How Machine Learning is Changing the Way People Explore the World at Airbnb
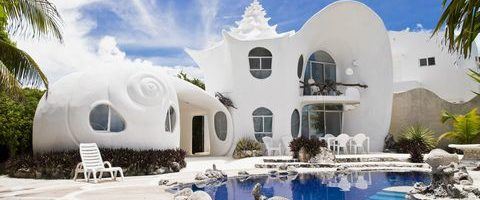
Guests have checked into Airbnb properties 300 million+ times, in 4.5 million locations across 81,000 cities, and the company collects more than 15 petabytes of data each day. How can Airbnb leverage this data to change the way we travel and experience new places?
The Sharing Economy
Over the past decade, consumers have seen an explosion in the sharing economy, and the companies driving this shift have become some of the largest and most innovative in the world. Uber and Lyft are changing the way we commute, creating a marketplace that matches idle drivers to consumer demand. Door Dash and Task Rabbit are doing the same for deliveries and household tasks. One of the largest beneficiaries of this economic shift has been the peer-to-peer rental market, dominated by Airbnb, which is rapidly taking customers away from more traditional lodging alternatives such as hotels and other vacation rentals (see Exhibit 1)[1]. As these markets have exploded, so too has competition, and market leaders are leaning on technical innovation to deliver the best possible experience to their constituents in these two-sided marketplaces and beat the competition.
Exhibit 1
Machine Learning at Airbnb
Airbnb began 10 years ago when its co-founders inflated 3 airbeds in their San Francisco apartment; since then guests have checked into Airbnb properties 300 million+ times, in 4.5 million locations across 81,000 cities, and the company is now worth more than $30 billion[2]. As usage has exploded, so too has Airbnb’s data collection (over 15 billion events and 15 petabytes of data each day[3]), and the company is using this data with machine learning (“ML”) algorithms to enhance the experience for both hosts and guests.
For guests, the benefits of these algorithms materialize primarily in their search experience. Airbnb can track how consumers interact with the site based on what they search, how much time they spend looking at a given property, and even the décor of the properties they like! The algorithms then comb through both structured (i.e. room type) and unstructured (i.e. reviews, listing descriptions) data to predict which accommodations guests are most likely to book and even which reviews they are most likely to find helpful. Furthermore, lower rank will be attributed to properties where the host is more likely to reject a request (due to guest rating, pets, etc.) In the future, Airbnb hopes to continue to expand the number of input tags into to these algorithms (i.e. adding different rental/experience types)[4]. Additionally, the company has started rolling out Airbnb “Experiences,” which will allow Airbnb to collect data not only on where its customers are traveling, but what they like to do in those locations!
For hosts, the largest benefit comes from Airbnb’s ability to help forecast demand and aid in pricing their listings. Hosts have access to information such as how many users are looking for their kind of home in that given area, how comparable listings are priced, and probability of getting a booking at a given price[5],[6]. This algorithm can even price listings automatically based on competing listings and guest search demand and can adjust prices accordingly on the fly.
What’s next?
As Airbnb continues to collect more data from its hosts and consumers, I believe there is an opportunity to shift the business model from lodging to a complete travel experience. The company is already testing the water with their Experiences concept; going forward, Airbnb should be leveraging ML not only to offer the best possible home for a weekend getaway, but a variety of experiences in that location specifically catered to that guest’s interests. In the near term, Airbnb should be focusing significant resources in understanding how consumers experience the places they travel, further building out and marketing the Experience concept. Longer term, I can envision a full concierge-type service (likely implemented with AI bots) that can offer guests both lodging and activities recommendations in the location of their choice based on individualized preferences!
Key Question to Consider
While ML algorithms are useful in enhancing the Airbnb peer-to-peer rental economy, there can be unintended consequences of predictive algorithms that are not always positive. In December 2016, Harvard University researchers released a working paper that concluded it was harder for consumers with African American sounding names to rent through the site (16% less likely to be accepted relative to identical guests with distinctively white names)[7],[8]. This problem was potentially exacerbated by Airbnb’s ML, which may not show listings from hosts likely to reject a given guest, leading to a more limited offering set for those who may have been rejected in the past due to discrimination. A key question to consider: Is it possible to leverage AI to reduce or limit discriminatory behavior in the shared economy?[9]
(755 words)
[1] “Machine Learning is Driving Growth at Airbnb,” Business Insider, June 16, 2017 [https://www.businessinsider.com/machine-learning-is-driving-growth-at-airbnb-2017-6]
[2] “How AI is Powering Airbnb’s Mission to Change How We Travel Forever,” London Evening Standard, November 9, 2018, Factiva
[3] “Airbnb Uses Big Data and Machine Learning to Enhance User Experience,” Computerworld Hong Kong, June 1, 2017, ProQuest
[4] “Airbnb Unveils Roadmap to Bring Magical Travel to Everyone,” PR Newswire, February 22, 2018, Factiva
[5] “Search Ranking And Personalization at Airbnb,” Proceedings of the Eleventh ACM Conference on Recommender Systems, Mihajlo Grbovic, Airbnb, August 2017
[6] “Airbnb Machine Learning – How Data and Social Science Make it All Work,” TechEmergence, April 13, 2018 [https://www.techemergence.com/airbnb-machine-learning-how-data-and-social-science-make-it-all-work/]
[7] “Racial Discrimination in the Sharing Economy: Evidence from a Field Experiment,”American Economic Journal: Applied Economics, December 16, 2016
[8] “Airbnb Adopts Rules to Fight Discrimination by its Hosts,” New York Times, September 8, 2016 [https://www.nytimes.com/2016/09/09/technology/airbnb-anti-discrimination-rules.html?_r=0]
[9] “A survey on measuring indirect discrimination in machine learning,” ACM Journal, October 2015
The unintended consequences are interesting – human bias can cloud the AI functionality significantly. Solving the discrimination factor correctly and quickly is crucial. To add to the question you posed at the end, I wonder if AI is limiting the exploratory process that some travelers enjoy going through by pushing options based on past trends? If for example a user was vacationing in a country on another continent, would his options only throw up results based on his previous travel choices which are likely skewed because they may have been business trips or trips in his own country?
Further, if houses which have good past ratings and views are more likely to be pushed to the users, will it create an imbalance in the process where houses that tend to be occupied more will continue to be occupied more as it is favoured by AI? At the moment, I feel that Air BnB should use AI in its search engine but complement it with some human intervention to oversee some of these anomalies that may arise. Over time as this normalizes, it could run on its own and then I agree with your hypothesis, that it will become a full concierge-type service.
One reason I find AirBnB’s algorithm to be so problematic is that it is surfacing different information to users on the basis of guest rating — thereby making it more challenging for individuals with low ratings to find accommodations that fulfill their criteria. Given that guest rating could be influenced by a multitude of factors — including any implicit or explicitly held host biases — it at first glance seems irresponsible to incorporate guest ratings into the algorithm. Uber, for example, doesn’t surface a rider’s name or guest rating to a driver until after the driver accepts the trip (and never displays a photograph), consequently reducing the likelihood that the driver will reject the rider on the basis of gender or race. While I recognize that AirBnB believes its host are entitled to additional precautions because they are letting strangers into their homes, I also believe that certain standards need to be in place to reduce the likelihood of discrimination.
While I do think it is possible to leverage AI to reduce or limit discriminatory behavior in the shared economy, I think technology companies will need to approach this issue very thoughtfully. They will need to creative find ways to reduce any inherent bias in each of the inputs to the algorithm. I think there are a couple of ways AirBnb could approach this. They could either remove any inputs that have a subjective component, or, more controversially, they could explicitly ask guests their race, and then calibrate the host’s ratings based on any detected discriminatory past behavior. This wouldn’t address the issues AirBnb has observed in the past with hosts rejecting guests on the basis of race, but it could help reduce the influence of racism on the search algorithm.
Super interesting article. I love what Airbnb is doing with machine learning. As a consumer too many options is overwhelming and if they can filter down to the 4-5 I’m most likely to select than it significantly improves my experience. One hesitation I have is with how accurate the machine learning actually is. When booking an Airbnb I tend to have different criteria every time depending on who I’m traveling with, if its for work or personal, or where the destination is. If the machine learning systems can’t pick up these differences I worry that it would be keeping certain options from me just because I haven’t selected something similar in the past. It will be interesting to see how Airbnb solves this problem.
To answer your question I think AI and machine learning will be excellent tools to reduce discrimination in the industry. The discrimination found still stems from human users and while it may have been exacerbated by the ML, I think that’s something that can be easily solved for once it is known. Even in other instances where ML has been found to be discriminatory, it still tends to fare better than when humans are left to their own devices. I think Airbnb can actually use ML programs to find hosts who are guilty of this behavior and remove them from the site. Additionally, as the programs get more advanced, AI will be able to find and book places independently, completely removing any identifying characteristics from the process (e.g. race, gender)