Major League Baseball and Machine Learning: Can the San Francisco Giants Compete in the Analytics Arms Race?
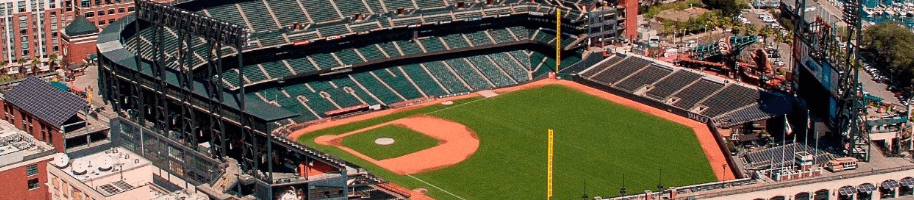
Can a storied franchise recreate itself amid the predictive analytics wave in sports?
Teams are collecting troves of data to gain an on-field edge
There is an incredible wealth of available data in Major League Baseball (MLB). With the advent of Statcast[1], a tracking technology that uses radar and video systems, teams are using artificial intelligence to analyze a growing set of player data[2] to identify patterns that can help coaches and players adjust in-game strategy. A popular use case is defensive shifts, where teams are using deep learning applications on large sets of data to adjust their defensive positioning[3]. Unlocking insights from this data requires the right organizational talent, which has presented a challenge for some teams, including the San Francisco Giants.
Just how far behind are the Giants?
The Giants are four years removed from winning three World Series titles from 2010 to 2014. However, their run of success ended abruptly, and the team has earned a reputation for eschewing cutting-edge analytics in favor of traditional, human-based scouting insights. Since the 2016 All-Star break, the Giants’.422 winning percentage is fourth worst in baseball. Their willingness to spend on expensive players is not to blame, as the Giants’ 2018 season payroll amounted to $199 million, third-most in MLB[4]. With the second oldest roster in MLB by average age (clearly ignoring the amount of evidence suggesting player performance declines precipitously after a certain age[5]), the Giants are a poorly constructed and expensive team with no sign of competing soon.
Using the number of R&D staff employed by each team as a benchmark, one can understand how lagging the Giants are in investing in analytics. As of 2018, the Giants’ R&D staff totaled six employees, which is far fewer than the amount employed by the most forward-thinking teams (New York Yankees with 20, Los Angeles Dodgers 20, and Houston Astros 15, respectively)[6]. Frustrated with the team’s recent performance and consistent overpayment of players past their primes, Giants ownership fired their top two baseball operations executives, both considered Luddites compared to the more data-driven executives leading successful franchises.
What are the Giants doing to close the gap?
Last week, Giants’ CEO Larry Baer (HBS ‘85) hired Farhan Zaidi as president of baseball operations. Zaidi, an MIT graduate with a doctorate from UC Berkeley, is known for his analytical savvy and considered among the preeminent talent evaluators in baseball. Zaidi was most recently general manager for the Los Angeles Dodgers, where he transformed the organization to incorporate more data in scouting, roster construction, and in-game decision making[7]. Zaidi has a track record of implementing AI-driven analytics capabilities to identify overlooked talent and make sound player contract decisions. Put simply, he consistently finds undervalued assets and refuses to overpay for value.
Though hiring Zaidi is an important first step for San Francisco, there is more work to be done. In the next year, the Giants must expand its R&D staff to further develop the analytical capabilities required to compete in today’s game. Long-term, San Francisco should explore strategic partnerships with National Basketball Association teams to source new and innovative ways to incorporate predictive analytics in sports. In addition, the Giants should entertain acquiring a sports analytics startup (a growing industry) to stay ahead of competing teams.
What’s next for machine learning and baseball?
Skeptics contend that analytics is detracting from baseball’s rich tradition and reducing the game to mere algorithms. I disagree. In baseball, as in most industries, more information is valuable. How teams use that information before, during, and after games determines competitive advantages. However, is there a limit to how much predictive analytics can be used in baseball? Will artificial intelligence render traditional coaching and scouting staff obsolete?
(744 words)
[1] Major League Baseball, “Statcast,” http://m.mlb.com/glossary/statcast
[2] Edd Gent, “How AI is helping sports teams scout star players,” NBC News, June 13, 2018 [https://www.nbcnews.com/mach/science/how-ai-helping-sports-teams-scout-star-players-ncna882516]
[3] Arjun Dutt, “How Is Deep Learning Changing The World Of Sports?” Forbes, August 16, 2017 [https://www.forbes.com/sites/quora/2017/08/16/how-is-deep-learning-changing-the-world-of-sports/#5a5c74184a09]
[4] Cot’s Baseball Contracts, “2018 MLB Tracker,” https://legacy.baseballprospectus.com/compensation/cots/
[5] Ray C. Fair, “Estimated Age Effects in Baseball,” Journal of Quantitative Analysis in Sports, 2008, Vol. 4: Iss. 1, Article 1.
[6] Marc Carig and Eno Sarris, “‘Cold Hard Cash’: How Brian Cashman played the long game and used analytics to transform the Yankees’ culture,” The Athletic, October 3, 2018 [https://theathletic.com/560514/2018/10/03/how-brian-cashman-deftly-played-the-long-game-and-used-analytics-to-transform-the-culture-of-the-yankees/]
[7] Andy McCullough, “How Dodgers GM Farhan Zaidi became one of the most coveted minds in baseball,” Los Angeles Times, March 30, 2017 [http://www.latimes.com/sports/dodgers/la-sp-dodgers-farhan-zaidi-20170330-htmlstory.html]
Interesting read. At what point does this scouting extend to minor leagues, college, and high school? At what point do you need to people to go to games and scout talent to feed into the machine, vs. deploying technology as a sensor to crowd source this?
I’ll be interested to see how their models and metrics shift if baseball continues to impose time limits between pitches or shortens the game in an effort to keep more fans engaged.