Lily Health – Scaling access to reproductive health advice with machine learning
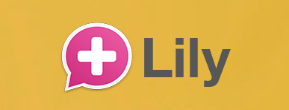
Introduction to Lily Health
Lily Health is an early stage start-up in Nairobi, Kenya that provides women with sexual and reproductive health advice via mobile messaging. While there are a large number of companies that provide women’s health information and period tracking through mobile applications, there was no equivalent solution for women in developing countries who don’t have access to smartphones and instead rely on SMS messaging. Moreover, in many developing countries, women lack access to information about family planning and reproductive health due to stigma surrounding these topics and/or access to the internet or healthcare facilities. Lily provides a subscription service that allows women to text questions to their friend “Lily” and receive responses based on a database of information about reproductive health as well as personalized information about that woman’s cycle. The company currently operates in Kenya but has plans to expand other markets, including India, Bangladesh, Brazil, and the Philippines [1].
Lily’s Machine Learning Strategy
When Lily initially launched, employees were manually providing responses to customers [2]. This manual process is not a scalable solution and would have prevented Lily Health from scaling and reaching the 10M+ young women they hope to eventually serve [3]. Machine learning will enable Lily to create an automated and scalable product that responds to customers using a database of customer details, reproductive health information, and a steadily improving algorithm that interprets customer questions in order to generate accurate responses – a “chat bot” solution.
The management team was forced to slow growth in order to focus on the development of a more robust chat bot. Lily’s customer base quickly grew to a size that exceeded its current capacity. The management team recognized the importance of building a robust technological solution and froze all new customer acquisition while focused on product development. In addition, the company developed a hybrid product – a combination of predictive database responses and human response curation – for use while it refines the product. Because the product has real impacts on women’s health, it is important for Lily to be cautious about the quality and accuracy of its responses during early product testing. In order to support the product under development, the management team has also invested significant capital in upgrading its back-end technology platform. Storing and processing large volumes of data requires a much more professional tech stack than the team’s original “bootstrapped” capabilities [2].
In order to prepare for the medium term, the company has increased its fundraising and talent recruitment efforts. As this solution scales and moves to new markets, the amount of data Lily processes will increase significantly, requiring talent with significant experience managing complex machine learning algorithms. In addition, Lily will require substantial additional capital to expand its technology infrastructure. Lily is designed specifically for developing countries where technology infrastructure often presents additional challenges and higher costs than a similar product might experience in established markets.
Recommendations
While machine learning automation will enable Lily to scale, it also presents a number of ethical and safety concerns as there is a risk of providing individuals with inaccurate information that could impact their reproductive decisions. In addition, there are cultural differences and societal norms that Lily may need to take into consideration as it enters new markets. There are a few actions Lily Health should take to help safeguard against this risk.
First, Lily should increase its in-country partnerships with healthcare providers and organizations. Lily has established partnerships with a few doctors and organizations, but should continue to build out this network in order to build credibility and bring in supporters who can help improve the quality of information provided.
Second, Lily should establish (if it hasn’t already) a comprehensive process for testing and updating its technology. Regular testing should be established to ensure information accuracy and to continually make adjustments that could improve the overall speed and effectiveness of the algorithm.
Open Questions
Technologies like Lily Health often provide “leap frog” solutions to populations that lack traditional access to certain information and services. What responsibility do companies like Lily have to address the underlying issues driving the need for their product (e.g. internet access, social stigma around reproductive health)?
What additional steps, if any, can Lily Health take to make sure it is providing accurate and safe information to its customers?
Word Count: 714
References:
[1]Emil Sjoblom and Macgregor Lennarz. Lily Health Merck Pitch Presentation, May 2018.
[2] Emil Sjoblom. Interview by Leah Coates, August 2018.
[3] Lennarz, Macgregor. “How might we radically improve access to, and quality of, sexual and reproductive health education and services for young people?” OpenIDEO, December 2017. https://challenges.openideo.com/challenge/youth-srh/ideas/lily, accessed November 2018.
I think it is paramount that Lily be aware of the ethical issues of providing accurate and safe information. In the developing world, (but I also believe this to be true worldwide), we need to hold companies to extremely high ethical bars as to not take advantage of , disenfranchise or do harm to those who have been historically underserved and ignored by society.
I also struggle with the issue of companies trying to leapfrog solutions in the developing country context. My view is that there is such a Silicon Valley mindset that’s being applied to international development, where engineers focus on sexy solutions (e.g. a machine learning enabled chat bot) and trying to fit that in to a problem (e.g. lack of access to quality care). Machine learning is a tool that can be used, and is helpful when there is scale, but from your essay it sounds like they have stopped the scale in order to first perfect the tool. That is a very interesting management choice and I would be interested to hear more why management decided to do this – in a setting where inputs are needed to accurately do predictions, I would have thought that scale would have helped make the chat bot more accurate as there would be more data points to understand 1) the types of questions users ask, and 2) how they ask those questions. Applying machine learning to language seems to be a very complicated thing (see: IBM Watson) and I question whether a Kenyan healthcare start up has a comparative advantage in pushing for this innovation.
Educating women about reproductive health is an immensely noble and necessary service and as such I applaud the focus of the company on this. I am very concerned about the potential of incorrect answers though. I was wondering what the behavior of the service is if a woman writes a question that is unclear or where the answer is uncertain. Will the service actually say that it cannot provide an answer or would it go with it’s best “guess” like the Toronto answer in the Watson case? The company would have an incentive to have it provide an answer no matter the certainty to not expose weaknesses. I hope that there is enough oversight to not allow for this to happen.
More fundamentally though, I question the choice of a subscription pricing model. This places a continued financial burden on the women when they might only have questions occasionally. If the mission of the company is to truly reach the most women possible and provide them with information, I wish they had a pay-per-use model in which a certain price is charged on the phone bill each time a text message is sent.