Machine Learning & Early Cancer Detection: Do We Know Enough to Ask the Right Questions?
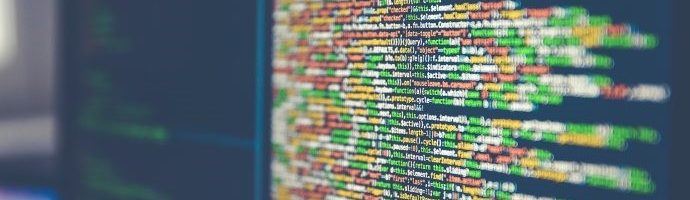
What if you could test for cancer before a tumor even develops?
The National Cancer Institute estimates that over 600,000 people in the United States alone will die from cancer in 2018 [1]. Survival rates increase dramatically when cancer is diagnosed early because the disease often has not spread and more treatment options are available [2]. Everyone wants to solve this problem. Early cancer detection could be the key.
Old Research, New Process Researchers have attempted to identify cancer for decades, initially by searching for the presence of tumors through the use of x-rays, colonoscopies, and mammograms [3] [4]. More recently, research has focused on detecting cancer earlier through minimally-invasive liquid biopsies that look for cancer biomarkers in the blood [5]. Instead of looking for cancer itself, they are looking for indicators that it is developing. Detecting cancer this early is difficult because the amount in the blood is infinitesimal and the differences from sample to sample are minute. How does a human sort through the data to separate significant patterns from the noise? The AI genomics company, Freenome, seeks to solve this problem with the help of machine learning. They leverage the computing power of machine learning to process large data sets and uncover complex patterns that might be missed by a human.
Freenome’s First Clinical Study Freenome recently launched its first clinical study, AI-EMERGE, to create a blood-test to detect colorectal cancer at an early stage [6]. They will collect blood and stool samples from both healthy patients and patients with colorectal cancer. Freenome plans to iteratively improve their algorithms by gathering the data from this study and training the model on it. They chose to focus on a subset of cancer for the first clinical trial to test the validity of their hypothesis. Other research studies in this field, such as Johns Hopkins CancerSEEK, have tested across many different types of cancer including ovarian, lung, and liver [7]. While understanding the difference across cancers is critical, targeting one cancer first and focusing the number of variables the algorithm must consider could help Freenome adapt their approach more effectively. Long-term, Freenome does plan to expand to other cancers and gather more data to fine-tune their algorithm.
Freenome also tests both healthy and cancerous samples. Training on cancerous samples, the algorithm learns what biomarker is associated with what stage and type of colorectal cancer. Training on healthy samples, the algorithm develops a biomarker baseline to compare against. Incorporating both could help Freenome develop a more useful test that can be used broadly on those with and without cancer. Machine learning is essentially “a set of statistical methods meant to find patterns of predictability in datasets” but it is not able to “access any knowledge outside of the data you provide” [8]. If you train your algorithm on only cancerous samples, it may draw the wrong conclusions when confronted with a healthy sample. The algorithm will find patterns for only cancerous biomarkers and will not be able to provide reliable data when those biomarkers are not present. If the goal is to develop an accessible blood test that can be performed on anyone when they visit their doctor, it is critical to integrate these healthy samples.
Do We Really Know What Healthy Is? Freenome has taken a methodical approach to designing its first clinical study. They defined strict parameters, narrowed the number of variables, and established a control and experimental group. However, this design assumes they have identified the correct control group. While research has made impressive progress in understanding how biology works, we have a long way to go; there are still more unknowns than knowns and cancer is a notoriously complex, heterogenous disease [9]. Freenome may need to more narrowly define their healthy control group to make sure the baseline for their algorithms is just that – a baseline. If not, Freenome runs the risk that their algorithms will draw meaningless conclusions rather than predict accurate patterns. The presence of simultaneous diseases or symptoms is another complicating factor that Freenome should consider. The algorithm may draw conclusions based on patterns that are actually related to a disease other than cancer, skewing the results. Understanding enough about human biology to control for these potential errors is critical.
Promising Potential – If We Can Learn Enough We all want to believe in the company that promises to offer a painless, cost-effective way to identify cancer when it is most curable. But an algorithm is only as good as the data you feed it and the questions you ask. It cannot make intuitive leaps beyond what you provide and the patterns it detects. Freenome valiantly strives to solve a global issue with the available research and resources. But do we know enough about human biology and cancer to ask the right questions? Can we accept a margin of error when the stakes are so high?
(Word Count: 800)
[1] “Cancer Statistics,” National Cancer Institute, April 27, 2018, https://www.cancer.gov/about-cancer/understanding/statistics, accessed November 2018.
[2] “Survival three times higher when cancer is diagnosed early,” press release, August 10, 2015, on Cancer Research UK website, https://www.cancerresearchuk.org/about-us/cancer-news/press-release/2015-08-10-survival-three-times-higher-when-cancer-is-diagnosed-early, accessed November 2018.
[3] “Liquid Biopsies: Past, Present, and Future,” Cancer.org, February 12, 2018, https://www.cancer.org/latest-news/liquid-biopsies-past-present-future.html, accessed November 2018.
[4] Yuichi Mori & Shin-ei Kudo, “Detecting colorectal polyps via machine learning,” Nature Biomedical Engineering 2 (2018): 713–714, https://www.nature.com/articles/s41551-018-0308-9, accessed November 2018.
[5] “Liquid Biopsies: Past, Present, and Future,” Cancer.org, February 12, 2018, https://www.cancer.org/latest-news/liquid-biopsies-past-present-future.html, accessed November 2018.
[6] Freenome, Inc. (2018). AI-EMERGE: Development and Validation of a Multi-analyte, Blood-based Colorectal Cancer Screening Test. Retrieved from https://clinicaltrials.gov/ct2/show/study/NCT03688906 (Identification No. NCT03688906).
[7] “Single Blood Test Screens for Eight Cancer Types,” January 18, 2018, Johns Hopkins Medicine, https://www.hopkinsmedicine.org/news/newsroom/news-releases/single-blood-test-screens-for-eight-cancer-types, accessed November 2018.
[8] Anastassia Fedyk, “How to tell if machine learning can solve your business problem,” Harvard Business Review Digital Articles, November 25, 2016, https://hbr.org/2016/11/how-to-tell-if-machine-learning-can-solve-your-business-problem.
[9] Konstantina Kouroua, Themis P. Exarchos, Konstantinos P. Exarchos, Michalis V. Karamouzis, & Dimitrios I. Fotiadis, “Machine learning applications in cancer prognosis and prediction,” Computational and Structural Biotechnology Journal vol. 13 (2015): 8–17, https://www.sciencedirect.com/science/article/pii/S2001037014000464, accessed November 2018.
This is a really interesting area of research and I can’t wait to see how Freenome evolves.
I agree with the author that the dangers of machine learning are predicated on what the inputs of the data are. I wonder what the time horizon of this research is, and how long Freenome plans on collecting data before coming to a workable “conclusion”? If for example, the healthy blood samples are from young men and women (in their 20s) and that colorectal cancer typically doesn’t emerge until 40s, then would Freenome wait 20 years to see if these healthy men and women developed colorectal cancer? If Freenome didn’t wait to see if these healthy blood samples developed cancer, then I question whether or not the data is representative of an accurate population of healthy and cancer patients and whether or not the results from the algorithm are meaningful.
After reading this post, I am left at the edge of my seat. As your numbers portray, cancer affects us all in some way, shape or form. With the hundreds of thousands of lives that are lost each year to cancer, there is clearly a tremendous opportunity for companies to apply breakthrough technology to address this major health need.
What Freenome is doing is incredible. I would recommend that they remain focused on a specific cancer type in order to make serious progress in their ability to effectively predict the actual biomarkers that lead to colorectal cancer.
Although Machine Learning is helping Freenome charge forward with breakthrough oncology research, it is fascinating to still see the critical role that oncologists will still need to play to ensure ultimate success. It reinforces how we are not being replaced by machines/machine learning, we need to learn how to work with machines/machine learning to truly unlock it total potential.
The research Freenome is doing is fascinating! Like Lindsey, I think that defining a control group for these studies will be their biggest challenge in developing an adequate algorithm. I am not very knowledgeable in this subject but my understanding is that we simply do not know enough about cancer, its causes, and like it is mentioned in the article its early stage symptoms, so clearing a group as “healthy” seems incredibly difficult, even with existing research — this is exactly the reason Freenome is doing this study to begin with! From reading this, I am left with the impression that Freenome needs to become very good at identifying patterns of biomarkers in the blood before they can actually use machine learning to enhance the capacity of doing this same identification. In a way, it is a cyclical issue: they need to be good at identifying the trends in order to feed good information to the study, which will become better at identifying trends based on the selection that was made. If they fail in doing this, their control groups will simply not serve their function.
Another question this leaves me with is how easy it would be to apply the same algorithms to other types of cancer in the future. Are types of cancer “similar” enough to be able to leverage the research or will they run into a “watson-type” problem when they try to broaden the applicability of their research?