Machine Learning in the Fragmented Construction Industry
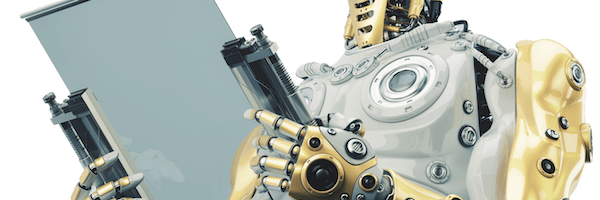
Machine Learning: Upward Trajectory for the Construction Industry
The construction industry represents 4% of the US GDP[1], and is one of the largest sectors of the world economy. However, it suffers from a slow rate of productivity growth at 1% per year in comparison to manufacturing that has a growth rate of 3.6% per year.[2] The construction industry is also the lowest on the digitization scale[3], where technology and innovation spend is amongst the lowest of industries.
The construction industry suffers from cost overruns and schedule delays that have become the norm. Mega projects and multi-billion-dollar capital programs with tight schedules are on the rise and companies are looking to technology to increase the efficiency of their building and information management processes.
Machine learning (ML) applications have the potential to increase the efficiency of the construction process. The industry is one of the most fragmented in the world,[4] which makes access to large data sets the biggest challenge for ML implementation. A large amount of structured data is required to train supervised learning algorithms. Organizations with access to large data sets are well positioned to take advantage of ML algorithms and advance the industry.
Skanska is one of the largest construction and development companies in the world, operating in 11 countries, with 40,000 employees and a revenue of $18.8 billion in 2017 [5]. The organization has access to a vast amount of project data that could be used to train ML algorithms and automate future tasks.

Skanska started implementing ML through the use of cloud-based platforms, such as Smartvid.io[6]. On any given construction site, the field team collects a vast amount of data through photographs and videos. Historically, collecting, storing, and labeling all this photographic data has been a time-consuming task and construction firms often outsourced the task to consultants such as Multi-vista[7]. The Smartvid.io platform structures the data collection of photos and videos from various sources including mobile, tablets and UAVs, provides unlimited storage and continuously learns from the user’s labeling. That allows a supervised learning algorithm to learn from expert labeling and support the construction site safety team by automatically identifying safety risks from the photographs. For example, it is able to identify if a worker is not wearing a hard hat or not using the required safety gear.
Current applications in the industry are only scratching the surface of the potential of ML. A large portion of the industry still communicates through hard copy documents and spreadsheets. Multi-billion-dollar infrastructure projects teams are still more comfortable with email and spreadsheets rather than more structured project management systems. There is a lot of opportunity for improvement in construction and it really starts with data collection. Construction firms including Skanska have been mostly focusing on structuring the majority of the unstructured data to be able to implement ML. With technologies such as cloud-based project management systems and building information management software, organizations today have much more data to analyze and learn from.
Future applications of ML include:
- Automatically develop and check project estimates – construction cost estimating relies on the construction firm databases and staff experience. Estimators perform quantity take-offs, search company databases for similar projects and reach out to their trade contractors to identify the latest market price. The process is repetitive, time consuming and prone to human error. ML can support estimators in comparing cost structures and breakdowns across the firm’s vast portfolio to increase the speed and accuracy of their estimating process.

- Automatically develop project schedules – planners spend a significant portion of their time visualizing the sequence of construction activities and communicating their plan to the project team through Gantt charts and 4D models (time phased 3D models demonstrating construction sequence). By combining 4D models, video and photo capture of actual construction sequence, ML can automatically generate realistic schedules and generate 4D models by learning from a database of past projects.
Opportunities for ML also include real-time performance measurement, supply chain management, generative design, and automating regulatory compliance checking through natural language processing applications.
In a fragmented industry plagued by adversarial contractual structures, there is little incentive for different companies to share data and advance ML. Technology companies are the emerging winners in the race. They are the impartial party collecting ‘anonymous’ data from all firms and training their ML algorithms. How can companies share data, remain competitive and capture value, without giving up their market share to technology firms?
(800 words)
[1] U.S. Department Of Commerce. Bureau Of Economic Analysis. Value Added By Industry As A Percentage Of Gross Domestic Product. 2018. https://apps.bea.gov/iTable/iTable.cfm?ReqID=51&step=1
[2] McKinsey Global Institute. 2017. “Reinventing Construction: A Route To Higher Productivity” Https://Www.Mckinsey.Com/~/Media/Mckinsey/Industries/Capital%20projects%20and%20infrastructure/Our%20insights/Reinventing%20construction%20through%20a%20productivity%20revolution/Mgi-Reinventing-Construction-A-Route-To-Higher-Productivity-Full-Report.Ashx
[3] McKinsey & Company. 2016. Capital Projects & Infrastructure. “Imagining Construction’s Digital Future”. https://www.mckinsey.com/industries/capital-projects-and-infrastructure/our-insights/imagining-constructions-digital-future
[4] World Economic Forum. 2016. “Shaping the Future of Construction”. http://www3.weforum.org/docs/WEF_Shaping_the_Future_of_Construction_full_report__.pdf
[5] Skanska. 2018. https://www.skanska.com/
[6] Caulfield, John. 2018. “Tech Report 5.0: AI Arrives”. Building Design and Construction. https://www.bdcnetwork.com/tech-report-50-ai-arrives
[7] Multi-vista. 2018. https://www.multivista.com/construction-photo-documentation/
Great article! Thanks for sharing.
It is surprising how broad is the potential opportunity of machine learning within the construction industry. In addition, I believe that the application of each feature can be replicated not only in other industries but also across geographies. For example, Smartvid could be applied in many other industries, such as mining or industrial goods manufacturing, and thus improve the safety conditions of its workplaces. This would result in a sharply decrease in the amount of fatal accidents that those industries currently experience, especially in emerging markets where regulation is far from optimal.
This is very interesting! I wonder – it seems like gathering data in this context (videos/photos) is very labor-intensive, but in order to continuously improve the system’s ability to detect, say a worker not wearing a hard-hat, would Skanska need to perpetually film/photograph the work site? I imagine data would need to be continuously gathered even after the model is “perfected” because there may be shifts in the construction industry (e.g. safety regulation changes). Is there an effort or option that improves upon the ease of data collection?
Your question around aligning incentives from various parties to share data reminds me of a similar challenge faced by the airline industry. In that space, data is fragmented and distributed across different players with little incentive to (and at times legal requirements not to) share data with each other. On the ground, airports hold a multitude of information such as local weather conditions, passenger location (i.e. at the security line), and other logistical information that would be incredibly useful for airlines. Airlines hold passenger data, flight information, among other things. And–if the planes are leased–owners usually hold maintenance, performance, and design information among other things. Combining data across all these players could lead to significant efficiencies, but fostering collaboration is challenging. GE’s Digital Aviation business is working with customers to figure out ways to address this issue, but I believe what it comes down to is finding win-win specific use case scenarios–starting small–building trust for that one use case, and then expanding from there. For example, you could start by having airports share traveler locations in the airport (i.e. when they check in at a restaurant or at the security checkpoint) to help airlines find passengers and ensure they don’t miss their flights. The airline wins by reducing costs of having to rebook passengers on new flights, and the airports win by driving higher customer satisfaction. If customers find that they’re missing less connections in one airport vs. another, for example, they might be more likely to fly through that airport. So in sum… it’s all about finding mutually beneficial use cases in the construction industry where both parties can see the value, and starting small… then growing from there, one use case at a time.
I think one of the very interesting things you bring up is that the value of ML to the construction industry is obvious and immediate, the ability and manner of collecting both training data and real time data to influence the process is the challenge. I think the front end of data collection is key in this instance, as schedules for laborers are heavily compressed, severely limiting the amount of time (or effort) available to enter data into the system. Outsourcing data entry to a 3rd party observer is expensive and less reliable than the person doing the actual work. I like the proposition you raised of leveraging computer vision to input data automatically, and the system of “bodycams” employed by police officers may be an option. The massive amounts of video would require huge computational power, however it may also be able to identify potential construction problems and defects which could be a great cost saver. Interesting article!
“How can companies share data, remain competitive and capture value, without giving up their market share to technology firms?”
To a large extent, I think this problem is unavoidable. Consumers and other businesses suffer from giving up their data to technology firms. It only becomes a problem when technology firms (1) suffer from breaches or (2) use that data to enter brand new businesses, ie Amazon. Given capital requirements and overall complexity in the construction industry, tech companies are probably further out from entering the space, giving construction businesses more space to adopt ml techniques and remain innovative. The technical challenges still remain, and it’s incumbent on major players to hire the right technical talent and start to instill the cultural change they need to remain competitive.
I found this article extremely interesting! As it is focused on one of the largest industries in the world, with the least amount of digitization so huge potential. Was also cool to see new applications of Machine Learning, which I did not think about previously or was exposed to as using security cameras to automate an automatic safety process in the company or building 4D project management schedules. While this sector is ready for a transformation my main concern is how do you implement these massive behavioural and adoption changes required to adapt new and complex software while most of the industry still works in a very old fashioned way and will probably be reluctant to change:
“A large portion of the industry still communicates through hard copy documents and spreadsheets. Multi-billion-dollar infrastructure projects teams are still more comfortable with email and spreadsheets rather than more structured project management systems.“
This is a very refreshing take on an industry that’s otherwise often regarded as archaic and slow-moving. However, my sense from reading the article is that there’s so much work to be done to transform the whole industry to be able to take advantage of new technologies and actually increase productivity. Too often, I have seen companies coming in trying to capture value from the lack of adoption and high fragmentation in the construction industry (think about the number of project management systems software promising the latest AI, cloud applications). I think the industry can benefit from bold innovators that truly believe in the deeply needed change and technology adoption, innovators that deeply believe in the power of creating lasting impact instead of merely extracting value, and consequently innovators that do not hesitate to open up, share data, and help create trust and value in this industry.
Thank you for sharing!
One key aspect where ML can revolutionize the industry is on inventory management, both from the perspective of waste reduction to order budgeting.
First, ML could help manufacturers of construction materials and equipment to understand how construction employees are usin their products on the field. As a result, these companies coud use the lessons learned to design better products that reduce waste on the construction site.
Second, ML could learn from the construction habits of the employees and anticipate future orders of materials, thus vastly reducing the lead time of key materials and reducing potential bottlenecks in the operation.
Great article. I really like the way Skanska is using ML to improve safety. This is the top priority of any job site and an excellent use of the technology. I wonder if it could also be used to optimize the use of building materials: 1) if it could lead to further our testing and understanding of the best construction materials to use in different climates and 2) lead to more efficient use of building materials once they are on the job site. For example, ML could help scientists and construction leaders find the correlation between a building’s useful-life and its underlying design and material. This could lead to buildings better suited for different environments. ML could also be used to warn construction managers of situations that tend to lead to cost over-runs in materials. I agree, there are plenty of future applications for ML in construction!
I am curious how the technology companies are gaining access to data in the construction industry. I wonder if there might ever emerge a third party within the construction industry, that can offer ML-enabled services to construction companies and replace tasks they already undertake. My hypothesis is it might be easier within the fragmented construction industry for a third party to be trusted with a company’s data over a competitor firm. However, this third party would have to find a way to deliver immediate value while it connects with many firms and amasses data, in order to eventually offer meaningful, ML-informed construction schedules or project estimates. Another alternative scenario would be for a new, ML-enabled construction company to emerge from the ground-up. If a new company came on the scene with this vision and the necessary talent, they could, over a long time horizon, find ways to make their business processes more efficient than a business not bothering to incorporate ML into their business processes.
Based on inherent barriers in this fragmented industry, it might be a while before we see ML have an impact in construction!
From the use of the machine learning to improve safety to using artificial intelligence to schedule and plan resources, there are so many ways the construction industry can benefit from the recent development in digitization. Throughout the history, construction has been highly labor intensive despite the industrialization. In my opinion, the biggest innovation in construction would be mechanization of cranes. Invention of steel concrete comes second. The industry values the successful track record more than anything else and the uptake of the new technologies has been painfully slow. Based on my personal experience, the accumulation of data and lessons learned are not systematic and a lot of knowledge only resides in people, forcing repetition of same/similar mistakes in every 10-15 years. In order to change this entrenched culture, the benefit of adopting these technologies should be very clear and the solutions must be made easy to adopt. I sincerely hope that along with development in robotics, AI will automate much of the engineering, planing, and the actual construction phase.
I really enjoyed reading this excellent essay.
It clearly explains the current application of machine learning in the construction field and outlines the vast potential for additional applications. For a traditional sector that suffers from low productivity growth and frequent cost overrun, machine learning could provide a new hope for elevated services in the future.