If an Algorithm Wrote This Blog Post, Would You Be Able to Tell?
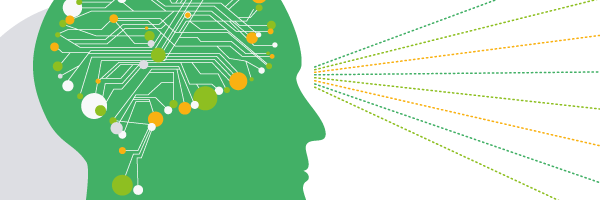
Narrative Science's value proposition is to transform data into a narrative indistinguishable from the writing of a human. How will software-generated content impact the future of journalism?
If an algorithm wrote this blog post, would you be able to tell? Chicago-based startup Narrative Science wants your answer to be “No!” Its business value proposition is to transform numerical data into a narrative indistinguishable from the writing of a human. Its proprietary natural language generation (NLG) software, Quill, takes as input raw quantitative data such as financial earnings reports and sports statistics and converts it into human-like, easily digestible prose that communicates the same takeaways a human would derive from analyzing the data.[1]
To better grasp the power of Quill, consider Exhibit 1 below. Quill’s NPG algorithm automatically converts the input data into the output narrative without relying on any sort of human curation.
Exhibit 1 – Input Data and Output Narrative [2]
To be clear, Quill is a lot more than just fill-in-the-blanks software that feeds data into human-written templates. Instead of relying on hard-coded formulas, Quill takes a Bayesian approach based on customized text corpora to construct free-flowing narratives tailored to each dataset.
Narrative Science and other players in the Artificial Intelligence community have made remarkable progress toward bridging the gap between traditional and automated content. A recent study published in the journal Journalism Practices asked readers to assess both types of content on 12 attributes: Coherent, Descriptive, Useable, Well Written, Informative, Clear, Pleasant to Read, Interesting, Boring, Accurate, Trustworthy, Objective. The study found a statistically significant difference in readers’ perception for only one metric: Pleasant to Read. Across all other measures, readers were unable to discern the kind of software-generated content created by Quill from human-written news articles.[3]
(The New York Times has an interactive feature where you can try your hand at telling the two apart.)[4]
Narrative Science’s innovative product has the potential to greatly disrupt journalism’s century-old operating model structured upon human writers. In a time when much of the news industry’s revenues are declining due to the widespread availability of online free content coupled with the big tech companies’s stronghold on content distribution and online advertising, this so-called Robot Journalism may represent a silver lining for the future of the industry.[5] Natural language generation software such as Quill can scale down newsroom labor costs and increase reporters’ productivity by reducing the time they need to spend doing busy work such as data processing and freeing them up for more value-added undertakings such as on-the-ground reporting.
While the majority of Narrative Science’s clients are business analytics enterprises such as Microsoft Power BI and Tableau, the start-up has already started tapping into the editorial market: One of its partner organizations is Forbes, a highly respected news outlet.[6] Kris Hammond, one of the co-founders of Narrative Science, has famously predicted that software-generated reporting will win a Pulitzer Prize by 2020.[7]
A shift toward the news industry is not without its risks for the company, however. Firstly, natural language generation algorithms are only as good as their input data. “What … Narrative Science, and other algorithmic approaches to information will need is good data. Some data will come from municipalities, other data will come from the private sector, nonprofits, and academia, and some will be created by media organizations themselves using sensors and scrapers,” explains Alexander Howard in The Art and Science of Data-Driven Journalism.[8] For Narrative Science, this means the perceived quality of its product is largely tied to a data collection process it has little control over.
Moreover, there is great concern in newsrooms about the impact of algorithmic content to the upholding of journalism’s editorial standards.[9] While laymen tend to see algorithms as inherently objective and credible, many editors view algorithm design processes as containing a lot of hidden biases which may compromise the editorial integrity of their vehicles – particularly when the algorithm powering content creation is not open source, as in the case of Narrative Science.
To tackle the risks posed by low-quality input, Narrative Science should consider investing in research to make Quill capable of handling more than just highly structured numerical data. For now, Quill is only able to process structured data that easily falls under the parameters of Knowledge Bases.[10] The ability to process unstructured data (i.e. text) would vastly improve Quill’s algorithmic resilience. (To that end, the work done by the IBM Watson team provides a great foundation for Narrative Science to build on.) Concerns about biases embedded into Quill’s algorithm can be largely appeased by transparency, meaning the disclosure of the algorithm’s inner workings. If algorithms are to replace human reporters, they must be held accountable to the same editorial standards that safeguard journalism’s societal role today.
(Word Count: 759)
References
[1] Narrative Science, “Quill,” NarrativeScience.org, https://www.narrativescience.com/quill, accessed November 2016.
[2] Steve Lohr, “Start-Up Lessons From the Once-Again Hot Field of A.I.,” The New York Times, February 28, 2016, http://www.nytimes.com/2016/02/29/technology/start-up-lessons-from-the-once-again-hot-field-of-ai.html, accessed November 2016.
[3] Christer Clerwell, “Enter the Robot Journalist,” Journalism Practice, Vol. 8, no. 5, p. 519-531, February 25, 2014, http://dx.doi.org/10.1080/17512786.2014.883116, accessed November 2016.
[4] NYT Sunday Review, “Did a Human or a Computer Write This?,” The New York Times, March 7, 2015, http://www.nytimes.com/interactive/2015/03/08/opinion/sunday/algorithm-human-quiz.html, accessed November 2016.
[5] Damian Radcliffe, “The Upsides (and Downsides) of Automated Robot Journalism,” MediaShift, July 7, 2016, http://mediashift.org/2016/07/upsides-downsides-automated-robot-journalism/, accessed November 2016.
[6] Tom Simonite, “Robot Journalist Finds Work on Wall Street,” MIT Technology Review, January 9, 2015, https://www.technologyreview.com/s/533976/robot-journalist-finds-new-work-on-wall-street/, accessed November 2016.
[7] Tim Adams, “And the Pulitzer Goes To… a Computer,” The Guardian, June 28, 2015, https://www.theguardian.com/technology/2015/jun/28/computer-writing-journalism-artificial-intelligence, accessed November 2016.
[8] Alexander Howard, “The Art and Science of Data-Driven Journalism,” Tow Center For Digital Journalism, May 30, 2014, http://towcenter.org/wp-content/uploads/2014/05/Tow-Center-Data-Driven-Journalism.pdf, accessed November 2016.
[9] Shelley Podolny, “If an Algorithm Wrote This, How Would You Even Know?,” The New York Times, March 7, 2015, http://www.nytimes.com/2015/03/08/opinion/sunday/if-an-algorithm-wrote-this-how-would-you-even-know.html, accessed November 2016.
[10] Joe Fassler, “Can the Computers at Narrative Science Replace Paid Writers?,” The Atlantic, April 12, 2012, http://www.theatlantic.com/entertainment/archive/2012/04/can-the-computers-at-narrative-science-replace-paid-writers/255631/, accessed November 2016.
Featured Image
Narrative Science | Quill, “Head Vision Narrative Analytics,” NarrativeScience.com, https://www.narrativescience.com/filebin/images/Narrative_Analytics/headvisionnarrativeanalytics.png, accessed November 2016.
Interesting post Nik. I agree that while there are several hurdles still to overcome, the technology definitely has the potential to improve journalism by taking over the lower value-added activities and let journalist focus on the high value-added components of reporting. I feel however that there is one element missing from your discussion: reader acceptance of automatically generated content. While it may be factually true that texts are indistinguishable from human-written texts, I am not sure an investor will accept automatically generated content with regards to investment advice, or even a general newspaper reader will accept automated reporting on his/her community. Such user resistance to adopt/accept this change, has slowed down many innovative products in the past and is not an element to be underestimated [1].
[1] https://www.washingtonpost.com/news/innovations/wp/2016/07/21/humans-once-opposed-coffee-and-refrigeration-heres-why-we-often-hate-new-stuff/
Nik, your article has got me imagining all sorts of dystopian futures where our perception of the world is completely divorced from reality, and from each other’s reality. Some would argue we are already experiencing this.
First, I imagine a situation where a bunch of robots do some algorithmic trading that humans could not possibly keep up with. Then, a bunch of other robots write investor reports and news articles about this trading. As long as things are going well, the news articles will be glowing. So the trading robots pile in more money into their bets and we have a robot-to-robert feedback loop with very little human judgment. Admittedly humans have failed in spectacular ways in our ability to spot bubbles and avert crises, but reducing the judgment element even further seems like a recipe for disaster.
Second, I imagine a situation (described in a Slate article) where robot reporters write personalized news stories for each reader. [1] In the article, the author gives an example of a story on Angelina Jolie – a reader interested in global events would get a paragraph about her work with refugees, while a reader interested in celebrity gossip would get a paragraph about her marital troubles. Having already seen how different perceptions of reality have created huge political divides in the US, I worry that this technology will further exacerbate the differences in how people view the world, and therefore exacerbate our ability to live and govern together.
All this is to say, I am sure that Narrative Science has many valuable applications, but, like with all AI technologies, we need to figure out ways to incorporate human judgment and oversight into its uses.
[1] http://www.slate.com/articles/technology/future_tense/2012/03/narrative_science_robot_journalists_customized_news_and_the_danger_to_civil_discourse_.html
I was interested to hear about how algorithms can reflect implicit biases on behalf of the coder, which on second thought is not so surprising because codes are a human creation.
Seems like there are a few competitors in this space, including Automated Insights. Obviously the existence of multiple companies suggests that people view this as a profitable market one day, reinforcing your point around the technology’s potentially disruptive nature. Would be curious to know how big the estimated market is, and what other applications could be viable beyond just journalism.
I was also shocked to see a big PE firm (Vista Equity) investing in this space via the purchase of Automated Insights. Based on this article, it seems like a lot of the big tech companies like Google and FB are pouring money into this space as well. As a result, I’m curious to see who wins in this marketplace between players like Quill and others.
https://automatedinsights.com/
https://techcrunch.com/2015/02/12/automated-insights-the-startup-behind-the-aps-robot-news-writing-gets-acquired-by-vista/
I think this post leaves out a key component of the potential success of a business like Narrative Science. That component is consumer demand for AI-generated news (or other content). Trust, especially in the news world, is a major hurdle that is not easily earned. With the rise of Fake News on sites like Facebook, and all the negative attention paid to it since the election, I am skeptical that a company like this can easily earn the trust of readers. Second, we mostly consume news today with a subjective slant or wanting to hear the opinion of the author of the article. Whether its cable news or a prominent columnist, more and more, readers are hoping to get the opinion of someone, not straight objective news. While I see potential limited application for aggregators like the AP, I do not see widespread adoption by major papers. With the business struggling (as the article mentions), papers will more rely on the differentiating aspect of its journalists and their voices. This business eliminates that, which is why I do not see it as compelling service in the long run.
Nice try Watson, almost had me believing you were a human. I think journalism will be one of the last spaces where algorithmic writing threatens jobs, as I view it in a similar way to acting or other artists. In theory, virtual actors can largely replace real life actors even with current technologies, but the consumers of the product still prefer that emotional connection they develop with the actor. Journalism is very similar, and in many instances consumers seek out articles made by specific authors for a specific opinion. Look forward to seeing you write a few NY Times articles, though, Watson.
Jaime
It is great to see that you’ve touched on the biases and low-quality input issues. To further elaborate, simply, these algorithms were created by humans, thus the “inputs” to the algorithms are man-made and inherently that means they are limited in their capabilities, and potentially flawed/biases as you pointed out above. In general, I am all for technology to improve our lives. However, aside from the issues above, my overall problem with using digitization to replace human beings is that I’m not convinced that these technologies will be able to fill in for our human’s blindspots. When it comes to the criteria used in building these algorithms, there is the potential that the filtering elements of the algorithms may suffer from some of the negatives of quant screening. For example, in quant screening, greater than 10% may prove to be too limiting if the stock’s value is 9.99%. Perhaps if there was a fundamental overlay on the output of quant models in 2007, the quant crisis may have been avoided or its negative impact minimized?
Overall, I think there is a synergy between technology and humans, in the same way that there is research showing evidence that there is a synergy between quant and fundamental investing strategies (http://www.barrons.com/articles/SB50001424052748704567604578410611617451922). We should see both options as complements. For example, in this case, instead of replacing reporters, the output of the software could be used as a starting draft by reporters who would then make edits to address the “Pleasant to Read” flaw readers found in the study you mentioned in your post. This could potentially lead to juicier content for readers.
Algorithms like those embedded in Narrative Science (NS) will only exacerbate the death spiral facing the business model of newspapers today. News providers like AP or Reuters are already providing a baseline for pure information-based news reporting. Listicles, Buzzfeed, and other click-bait articles might as well be written by computers – I am not sure anyone would care. I would imagine that these are the jobs NS will have the potential to replace.
However, the higher quality form of journalism that involves on-the-ground reporting or investigative journalism will probably never be replaced by data-crunching algorithms because, by definition, they require digging in for new information and alternative theories that cannot be extracted from databases. The real challenge is to convince users to pay to access high quality online content (NYT, Wall Street Journal, etc) that requires significant resources to produce.
Interesting post Nik. I really like the idea of using existing technology like IBM Watson to improve the database from which technology like this would undoubtedly need to rely on. I wonder how people would react to this technology though – would we automatically expect amazing results simply because we know the vast amount of data the software has access to? And will we be let down when a software written blog post fails to invoke the same emotion as a talented writer?
This was a very well written and informative article. Thinking about where this technology will be in 20-30 years is absolutely scary. I envision a world where there is no need to write a broad majority of simple news articles, but this is in a “downside” scenario. If this technology continues to accelerate there is the potential to eliminate millions of research and journalism jobs. I actually envision this technology service as the mechanism to take the data collected by AI / Machine learning and synthesizing it into an article or a paper that is easily consumable for humans. While this appears, at its current stage, to be a technology focused on generating simple articles I actually see the potential for this technology moving way beyond the journalism industry.
The machines are indeed coming. I get that it is difficult for people to imagine how machines could produce things that are so dependent on nuanced semantic understandings, but they are. Recently, there was the first ever AI-produced music video… https://www.youtube.com/watch?v=023PJjBIbkM Admittedly, it was terrible, but it exemplifies where we are going.
It is entirely reasonable to imagine that the vast majority of content creation will come from machines in the future. With satellite and drone technology already able to give use on-demand access that any corner of the earth, why cant machines take the data generated by this technology, apply voiceovers based on how the machine interprets what is going on, add filters etc. and release the content as CNN would do?
There is a theorem that shows that with enough time, a monkey could have written a Shakespeare play(1). It is therefore utterly plausible that an algorithm could have written the above post with less than infinite time.
(1) https://en.wikipedia.org/wiki/Infinite_monkey_theorem
Thanks for the great post, Nik. I agree with what many here have added. The future of machine learning (predictive and adaptive output based on raw input) is going to be huge. I believe there are plenty of great synergistic areas to put natural language programs to use in journalism and media and news. I do not foresee a near future where programs like Quill will be taking over however (maybe for lesses publications). Big news outlets have high quality and sourcing standards. A human would need to review these things before pushing to print the article. Also opinions are often important in the news these days, as is a more flowing and compelling use of language. A computer CAN do all this. The issue is that the program would need to be hard-coded to write in such a way, based on the data input, which you do discuss. What’s not spelled out is how much time and energy it takes to build even a single algorithm for machine learning output. Over time a company might be able to build algorithms for weather articles, business articles, film articles, etc etc etc but this would require a great deal of upfront work to really get it right and get a way to adapt language naturally for each article. They would also need a more naturally sourced and less structured data input method to be developed for long-term sustainability. It is certainly possible, but we are talking about very, very hard stuff here. If there resources required would always take more than a few humans, why not just hire humans? Autogenerating cheap news can easily be replaced. Good news, poetry, novels, etc would need to be much more heavily scrutinized from a cost-benefit perspective.