Machine Learning at Airbnb
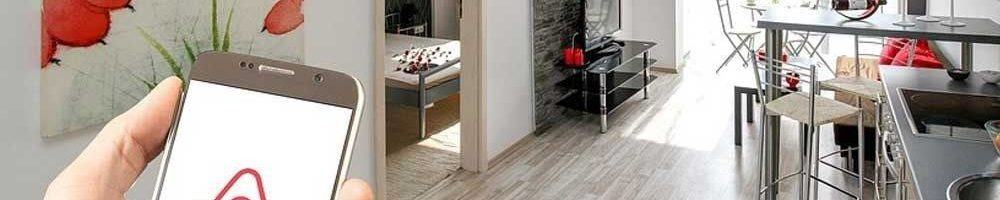
Airbnb is a two-sided rental marketplace, where market dynamics play a key role in matching guests with hosts1. For Airbnb, supply and demand vary drastically across different geographies, different customer preferences and different check-in dates.2 Hence, optimizing matches between hosts and guests is critical to the success. Airbnb offers variety of accommodations; however the corporate is at advantage only when guests can easily find a host that meets their criteria.2 And as, Airbnb adds to its “3 million current listings, ensuring both guests and hosts are satisfied will become more crucial. If users can find the exact accommodation they are looking for, especially if it is at a cheaper price, they are unlikely to revert to using hotels.”2
To optimize this search process for both hosts and guests, it is important for Airbnb to understand and forecast these spatial and temporal trends in order to find better matches and improve experience1. Currently, Airbnb highly leverages on machine learning to crunch data and generate models. “The measurable impact that machine learning has had on Airbnb’s unique technological challenge — creating great matches between guests and hosts — has been “profound,” says the company’s VP of engineering Mike Curtis”.4 Additionally, “Airbnb’s machine learning infrastructure have lowered the cost significantly to deploy new machine learning models to production”.3
How are they addressing the issue?
Airbnb is leveraging machine learning to optimize two processes as below:
- Customer search: “Initially, search rankings were determined by a set of hard-coded rules based on very basic signals, such as the number of bedrooms and price. And these rules were applied to every guest uniformly, rather than taking into account the unique values that could create the kind of a personalized experience.4”As Airbnb decided to scale the operations, it became an imperative to optimize this process. “In 2014, Airbnb created model to personalize search results for guests to easily discover homes. The company uses a machine-learned search ranking model to personalize results for guests. The model factors in guests’ tendencies to click on certain bookings. For example, Airbnb might look at whether customers favor specific types of décor in places they book. The company feeds more than 100 characteristics into the model, which then uses the data to identify patterns and personalize search rankings. It also uses host preferences to personalize search results for guests, promoting hosts likely to accept the accommodation request”.2
- Pricing: Airbnb has heavily invested in machine learning to design dynamic pricing model i.e. offer price tips to the renters based on changing scenarios. It is highly critical for Airbnb to offer hosts an automated source of pricing information to come to a pricing decision in a time efficient manner. “Airbnb hosts vary in their willingness to play concierge, cook, or tour guide. And events—some regular, like seasonal weather changes; others unusual, like large local events”5. Given the model must take in considerations multiple factors, pricing process can be optimized with machine learning processes. “The machine-learning pricing model uses historical travel patterns for the area to find the likelihood of any listing being booked at any time”2. Using this inter-linkages and dependencies of data sets, model generates patterns that can be leveraged to predict right pricing for future host requests.
What could they do differently going forward?
Short term: Airbnb should leverage machine learning in tackling inclusion challenges that it has been facing in recent future. Research says that guests tend to give lower ratings to the hosts if they belong to minority community, which in turn affects the future business of the hosts.6 To calibrate these racial/ community affects in the ratings, Airbnb should put race of the guest/host as one of the filters and then based on historical data calibrate the ratings so that everyone is on the same level for future business.
Medium term: “As the platform expands into tours, activities, restaurant bookings, and concierge services, Airbnb’s future growth will rely on their ability to offer guests ultra-personalized booking services”2. Airbnb should try to leverage machine learning to model guests experience over their customer lifetime cycle. Machine-learning led modeling could use data sets such as age, geography, socio-economic status etc. – values for these data sets will change over time for an individual and hence Airbnb will be able to give personalize services that will differentiate it from the competitors.
Questions to consider
- Can machine learning help Airbnb in tackling regulation challenges that it’s facing in recent future, such as ‘One house One Guest’ model?
- How can Airbnb leverage machine-learning to design customized solutions for different economies?
(word count: 753)
Endnotes
- Sharan Shrinivasan, “The Medium.”https://medium.com/airbnb-engineering/learning-market-dynamics-for-optimal-pricing-97cffbcc53e3, accessed August 2010
- Stephanie Pandolph, “Business Insider.”https://www.businessinsider.com/machine-learning-is-driving-growth-at-airbnb-2017-6, accessed Jun 2017
- Robert Chang, “The Medium.” https://medium.com/airbnb-engineering/using-machine-learning-to-predict-value-of-homes-on-airbnb-9272d3d4739d, accessed July, 2017
- VB Staff, “The VentureBeat.”https://venturebeat.com/2017/06/14/airbnb-vp-talks-about-ais-profound-impact-on-profits/, accessed June 2017
- Dan Hill, “The Secret of Airbnb’s pricing algorithm.” https://spectrum.ieee.org/computing/software/the-secret-of-airbnbs-pricing-algorithm, accessed August 2015
- Deanna Tang, “The Skift.” https://skift.com/2016/06/22/figuring-out-the-tech-solutions-to-airbnbs-racial-discrimination-problem, accessed June, 2016
AirBnB is in a particularly interesting position to leverage machine learning, because of the inherent amount of data a customer provides to transact on its platform. In any business, machine learning is most effective when the company has a lot of data. In retail, for example, it would be priceless for retailers to know every relevant data point on their customers (e.g. size, color preferences, location, gender, age, etc.). However, due to privacy concerns or simply not wanting to spend the time, customers are often reluctant to provide this information.
AirBnB has the luxury of collecting information on customers’ budget, contact information, and various data points on style, size and location of preferred accommodations. I am interested to know how they will apply this technology beyond customer search and pricing going forward.
I think that leveraging machine learning to reduce race and social bias is a strong value-add of its implementation in Uber. One of the outstanding questions of that, however, would be to ask whether having all this information implies Airbnb should have any responsibility over ensuring the bias is actually reduced. The way I see it, it is once thing to point out that there is an issue and create a more level playing field for hosts (and bookers), and another to solve it and put programs in place to raise awareness.
Thanks for the interesting read. I believe machine learning holds tremendous potential in platform/marketplace products such as Airbnb. Perhaps with a large enough training set based on a user’s historical hosting/renting activity, a machine learning algorithm will be able to make house recommendations much more accurately than what the site is able to do currently. I do however, foresee a problem with using historical data to predict a user’s preferences. There is an element of novelty in travelling overseas to experience a new city. Some users might have a better experience if they stay in an apartment that is outside of their usual pattern of preferences.
Awesome article – thanks for sharing! I 100% agree that AirBnB should aim to reduce inherent biases in ratings and ensure that all hosts/guests are treated fairly on the platform. The research says it’s there and I agree it’s on AirBnB management to reduce it. At the same time, however, I struggle to understand how a machine learning algorithm could control for factors like race, gender, etc. How would it work in practice? I’d love to know more how it works at Uber (also in response to @Rio’s comment above).