JP Morgan Chase & Machine Learning
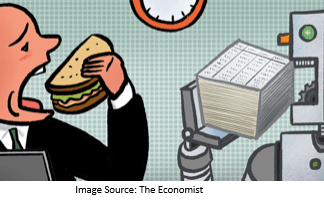
JP Morgan Chase and Machine Learning
Banking has traditionally been a human capital industry from the booking of trades in the back office, to the verification of contracts in the middle office to serving customers in the front office. There are also many cross-functional departments that enable core function of banks to operate such as legal and human resources. Like many publicly traded companies, big banks face pressure to improve their operational efficiency, deliver shareholder value, while growing revenue, improving margin, and increasing total assets. One way that the banking industry is tackling this challenge with machine learning thus making investment in innovation critical to remaining competitive. In the banking sector, machine learning is currently used in several forms: chat bots, profiling customers, streaming lining processes (from client interactions to managing regulatory risks), spotting patterns (to detect potential fraud), and risk management[1]. The mega trend is important to JP Morgan Chase not only as one of the biggest banks in the US market, but also as a company that looking to invest to gain a competitive advantage in the industry. So far it has been able to capitalize on machine learning through the use of new technologies primarily in the area of streamlining processes, profiling customers and chat bots[2], and are investing in furthering in the development of the bank’s technological capabilities.
JP Morgan Chase has over $2.6 trillion in assets and operates with over 250 thousand employees[3], however to deliver on its corporate mandates, the bank has made substantial investments in technology, with multiple machine learning based implementations. One area where this implementation has been successful is with its Contract Intelligence Platform. This platform is used to analyze commercial credit agreements that would normally require 360 thousand hours of labor to and with the new platform the work can now be done in seconds[4], essentially reducing the necessity for labor in this middle office processing task. This becomes critically important to the organization’s management of process of improvement because not only is it improving operational efficiencies, but it also serves the function of removing human error that can occur with manual reviews.
JP Morgan Chase is leveraging machine learning in the short-term to match clients with investing solutions to not only reduce labor but also with the implication of improving customer satisfaction. Internally the company has also released a chat bot to address 120 thousand employee ticket requests with a medium-term goal of efficiently processing the 1.7 million that it receives annually. When considering the medium-term strategies of the bank, JP Morgan Chase has invested over $9 billion towards new technologies with $3 billion dedicated towards new initiatives with an allocation devoted to partnerships[5].
Additional steps that I would recommend JP Morgan Chase to take in the short-term as they invest substantially in developing new technologies is to consider focusing developing technologies that will further enable their front office to be more efficient. An example of this would be using machine learning in developing an optimal robo-advisory program that can automatic generate sales pitches. This can be accomplished by using the patterns of buzzwords and trends that are proven to empirically enhance the propensity of clients to engage in transactions. In the mid-term using machine learning can also enable the firm to better understand how it is performing versus competition in markets where banks are obliged to disclose cost and performance scenarios on a trade by trade basis.
When considering the medium-term use of machine learning, I would recommend JP Morgan Chase consider the impact that the integration of machine learning will have on the bank’s current operational business model and strategy. Although machine learning has the ability to improve process within the front, middle, and back office of the bank, there are also potential ramifications to employees and culture. Therefore, it will be important to plan team structures and training so that employees whose role’s may become redundant with the further integration of new machine learning technologies will have an opportunity to move cross functionally within the bank.
In the context of this organization, an important open question that I am unsure about that merits comments from my classmates related to machine learning in the context of JP Morgan Chase is how will smaller banking entities be able to compete? Additionally, could substantial investment in machine learning potentially lead to further consolidation of the banking industry within the United States if smaller banks cannot compete? (787 words)
[1] Financial Times AI in banking: the reality behind the hype https://www.ft.com/content/b497a134-2d21-11e8-a34a-7e7563b0b0f4
[2] Financial Times AI in banking: the reality behind the hype https://www.ft.com/content/b497a134-2d21-11e8-a34a-7e7563b0b0f4
[3] JP Morgan Chase www.jpmorganchase.com
[4]Tech Emergence AI in Banking – An Analysis of America’s 7 Top Banks https://www.techemergence.com/ai-in-banking-analysis/
[5] Tech Emergence AI in Banking – An Analysis of America’s 7 Top Banks https://www.techemergence.com/ai-in-banking-analysis/
I agree with you that by leveraging machine learning technology, bigger banks like JP Morgan appear to have a strong competitive advantage that smaller banking entities might not be able to afford. Perhaps the smaller banks might be able to promote the “human” or “service” aspect of their functions to better relate to customers, but I think ultimately the results will trump all other factors.
In terms of other front office disruptions that new technologies might be able to impact, it would be interesting to see if machine generated sales pitches are as effective. Quantifying effects and perhaps running A/B testings will be imperative to determine the effectiveness of these pitches.
Excerpt from the New York TOMs review:
Although the author raises the prospect of consolidation within the banking industry due to the impact of machine learning, the rest of the article gives the impression of a trend in the opposite direction. If a chatbot can address a significant portion of 120,000 employee tickets, shouldn’t the order of magnitude improvement in efficiency allow smaller banks to compete at a level that would previously have been impossible? Parallel developments have taken place in the lending industry, with companies such as Affirm leveraging machine learning to make smart lending decisions without the benefit of JPMorgan’s scale. Unless the machine learning models involved rely on deeply trained neural networks, which require up to thousands of GPU-years of training and can be cost-prohibitive for smaller firms, machine learning seems more likely to act as a democratizer that replaces the labor of dozens or hundreds of employees with that of a single computer.
I worked on the JPM Trading floor, and we had a huge push to go to electronic trading. We did everything in our power to get people to stop high touch service, but we quickly learned at a certain threshold of capital people want to have “someone on the other side of the phone”. Honestly, I think this is something that can change, but it was an interesting learning. We assumed it is because clients wanted someone to blame when things went wrong. As younger managers move up on the buyside, this will probably shift.