Machine learning ruined baseball. Machine learning will save baseball.
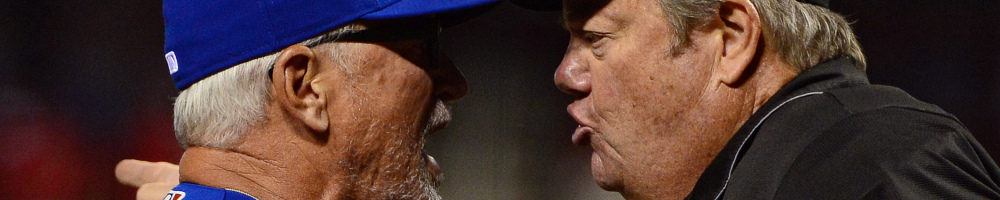
Baseball has fundamentally changed over the past 40 years, largely thanks to data and machine learning. Some say it's made baseball less popular as a result. We will examine data's impact on baseball and how it might help the sport's popularity going forward.
Baseball: Getting to Today
Baseball has a long and storied past in America. Over the past 40 years, machine learning and data have fundamentally changed the game. As teams used data to perfect the “product,” the game changed in fundamental ways. Machine learning ruined baseball. Now, it has an opportunity to save it. This paper will analyze how machine learning impacts baseball’s product development: developing players and winning games.
Professional baseball began in the mid 1850s, and the National League – a prominent baseball fixture even today – was born in 1876. For over a century and a half, fans have been captivated by the sport. Baseball has a propensity for wild, crazy, and unpredictable turns. As baseball legend Yogi Berra says, “it ain’t over ’til it’s over.”
The future of baseball shifted in 1980, when “sabermetrics” began to take hold. It is “the search for objective knowledge about baseball.” For almost 100 years, managers led based on intuition and small amounts of data. Sabermetrics promised an edge: Player A might be the best, but 80% of his ground balls are towards first base. Why not change your defense to adjust? Player B might be a top pitcher, but towards the end of the game, why not bring in three different pitchers to face three different batters, each tailored for the situation. The game began to shift, shown below.
Baseball Today
So what? As the data got better, more teams used it. Coaches made more and more changes to players throughout the game. Games took incrementally longer, and star hitters saw their ability to produce runs diminished, as shown below. Fans get to spend more time at a ballpark to watch less action – thrilling!
The numbers support this. In 2016, the Chicago Cubs won the best championship game in history. The Cubs broke a 108-year draught, the longest in any American sport. It was the most-watched in 25 years, with 40+ million viewers, 70% higher than the prior year. How does that compare? On a down year, the NFL brings in 100+ million Super Bowl viewers.
Data ruined baseball. Now, we will discuss how data can save baseball.
What’s Next for Baseball?
First, there are baseball’s current plans. The trend towards longer games and more data-based decisions is not going anywhere. Baseball will continue to leverage machine learning to produce a better “product” – a winning team. Of course, teams cannot simply ignore data simply to make games faster. The algorithms will continue to get smarter with more data, and new sensors and data collection methods will allow more analysis.
Longer-term, baseball plans to use data to analyze more types of information. Companies are investing in technology that would allow algorithms to monitor players’ physical health and even mental health. Imagine knowing a player might not perform because of his or her brain activity or heart rate, not just the opposing pitcher for the day.
As the algorithms get better, games will get longer. Baseball will implement more and more controls to shorten games: pitch clocks, automated strike zones, and perhaps fewer pitching changes. Still, these will remain reactive and not buck the trends.
What else can MLB do? There are several opportunities. First, if data is such a big opportunity for coaches, why not for fans? We interact with the output of machine learning in a boring way: basic stats are broadcast. Why not make this data a product for customers, too? Imagine a young fan seeing all the pitching data on her iPhone at the ballpark – she votes to keep the starting pitcher in place and sees what other fans selected. The coach decides to keep the pitcher in, and he gives up a homerun. The fan felt the impact and interacted with the data. This is essentially an HBS case every single play. Data does not need to be behind the scenes.
Second, baseball should use data to make better games and schedules. Our world is dramatically different than it was in the early 1900s, yet teams still play the same in-division games for most of the year. Data can better inform not just how teams play, but whom teams play. Baseball should loosen the structure between leagues and allow data to inform what games would draw the most fans for at least some part of the season.
There is a fundamental question raised here: what is baseball’s product? Is it entertainment? It is winning? Is it the entertainment of winning? That is, if a game is more fun but your team loses, do you go home happier? Data does choose a side – it goes for winning. How do we strike the balance? And, how involved do we keep human decision-makers along the way? (783 words)
SOURCES:
- Baseball Game Length Visual Analysis
- Roth, David. Baseball no longer a supergiant but it is still the most American of sports. The Guardian. Link
- Kurkjian, Tim. Putting the future in focus: The blueprint for baseball in 20 years. ESPN. Link.
- Keri, Jonah and Paine, Neil. How Bullpens Took Over Modern Baseball. FiveThirtyEight. Link
- Society for American Baseball Research
Interesting post – which highlights the necessity of tying machine learning to a company’s business model. Baseball is unique in that the MLB has 30 clubs all focused on their own P&L – which is often most easily influenced by winning. Machine learning / sabermetrics may help any individual team increase its odds of winning, and thus improve its P&L, but at the expense of the overall enjoyment of games and the league as a whole. As you addressed at the end of your post, how can the MLB (and individual teams) leverage machine learning to better appeal to the casual and serious baseball fan? And how can machine learning and advanced statistics be used to increase the watch-ability and market-ability of baseball? Both the NBA and the NFL have grown massively in popularity over the last decade, a portion of which can be attributed to the leagues’ marketing of its stars. Can the MLB do something similar to increase its popularity?
Billy Beane was a pioneer in this space but even though he found divisional success, he never won a World Series (as a GM). One thing that makes sports so great is that imperfect human interaction is involved in every aspect of the game. No matter how much data we get through machine learning, there will always be a human element making it so anything can happen.
Criticizing Billy Beane for not winning a World Series is not necessarily fair as other teams quickly began copying his approach. In professional sports, just like capital markets, when you find a data driven edge it will quickly be competed away. The goal of a manager is to win but the goal of the MLB is to create entertainment. I am all for managers continuing to improve the chess match that is baseball, but lets make sure to do so without hurting the consumer. That is where the MLB must step in with reactive measures. As long as those reactive approaches are timely, I do not see the need to buck the trend and stifle competitive innovation.
We might also want to consider the human element here – are we simply approaching the limit of what’s physically possible given today’s rules? Data might help us here too. How many guys are throwing 100+ mph today? It’s higher than it was even 10 years ago. Perhaps we need a few more feet than the 60′ 6″ to home to get the same performance out of the boys at the plate.
Thanks for the thought-provoking post. As a lifelong baseball player and fan, two of the things I love about the game is the human element and tradition, so I worry about baseball introducing elements like robo umpires or data informing which teams play each other. That said, I see the slowing pace of the game as a threat to its popularity and I am intrigued by the idea of using machine learning to improve the game. For instance, could baseball use data and machine learning to solve for the optimal fan experience — i.e. determine what balance of length of game, runs per game, pitching changes per game, etc. on average leads to the highest fan enjoyment. This could help MLB determine what initiatives to prioritize as it seeks to make the sport as popular as it can be, without surrendering the human element or tradition.
Your suggestion to allow data to inform scheduling is an interesting one, and I like the idea, but I definitely worry about this being taken to the extreme. If we seek to always pit the most popular teams against each other, the Yankees and Red Sox could play a majority of their games against each other and neither would ever play the Padres.
Lastly, as a baseball player and fan, I have to say that @Scuba Steve’s suggestion to move the mound further from the plate is a ludicrous one. Baseball will never and should never change the fundamental dimensions of the infield. That’s one element of the game machine learning should never be allowed to alter.
Interesting reading, I like it a lot! Thank you for sharing.
I agree that data eliminates room for players to play their own baseball making them just one piece, and on the other hand, strategy/tactics weighs in more. But at the same time, there are star players even when players are analyzed, and when players and teams go beyond data, next level of excitement is there!
On your final point on the fundamental goal – I believe the decision of balancing needs to be made by humans. It would be amazing (and also a bit scary) if machines learnt the best mix of winning, game duration, highlight scenes, etc.
Hmm Billy, interesting opinions here. I think baseball has a number of challenges which are addressed in the other (better) baseball article on this website but you certainly raise some good points about the impact of data on the sport. I do think that despite the impact on game duration, the use of data across the sport has made it better and more competitive. We are seeing pretty good parity in the sport with small market teams competing well with big markets (even if the big markets still typically win it all, see: Red Sox).
While I can definitely understand the use of data in manager decision-making, I think we’d be taking things too far if we used data to affect scheduling since there is an important element of tradition and rivalries which are reflected in the current division setup. Similarly, the second we allow fans to impact manager decision making we start to lose the legitimacy of the sport in terms of the competition element. The coaches and players should decide and determine what happens on the field, not the fans. Now, where I do see potential on this type of voting you brought up is in sports betting which is another story altogether.
Baseball has a big challenge ahead of it in that its US audience is considerably older and typically whiter than other sports. With changing demographics in the US, it’s interesting to think about what impact the use of data will have on the popularity of the sport. Will it bring in younger and more diverse fans or just alienate them even further?
Go check out the better baseball article here to see how data is used in baseball ticket pricing!
https://d3.harvard.edu/platform-rctom/submission/get-em-while-theyre-hot-dynamic-ticket-pricing-in-major-league-baseball/?section=7756&sort=rand