Is machine learning the new wingman?
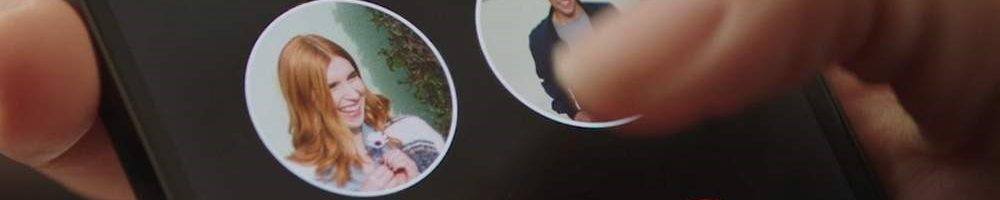
Machine Learning is Taking-Over the Online Dating Industry
Match Group and the Dating Scene
Today, 4% of the global population, utilize online dating for their matchmaking needs. This equates to approximately 291.8 million people.
Match Group is the “world’s leading provider of dating products with a global footprint of marque dating assets [1]”. The Match Group operates a portfolio of dating brands, including Tinder, Match.com, PlentyOfFish, Meetic, OkCupid, OurTime, and Pairs, each designed to increase its users’ likelihood of finding a meaningful romantic connection. The group provides tailored dating products in 42 languages across more than 190 countries to over approximately 8.1 million subscribers each year [1]. For most consumers, however, the experience can often become never ending swiping sessions [6] filled with “mismatches” (i.e., profile offerings that are not in line with the single user’s dating preferences).
Artificial intelligence is progressively entering the dating world. Match Group’s size allows the company “to access an unprecedented amount of consumer dating preference data” [5] (e.g., location, age, gender, sexual preferences, online purchasing history and even music playlists), in which the company has decided to leverage by investing heavily in artificial intelligence and machine learning as a part of its business model. According to Greg Blatt, former Chairman and CEO of Match Group, “Artificial intelligence is just starting to scratch the surface, but we’re making a huge investment in it” [2]. The investment is aimed to help the company remain the industry leader of the incredibly competitive online dating market. To improve user experience, Match Group has embarked on a journey to implement machine learning to help you find your perfect match.
How Match Group Uses Artificial Intelligence:
Match Group’s namesake brand, Match.com, has introduced Lara, ”the first artificial intelligence dating assistant that can help website subscribers optimize their dating profile to attract potential desirable matches” [3]. The company partnered with Google to develop this dynamic service to help singles looking for love. Built with deep artificial intelligence and keyword recognition technologies, Lara is created as a personal wingwoman who can understand your intentions and help you through your dating journey[3]. The chatbot will select a unique member profile based on your search preferences, suggest ideal locations where you and your date can get to know each other based on shared interests and give dating advice to keep your first date jitters at bay. Lara learns from her interactions based on user feedback and will suggest more relevant date locations as well as provide more unique dating advice by leveraging her prior interactions[3].
Looking Ahead
Looking forward, Tinder has begun to roll out a feature called “super likeable”. Tinder has leveraged the latest advancements in machine learning and natural language processing to generate behavior data, learn your “type” of partner and offer a more personalized recommendation. However, according to artificial intelligence consultant, Marina Pavlovic Rivas, this comes with its fair share of risks. “Artificial intelligence is not neutral. It feeds off the information we give it, which can include biases that the algorithm will then generalize. In a society ruled by algorithms, artificial intelligence could, for example, deduce socio-economic status from a ZIP code, and propose only profiles coming from the same background as its users. The same could happen for skin color.” [4]. This could be a societal issue at large and could expose any one of the Match Group’s portfolio companies to legal risk.
Further, Sean Radd, CEO and Founder of Tinder expects machine learning to help users escape information overload. According to Radd, “Five years from now instead of scrolling, searching, swiping I think these devices will be intelligent enough to just give you the answer through a Siri-style interface”[6]. This adjustment would change the act of swiping right or left as we’ve come to know it and essentially create an interactive matchmaker that can be carried anywhere in your pocket.
Recommendations:
With these innovations in dating technology, Match Group needs to remember the societal implications of their algorithms. The company’s platforms are becoming increasingly aware of the impact they have on dating discrimination. In the short term, I recommend they remove certain assumptions from their data sets (e.g., zip codes). This could combat the likelihood of associating certain inputs with a negative connotation. Further, to deepen its innovative strategy, Match Group should increasingly use face recognition to identify if an uploaded profile picture would be seen as attractive by another user. This will allow for more positive matches and an overall beneficial user experience, further increasing the company’s competitive advantage.
What’s Next?
The question remains – how do you program algorithms to disregard biased consumer data that could potentially negatively impact both your consumers and society at large? Given such risks, should Match invest more heavily in machine learning? Do we even still need humans to know what the heart truly wants?
[1] Iac.com. (2018). Match Group | IAC. [online] Available at: http://www.iac.com/brand/match-group [Accessed 13 Nov. 2018].
[2 Bary, E. (2018). Tinder Has Big Plans for Artificial Intelligence. [online] Barrons.com. Available at: https://www.barrons.com/articles/tinder-has-big-plans-for-artificial-intelligence-1493850214 [Accessed 13 Nov. 2018].
[3] Match UK. (2018). Match launches UK’s first AI Dating Chatbot, Lara, on the Google Assistant – Match UK. [online] Available at: https://uk.match.com/pages/advice/ai-dating-chatbot-lara [Accessed 13 Nov. 2018].
[4] Greenacre, M. (2018). For Dating Sites, Artificial Intelligence v. The Human Heart. [online] Worldcrunch.com. Available at: https://www.worldcrunch.com/tech-science/for-dating-sites-artificial-intelligence-v-the-human-heart [Accessed 13 Nov. 2018].
[5] The Economist. (2018). How the internet has changed dating. [online] Available at: https://www.economist.com/briefing/2018/08/18/how-the-internet-has-changed-dating [Accessed 13 Nov. 2018].
[6] Startupgrind.com. (2018). The Future of Dating Is Artificial Intelligence. [online] Available at: https://www.startupgrind.com/blog/the-future-of-dating-is-artificial-intelligence/ [Accessed 13 Nov. 2018].
As you mentioned in your blog post the dangers of the vicious bias loop in the algorithm are quite considerable. Effectively, if we feed biased data to the machine, it will output biased recommendations. But putting this aside for one moment, I´m also concerned that if we assume that past performance can always predict future tastes, we are not giving an opportunity to the scenario in which a consumer’s preference actually change (maybe because she/he is at a different stage of her/his life and prioritizes different things). I think the algorithm should also have some sort of adjustment considering the lifetime of the user to avoid this loss in quality of prediction.
Great essay! I am thinking a little more about your recommendation to remove some variables from the data set (e.g. Zip codes) to reduce potential biases. Does removing some of these variables potentially negatively impact the quality of the matches produced? For example, you may find what appears to be a great match but then discover that you live hours away from each other. Could this scenario negatively impact the perceived value of the app? Additionally, might the system eventually begin to intuit this information? If a subset of the population for one zip code exhibits similar behaviors internally, then the system may begin categorizing those people together anyway and continuing to make decisions based on this category. The machine may “learn” the zip code relationships informally despite not being fed the information directly.
It is interesting to see how nowadays people want to limit first physical interaction and opt to use Tinder or match.com to get dates. One might argue that technology helps facilitate those interactions more efficiently. However, it might perpetuate certain biases about people. How do you know whether one is a good fit for you just purely based on works he or she describes herself in an online post or some Instagram filtered pictures? In the future, those dating apps might incorporate more human-centric designed features to help connect humans more on an emotional level than just helping them swipes to get dates.
I wonder what happens when the user’s dating preference changes or when a user decides to switch from casual dating to serious dating. You said in your analysis that “the chatbot will select a unique member profile based on your search preferences, suggest ideal locations where you and your date can get to know each other based on shared interests and give dating advice to keep your first date jitters at bay.” The problem I see with any machine learning algorithm is that what happens when your historical behavior (i.e. your search history) is not a good indicator of your future preference, or better yet, it is not a good prediction of what is actually a good match for you? How can machine learning advance its algorithm in a way that it not only predicts a likable partner based on superficial elements to the user but also finds a partner that is actually good for both parties?
Interesting to think that in five years it would be possible to match someone, without even asking the consumer to “scroll, search, swip”. Impressive to imagine that even when it comes to the heart, the machine learning is being used in a rational manner to update your preferences in such an objective way. In this sense, I have a hard time believing that consumers will allow the future algorithms to decide more precisely, without they having a more emotional connection through the process. I was also wondering while reading this article, that the new generations are changing a lot of what was dating in the past, for their preferences, for trying new things, and algo age connections. And I would suggest that machine learning for the future apps consider that the trend is changing, and not use totally biases and historical data to predict and pairing potential couples for the future.
Very interesting essay with a very interesting display name! I’m intrigued by your recommendation to remove certain variables to avoid discrimination, e.g. postal code. There are inherent trade-offs between having complete data that could help to fine-tune algorithm, and adding bias which are inherent in input and feedback loop since those are done by human. In fact, the fact that machines could eventually pick up patterns to quickly understand what you’re looking for is dangerous in itself. In that scenario, we would be stuck in our original preferences without given opportunities to branch out and learn from divergence. Information overflow is pushing us to rely on machine to screen data, but what if that’s preventing us from receiving the diverse data points we need?
I think its fascinating that you have written on this subject. For many people in our society, dating remains a very difficult task, particularly because its emotion driven and its really hard to quantify what are the factors that lead to someone feeling that “lighting” love and attraction emotion bolt that is caused by romantic attraction. To that end, I do feel machine learning is important here and if you can identify correlations between what attracts people, its should be fine for the algorithm to provide that. Getting the wrong partner could be a lifelong issue, so any help these machine learning-based logarithm can help you minimize Type 1 errors while still incurring some Type II errors is potentially fine if it helps speed things up for you and get you a better match to date and hopefully ultimately marry!
Interesting application of machine learning! I think in this case, our personal opinion on who we think we would be compatible with are much more biased than AI’s applications. Many times, what qualities are qualifications we think we will like are very different than who we actually get along with. For example, I can be picky and filter strongly on height or age in an application, but many times I don’t realize those are things I’m more than willing to put aside if I find the right person. However, apps don’t give you the chance to meet those potential people who don’t meet all your qualifications because they are filtered out from the start. By using AI, I think there would be less bias because you are given recommendations based on things like purchase history and searches. Those things will show you what your interests and hobbies are. I think AI will actually remove our preconceived biases and give us better matches.
Very interesting read. My article is similar but focused on Bumble. My analysis is that Machines would definitely get better and more efficient at matching single people looking for love. However, that is contingent on the amount of users they have as well as the number of data they can collect per user. Given this, do you think Match.com will start consolidating different platforms so they can cross-match people and generate greater efficiency? In addition, once the algorithm has been significantly optimized, do you think the economics model of these apps will be different? (e.g., charge you a lot more per match given its accuracy instead of providing the service to its users for free and make money off subscription and advertisement).
Great article and interesting read! While online/app dating is becoming more common and acceptable, it still remains challenging for certain demographics, and I think there’s real potential for ML/AI to play a role in improving the customer experience for these groups. This reminded me of a story that I read when Apple introduced the facial recognition technology; however, the technology was often more difficult to use for people of color when it was first introduced because of the population it was tested with. I’m curious to get your thoughts on algorithmic justice and how should companies/employees be more thoughtful about the technology they’re building.
Great article! I actually wonder if dating apps already emphasize our biases as they are. When we swipe, given that we only have a picture, a city/school, and maybe a couple of words, I believe we are already making extremely biased decisions. We make assumptions based on just that very limited information, about how much we will be compatible with someone. Because of this, I’m not really convinced that the machine learning will make the dating apps any more biased than we already are, but rather that they will just mimic our existing biased behavior. If they focus their efforts on feeding us people similar to those that we have swiped right in the past, then it will in fact be more efficient and likely yield a higher matching rate, but in the end I think the percent of lasting relationships won’t change much. I actually think dating apps have a unique opportunity to reverse our biases, help bring people of different backgrounds together, and connect us with people that we might not have swiped right on based on just our past swiping behaviors, instead showing us people that they determine that we actually have things in common. I almost think of it like arranged marriages; though controversial, I can totally believe that our parents could actually know who would be a good match for us. In arranged marriages, the focus is on compatibility and values, which frankly are likely to be more important in a long-term relationship than mere physical attraction. With all the data that Match and Tinder have on us, they would likely be able to find us people that we are compatible with on an emotional level, perhaps better than we could ourselves, and that we may have missed when swiping only based on only looks. Maybe the concept of replicating arranged marriages isn’t the most romantic.. but how romantic is Tinder really anyways?
Very interesting article. Currently the users on dating apps face paradox of choice problem. In the future, AL and ML have the potential to create a highly customized list of matches for these users. I believe that in the competitive world of online dating, the companies that can crack this code first will setup themselves up for long-term success. Users are swiping for their preferences on these apps for hours every month and this existing data can be leveraged to create future matches that these users are much more likely to find suitable.
This is interesting. Though I think it’s possible for a machine learning algorithm to identify whether or not a person will be attracted to another, I’m skeptical about whether such an algorithm can predict long-term compatibility between partners. It would be difficult to collect the numerous data points necessary to make any conclusion related to the factors that predict such compatibility. Related to this, it would be interesting to see how Match Group’s revenue model could change if the company’s customers judged its value based on long-term success (i.e. staying together with their partner). Finally, suppose Match Group’s algorithm is widely successful and more accurate than human judgement. What then becomes the purpose of courtship and dating? Should we all just accept marriages arranged by a machine?