Increasing financial security with machine learning
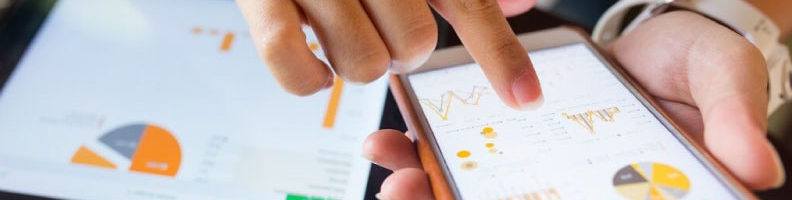
Introduction: using machine learning in household finance
Household finance is one area where machine learning has the potential to positively impact consumers’ lives at scale. Mint.com has developed algorithms to classify each of its 10 million users’ expenses and predict when they will be approved for credit cards.[1] Even Responsible Finance (Even) is developing algorithms to provide consumers with spending recommendations based on their recent and expected expenditures; founded just in 2014, Even has raised over $40 million in venture funding and landed a pilot to test the technology on workers of a leading retailer.[2]
Against this backdrop of rapid change, I am working on a new venture (name withheld) that seeks to work with large employers to improve the financial health of their workforces. Our core thesis is that highly personalized interventions that measurably improve an individual’s day-to-day financial security will have an outsized impact on retention rate, productivity, and other outcomes of interest to their employer. Packaged up as a decision engine, these relationships can guide employers to expand employee benefits with positive impact on both consumer financial health and their bottom lines.
Several factors explain why machine learning is core to our business model. First, our value proposition is based on our ability to predict, and help affect, future workplace outcomes (retention and productivity). Second, employers’ orientation toward data-driven business decisions in HR is rapidly increasing (evidenced by the newly coined “people analytics”).[3] Third, there has been a convergence of both the availability of data (including the digitization of information through financial data APIs such as plaid.com) and a realization in the market that one-size-fits-all financial services dramatically fail to meet the needs of consumers.[4]
What we are doing in the short- and medium-term with machine learning
Several actions are already being undertaken to prepare us to successfully use machine learning to predict workplace outcomes on an individual basis based on consumer financial data. In the short term, we are engaging in highly qualitative user research, giving us unprecedented contextual information about user needs that guide our feature engineering and regularization efforts so that we know that we are only using relevant and necessary predictors across different types of consumers.[5] We are also doing customer discovery work with employers in order to: (1) refine specific outcomes of most interest and (2) develop an understanding of available workplace data availble on a recurring basis to predict these outcomes.
There are several steps being taken to position us well in the medium term. First, since,building a comprehensive picture of each user’s financial life requires accessing highly-personal information, we are investing heavily in understanding constraints related to data privacy, an increasingly salient issue today. This has two major implications: first, we must focus other elements of our product development (user interface design, messaging, etc.) on maximizing trust and openness to sharing; second, our product must still function with users who have granted partial access to their data, likely requiring a “menu” of algorithms with varying or flexible data requirements. Second, an important element of our product roadmap is an “ROI calculator” that turns estimates into an actual estimation of financial savings to each partner employer that result from their workers’ uptake of the financial benefits that we recommend. This will require us to continually ensure we are collecting sufficient predictive and contextual data in order to reliably build decision engine that maximizes estimated ROI for each employer we work with.
Other anticipated challenges that we will need to address*
There are several challenges to the use of machine learning in this space that require our attention. The first challenge is the “cold-start” (or “chicken and egg”) problem that many other data companies face: since this is a relatively un-addressed business problem to date, our binding constraint to the development of value-creating predictive models is the amount of data that we have at our disposal, rather than algorithm development itself (additionally, since it is uncertain whether we’ll ever operate in the world of truly “big” data with multi-million row datasets, neural networks and other highly advanced classes of algorithms likely lead to overfitting).[6] We must in the short term rely somewhat on the founding team’s expertise in order to convince an initial set of partner employers to give us access to their workers. A second challenge is “explainability”: by design, we will create value through generating personalized recommendations that may appear unequal with regard to the amount or nature of financial support between workers.
Despite these challenges, we are optimistic about the transformative social impact these innovations can bring. One key question we have is: if you were a manager with a large low- and middle-wage workforce, how would you think about the potential use of machine learning in an employee benefits system like this?
(word count: 800)
*As a co-founder, “recommendations” are already part of the existing product roadmap, discussed in the second section.
References:
[1] Dustin Blitchok, “Now In Its 10th Year, Personal Finance App Mint Is Investing In AI, Data,” Benzinga.com (website), October 18, 2017, https://www.benzinga.com/fintech/17/10/10183117/ now-in-its-10th-year-personal-finance-app-mint-is-investing-in-ai-data, Accessed November 12, 2018.
[2] Matthew Boyle, “Walmart’s Fintech Partner Helps Break Paycheck-to-Paycheck Cycle,” Bloomberg.com (website), July 19, 2018, https://www.bloomberg.com/news/articles/2018-07-19/walmart-s-fintech-partner-helps-break-paycheck-to-paycheck-cycle, Accessed November 12, 2018.
[3] Josh Bersin et al., “People Analytics: Gaining Speed,” Deloitte Insights (website), February 29, 2016, https://www2.deloitte.com/insights/us/en/focus/human-capital-trends/2016/people-analytics-in-hr-analytics-teams.html, Accessed November 12, 2018.
[4] Laura Brodsky and Liz Oakes, “Data sharing and open banking,” McKinsey Quarterly (website), September 2017, https://www.mckinsey.com/industries/financial-services/our-insights/data-sharing-and-open-banking, Accessed November 12, 2018.
[5] Mike Yeomans, “What Every Manager Should Know About Machine Learning,” Harvard Business Review (website), July 2015, https://hbr.org/2015/07/what-every-manager-should-know-about-machine-learning, Accessed November 12, 2018.
[6] Trevor Hastie, “The Elements of Statistical Learning: Data Mining, Inference, and Prediction, 2nd Edition,” Spring Series in Statistics, February 2009, https://web.stanford.edu/~hastie/ElemStatLearn/, Accessed November 12, 2018.
Very cool article and very cool company!
One question I had was whether you anticipate much conflict between the outcomes that employers are optimizing for and the ones that employees would optimize for with perfect information? Overall I agree with your thesis that there is a lot of alignment between good personal finance and good professional performance, but could there be some situations on the margin where the models would recommend solutions that are not in the best interests of the workers?
Particularly in the case of low-wage workers that you brought up, if the company is paying for this solution, and the suggestions are not truly mutually beneficial, who would you side with? One the one hand, you want to maximize the benefit to your customers (the companies), but you also want to avoid reputational or legal risks from hurting employees.
Maybe these will just be edge cases, but would be interested to hear more about it! Again, great idea, and good luck!