc corporate investor day – final. (2016, Nov 10). Fair Disclosure Wire Retrieved from http://search.proquest.com.ezp-prod1.hul.harvard.edu/docview/1842918111?accountid=11311
[iv] Carr, Mark, and Amy Modini. 2012. “A New Approach To Segmentation For The Changing Insurance Industry”. Cmbinfo.Com. https://www.cmbinfo.com/cmb-cms/wp-content/uploads/2012/03/HealthDoc_FINAL.pdf.
[v] “Building Stronger Engagement Through Employee Segmentation | Workforce”. 2018. Metlife.Com. https://www.metlife.com/workforce/stronger-engagement-segmentation/.
[vi] Mehra, Sanjay, and Leah van Zelm. 2013. “Segmentation. Customer Strategy Done Right – PDF”. Docplayer.Net. https://docplayer.net/13983641-Segmentation-customer-strategy-done-right.html.
[vii] Barlyn, Suzanne. 2017. “Metlife To Invest $1 Billion In Tech To Reach Cost-Savings Goals”. U.S.. https://www.reuters.com/article/us-metlife-investment-technology-idUSKBN17T2R6.
[viii] Bughin, Jacques, Eric Hazan, James Manyika, and Jonathan Woetzel. 2017. “Artificial Intelligence The Next Digital Frontier”. Mckinsey Global Institute, 5.