Machine Learning in Radiology: Threat or Opportunity?
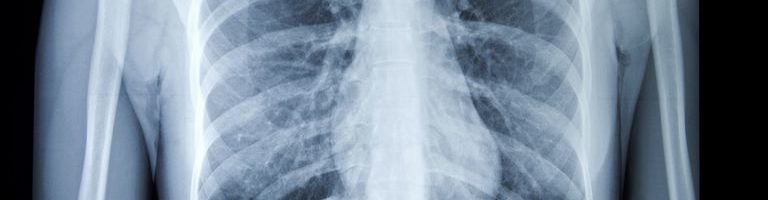
Is the use of machine learning in radiology hype or reality? That is the question that the American College of Radiology must answer.
Prompted by recent developments in machine learning and artificial intelligence, there has been a growing national debate about automation and the future of work.[1] Few types of work have received more attention in this debate than radiology. Geoffrey Hinton, one of the leading researchers in machine learning, went so far as to say that machine learning makes “it quite obvious that we should stop training radiologists.”[2] On the other side of the debate, Seth Berkowitz, a notable radiologist at Beth Israel Medical Center, argued unequivocally that “radiologists will not be replaced by machines.”[3] So what is hype and what is reality in this debate? This is the question that the American College of Radiology, the professional medical society representing radiologists in the United States, must answer. How should radiologists respond to the threat posed by machine learning?
Radiology is a frontier in the application of machine learning. This is true for several reasons. First, radiology has large, categorized datasets, making it ideal for supervised learning.[4] Second, the core task of radiology involves image classification, a demonstrated strength for machine learning.[5] And third, radiology is a massive market; 800 million radiology exams, generating 60 billion images, are conducted annually in the U.S.[6] Two landmark papers recently demonstrated the potential for machine learning algorithms to replace radiologists. In the first paper, researchers at Berkeley matched the ability of radiologists to diagnose Alzheimer Disease using PET scans.[7] In the second paper, researchers at Stanford identified pneumonia in chest x-rays more accurately than a comparison group of radiologists.[8] Results like these have led to expectations that the use of artificial intelligence in radiology is set to grow rapidly; Frost & Sullivan forecasts that the market size for applications of artificial intelligence in radiology will grow over the next five years from $150 million to $1.4 billion [Figure 1].[9]
The American College of Radiology (ACR) has been increasingly focused on this topic; one recent issue of the ACR’s journal was entirely dedicated to the use of machine learning in radiology.[10] The ACR’s response to the threat seems primarily to be to deny that it exists. One paper summarized a recent conference co-hosted by the ACR: “The attendants grappled with the potentially disruptive applications of machine learning to image analysis. Although the prospect of algorithms’ interpreting images automatically initially shakes the core of the radiology profession, the group emerged with tremendous optimism about the future of radiology.”[11] Another paper published by the ACR was even more explicit “Predictions have been made that suggest AI will put radiologists out of business. This issue has been overstated.”[12] Even within the ACR, however, a dissenting minority takes the opposite view: “There is growing anxiety throughout the field that much of the work currently performed by radiologists will be carried out more quickly, accurately, and at lower cost by computers, soon completely replacing man with machine. We believe that talk of the impending triumph of machines in radiology is far more prescient than most radiologists suspect.”[13]
The reality is that neither side of this debate is correct: machine learning is neither an existential threat nor a non-issue in radiology. On the contrary, machine learning is most likely to become a complement to, rather than a substitute for, radiologists. The reason is simple; while machine learning has already proven its ability to match or exceed the performance of radiologists on some tasks, these tasks represent only a small portion of the responsibilities of a radiologist. Algorithms won’t replace the human touch involved in discussing a diagnosis with a patient.[14] And because algorithms are hyper-focused, they won’t be able to provide a holistic and exhaustive diagnosis.[15] This all means that “the only radiologists whose jobs may be threatened are the ones who refuse to work with AI.”[16] The ACR should therefore shifting from denying the importance of machine learning to embracing it; it should actively take steps to encourage the adoption of machine learning in radiology and to improve its efficacy.
Though it is clear that machine learning will significantly impact radiology in the future, several important questions remain. Because machine learning will enable radiologists to read images faster and more effectively, market forces are likely to drive adoption of machine learning applications by radiologists. But how will other stakeholders in the ecosystem respond? How should the FDA regulate an algorithm with limited precedent, particularly one that is dynamic rather than static?[17] And will consumers ever learn to trust an algorithm, even one backed to rigorous studies?[18] The stakes are far too high to let these roadblocks limit adoption. The use of machine learning in radiology will save lives.
(Word Count = 767 Words)
Figure 1: Total Medical Imaging AI Market: Revenue Forecast, Global, 2015-2022 (Frost & Sullivan)
[1] Thompson, Derek, “A World Without Work,” The Atlantic, July/August 2015, https://www.theatlantic.com/magazine/archive/2015/07/world-without-work/395294/, accessed November 2018.
[2] “AI, Radiology and the Future of Work,” The Economist, June 7,018, https://www.economist.com/leaders/2018/06/07/ai-radiology-and-the-future-of-work, accessed November 2018.
[3] Pearson, Dave, “Artificial Intelligence in Radiology: The Game-Changer on Everyone’s Mind,” Radiology Business, October 13, 2017, https://www.radiologybusiness.com/topics/technology-management/artificial-intelligence-radiology-game-changer-everyones-mind, accessed November 2018.
[4] Walach, Elad, “Which Area of Medicine is Most Ripe for AI Disruption?”, Forbes, April 12, 2018, https://www.forbes.com/sites/forbestechcouncil/2018/04/12/which-area-of-medicine-is-most-ripe-for-ai-disruption/#1729e5b92943, accessed November 2018.
[5] Ibid.
[6] Ip, Greg, “How Robots May Make Radiologists’ Jobs Easier, Not Redundant”, The Wall Street Journal, November 22, 2017, https://www.wsj.com/articles/how-robots-may-make-radiologists-jobs-easier-not-redundant-1511368729, accessed November 2018.
[7] Ding, Yiming, et al., “A Deep Learning Model to Predict a Diagnosis of Alzheimer Disease by Using 18F-FDG PET of the Brain,” Radiology, November 6, 2018, https://pubs.rsna.org/doi/10.1148/radiol.2018180958, accessed November 2018.
[8] Rajpurkar, Pranav et al., “MURA: Large Dataset for Abnormality Detection in Musculoskeletal Radiographs,” Medical Image Analysis, June 5, 2018, https://openreview.net/forum?id=r1Q98pjiG, accessed November 2018.
[9] “Growth Opportunities Growth Opportunities in the Global Medical Imaging Artificial Intelligence Market, Forecast to 2022,” October 30, 2018, https://cds-frost-com.prd2.ezproxy-prod.hbs.edu/p/71319/#!/ppt/c?id=MD1C-01-00-00-00&hq=global%20artificial%20intelligence%20cognitive%20computing%20healthcare, accessed November 2018.
[10] “Special JACR Issue Focuses on Data Science and Artificial Intelligence Use in Medical Imaging,” Journal of the American College of Radiology, March 1, 2018, https://www.acr.org/Media-Center/ACR-News-Releases/2018/Special-JACR-Issue-on-Data-Science, accessed November 2018.
[11] Kruskal, Jonathan et al., “Big Data and Machine Learning—Strategies for Driving This Bus: A Summary of the 2016 Intersociety Summer Conference,” Journal of the American College of Radiology, June 2017, https://www.jacr.org/article/S1546-1440(17)30199-0/fulltext, accessed November 2018.
[12] Thrall, James et al., “Artificial Intelligence and Machine Learning in Radiology: Opportunities, Challenges, Pitfalls, and Criteria for Success,” Journal of the American College of Radiology, March 2018, https://www.jacr.org/article/S1546-1440(17)31671-X/fulltext, accessed November 2018.
[13] Kirk, Ray et al., “The Triumph of the Machines,” Journal of the American College of Radiology, March 2018, https://www.jacr.org/article/S1546-1440(17)31175-4/fulltext, accessed November 2018.
[14] Pearson, Dave, “Artificial Intelligence in Radiology: The Game-Changer on Everyone’s Mind,” Radiology Business, October 13, 2017, https://www.radiologybusiness.com/topics/technology-management/artificial-intelligence-radiology-game-changer-everyones-mind, accessed November 2018.
[15] Mukherjee, Siddhartha, “A.I. versus M.D.”, The New Yorker, April 3, 2017, https://www.newyorker.com/magazine/2017/04/03/ai-versus-md, accessed November 2018.
[16] Davenport, Thomas and Keith Dreyer, “AI Will Change Radiology but it Won’t Replace Radiologists,” Harvard Business Review, March 27, 2018, https://hbr.org/2018/03/ai-will-change-radiology-but-it-wont-replace-radiologists, accessed November 2018.
[17] Kohli, Marc et al., “Implementing Machine Learning in Radiology Practice and Research,” American Journal of Roentgenology, April 2017, https://www.ajronline.org/doi/abs/10.2214/AJR.16.17224, accessed November 2018.
[18] “Accenture 2018 Consumer Survey on Digital Health,” Accenture, 2018, https://www.accenture.com/us-en/insight-new-2018-consumer-survey-digital-health, accessed November 2018.
I agree with you saying that AI replacing radiologists is overblown. It’s a bit like saying that autopilot in planes will replace pilots – something that is unlikely to happen anytime soon even though most planes spend the majority of time in autopilot mode. A critical thing to remember is that radiologists are the ones training the algorithms, so in fact, we as a society ought to invest in the development of superior radiologists who could spot existing and new pathologies with superior accuracy so that we can have better algorithms for mainstream use.
As for the regulators, CDS (clinical decision support) is still a point of learning and evolution. CDS is currently bundled under Medical Device classification, but the guidance on whether a piece of medical software is a CDS or not is example/case based and in my opinion does not provide a clear enough criteria. If I’m correct Zebra Medical Vision was the first to have an FDA approved/CE marked radiology CDS that was approved this year under the medical device classification. As of now, new versions/retrained models of CDS do not have any fast track approval and must go through a new review process. Hopefully that at least is bound to change in the future as the technology becomes more mature and regulators have figured out the best way to harness the fact that ML based CDS has the potential to improve over time.
My diagnosis: a great article! Really interesting to hear how this is shaping hospital decision-making processes.
I’d be particularly keen to understand more about the patient response to the level of human involvement. These kinds of innovations are somewhat reliant on reducing the cost of patient treatment – if radiologist involvement stays high based on patient demands, it becomes harder to role out at greater scale. What confidence level does a patient need that they are receiving the correct diagnosis, even when a machine learning approach is measurably better than a human’s judgement?
Really awesome/intimidating article considering the only member of my household currently earning an income has similar concerns about the impact of machine learning on the role of medical practitioners.
I would be interested to examine whether the FDA would actually have authority to regulate an algorithm. Given this is a newer space, would a diagnostic algorithm function in a similar fashion to medical reference apps (e.g. Epocrates)? If so, should the FDA start to shape new policy on the way it monitors “reliable sources.”
November 15, 2018
JP says:
This essay is an interesting take on the future of radiology in a world of Artificial Intelligence. I disagree and think that AI does have the potential to replace or at least drastically reduce the number of jobs available for radiologists in the future. Radiology is not a patient-facing specialty. They work mostly in dark rooms on computers and imaging results are delivered through physicians of other specialties. Therefore, the human aspect of radiology is not as relevant. Radiologists do take into account the clinical situation, but it is easy to build an algorithm that could also take into account the clinical picture of a patient when reading an image. In fact, it might be better to have an “unbiased” read of the image, so that the clinical picture does not bias how the image is read.
Your final question on whether or not patients will learn to trust algorithms is also interesting. I also wonder whether providers will learn to trust algorithms. From my experience in the hospital, almost all physicians look at their patient’s images with their own eyes before looking at the radiologist’s read of the image (at times completely disregarding what the radiologist says). I wonder if physicians will actually trust an algorithm’s read of an image more than they would a radiologist that they know has greater variance in accuracy. It is also interesting to think about whether patients will at the point of care who or what read their image. Will regulation around AI in radiology require physicians to disclose that the results were generated by an algorithm, or will the physicians more likely say that they themselves read the scans?