Google and Gmail: Machine Learning for Defense, Not Disruption

How Google is using Machine Learning to keep Gmail (and other core products) ahead of the rest.
Introduction
I, like many around the world, use Google’s email product, Gmail, on a near-daily basis. In fact, Gmail was the world’s most-used multi-platform (e.g., mobile and desktop) email client by traffic in 2017 [i]. Given this apparent success, it may surprise you to learn that according to Gmail’s Product Manager, Gmail just underwent “a ground-up rewrite” in 2018 aiming to “use Google’s AI to make Gmail smarter.”[ii]
Megatrend of focus: Machine Learning
This recent reboot raises the question: why rework the most popular email client on earth? The answer has to do with Google’s commitment to using Machine Learning to make process improvements in its suite of products. As far back as 2014, Google’s early interest in Machine Learning, prompted a former employee to assert that “Google is not really a search company. It’s a machine-learning company.”[iii]
At the time, however, this focus was driving the innovation in some of Google’s more tangent businesses (e.g., autonomous vehicles), but has quickly spilled over into Google’s core. Google has recognized that, as a company whose reputation and competitive advantage rests on its expertise in algorithmic computation, Machine Learning is the next frontier for process improvements. Rather than allowing its markets to be disrupted by Machine Learning-driven competitors, Google has recognized the trend toward Machine Learning-enabled products, and chosen to defend its market position by investing and innovating first.
Google has been making investments in Machine Learning across its products, from Cloud to Advertising[iv], and the recent updates to Gmail are simply the latest manifestation of this commitment, presenting themselves to the consumer in time-saving features like Smart Reply[v] and Smart Compose[vi].

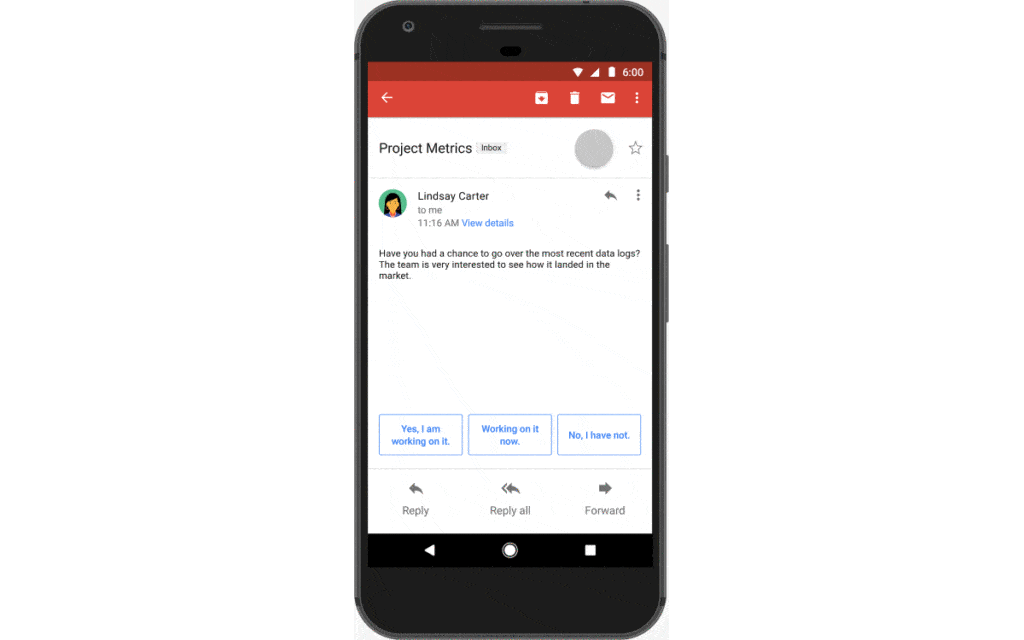
Organizational action for short and medium term
As Google looks forward in the short term, it appears a large part of its Machine Learning-based advantage is expected to come via acquisition. Large early investments like its $500M acquisition of DeepMind opened the door for a slew of AI and Machine Learning acquisitions, up to a total of 14 as of May 2018.[vii]
Again, many of these acquisitions mean Google has added features to its core products which will protect the market leadership positions of those products. Google has a well-defined core it knows it must defend, and “through machine learning, Alphabet [Google’s parent company] can better solidify its dominance in search and advertising.”[viii]
In terms of developing its Machine Learning capabilities in the longer term, Google has begun pursuing two longer-horizon channels: R&D and venture capital investment. In terms of R&D, Google has been outspending the majority of tech behemoths, with a differential focus on spending in Machine Learning, evidenced by its recent leadership in AI patents.[ix]
On the venture capital side, Google has launched two AI-only investment funds, Gradient Ventures and Google Assistant Investment Program. The name of this second fund tells the tale; its charge is to invest in Machine Learning technologies which can enhance the quality of the Google Assistant product[x]. Once again, investment is being made in Machine Learning in order to defend and grow one of Google’s most important products, one already in a leadership position in terms of quality[xi].
Recommendations
What Google must continue to pursue as it invests in Machine Learning to generate process improvements are adjustments which add value to the users of the product first, and to the strength of its advertising algorithm second. While its revenue is dependent upon success in an increasingly competitive market for online advertising[xii], the strength of its business relies on the ability of its core products to attract users and drive engagement.
For Gmail specifically, Google should continue its pursuit of product improvements which make sifting through email less of a chore, such as filtering out promotions and spam, additional improvements to Smart Reply and Smart Compose, or drawing users attention to messages he or she will likely want to prioritize. Machine Learning should continue to be helpful across these dimensions.
Outlook: open question
The most important open question for Google in its investment in and application of Machine Learning is: How can it ensure that the capabilities it builds are sufficiently transferable across products to justify the investment?
As we reflect on IBM Watson, we see an example of an organization and technology which requires meaningful rework and additional investment with each new application[xiii]; this appears to be a significant and potentially expensive risk of these investments.
Word count: 730
[i] Litmus: Market Share of the most used email clients as of December 2017, via Statista, accessed November 2018
[ii] Anthony Carcz, “Google’s New Gmail Is the Best Thing To Happen To Email Since the Old Gmail,” Forbes, April 25, 2018, https://www.forbes.com/sites/anthonykarcz/2018/04/25/googles-new-gmail-is-the-best-thing-to-happen-to-email-since-the-old-gmail/#7510cd854477, accessed November 2018
[iii] Robert McMillan, “Inside the Artificial Brain That’s Remaking the Google Empire,” Wired, July 16, 2014, https://www.wired.com/2014/07/google-brain/, accessed November 2018
[iv] “Google Strategy Teardown: Google Is Turning Itself Into An AI Company As It Seeks To Win New Markets Like Cloud And Transportation,” CB Insights (August 2018), CB Insights, https://app-cbinsights-com.prd2.ezproxy-prod.hbs.edu/research/report/google-strategy-teardown#ai
[v] Google, “Save time with Smart Reply in Gmail,” https://blog.google/products/gmail/save-time-with-smart-reply-in-gmail/, accessed November 2018
[vi] Google, “SUBJECT: Write emails faster with Smart Compose in Gmail,” https://www.blog.google/products/gmail/subject-write-emails-faster-smart-compose-gmail/?_ga=2.67287953.1073448467.1542036234-1210834642.1542036234, accessed November 2018
[vii] “The Race For AI: Google, Intel, Apple In A Rush To Grab Artificial Intelligence Startups,” CB Insights (February 2018), CB Insights, https://www.cbinsights.com/research/top-acquirers-ai-startups-ma-timeline/
[viii] “Google Strategy Teardown: Google Is Turning Itself Into An AI Company As It Seeks To Win New Markets Like Cloud And Transportation,” CB Insights (August 2018), CB Insights, https://app-cbinsights-com.prd2.ezproxy-prod.hbs.edu/research/report/google-strategy-teardown#ai
[ix] Ibid.
[x] Ibid.
[xi] John Koetsier, “AI Assistants Ranked: Google’s Smartest, Alexa’s Catching Up, Cortana Surprises, Siri Falls Behind,” Forbes, April 24, 2018, https://www.forbes.com/sites/johnkoetsier/2018/04/24/ai-assistants-ranked-googles-smartest-alexas-catching-up-cortana-surprises-siri-falls-behind/#7be60c29492a, accessed November 2018
[xii] EMarketer Editors, “Data Suggests Surprising Shift: Duopoly Not All-Powerful,” eMarketer Pro (March 2018), eMarketer, https://content-na1.emarketer.com/google-and-facebook-s-digital-dominance-fading-as-rivals-share-grows
[xiii] Willy Shih, “Data Suggests Surprising Shift: Duopoly Not All-Powerful,” HBS No. 9-616-025 (Boston: Harvard Business School Publishing, 2016)
Thanks for this interesting take on an established company recognizing the potential disruption from Machine Learning and making that a core focus to maintain its competitive advantage. One thing that strikes me as part of Google and Alphabet’s competitive advantage is their ability to be right more often than not when it comes to investing in new opportunities or through M&A with promising up and coming companies. With their uptick in M&A with a focus on ML and AI many of these investments seem to have resulting in value add for their core and even tangential products and services. While I grasp the importance of ML in google’s context and also the continued importance of machine learning to continuously iterate and improve its processes and products, I wonder how significantly its strategy and investment might shift as new disruptive trends arise. Will google truly continue to be a machine learning company or is this merely a titanic company’s response to emerging trends and competitive threats?
Thoughtful piece on how one of the technology industry’s “poster-adults” Google is aggressively pushing to maintain its dominance in email services. We often associate a strong core as not requiring additional investments to fend off competition – but Google has taken the approach of embracing ML in order to avoid being disrupted. I appreciated the author’s acknowledgment that Google’s strong balance sheet also allows it to simply acquire the technologies it needs, so I question how much of an innovator the company is, versus its ability to assert its dominance through inorganic growth which it can execute virtually at will? In answering the question of transferability, I would argue that the ease of transferring to other areas of its business is almost irrelevant for the tail of Google’s smaller experiments which would have a marginal improvement in its overall revenue growth. Google’s revenue predominantly comes from its search business (https://qz.com/1334369/alphabet-q2-2018-earnings-google-is-more-than-just-advertising-now/) so can the business simply position itself as a cash-rich company that simply brings technologies in-house whenever it so desires?
Insightful analysis of Google’s strategic shift to becoming an AI-first company. I completely agree with you in recognizing the motivation for Google to re-design one of its most popular products, Gmail, is to prevent major disruption from happening from a smaller, more agile startup that realizes the value ML has to impact existing industries. I very much appreciated the broad approach you took looking at how Google on a company-wide perspective also is looking to infuse machine learning in the rest of the company.
You spend some time discussing the new machine learning enabled features in GMail. It would be interesting to hear more about how Google will evaluate the performance of these features, given that there is most likely a learning period where the features are not giving the most accurate predictions and detract from the user experience.
Finally, I would be curious to hear Google’s perspective regarding your open question of the transferrability of the technologies used in gmail. Personally, I imagine that since Gmail is one of the few billion user products at Google, they would actually be fine investing in technologies that just cater to this large customer base.
I’m not surprised to learn that Google is heavily investing in machine learning and AI. I believe that no other company is as well positioned as Google to develop predictive algorithms. In fact, I think that Google is at a tremendous advantage because of its existing access to data. I’d be curious to learn if Google records the content of personal emails and messages, and using that text to inform its algorithm. It likely does. Additionally, Google can integrates learnings from Google searches with Gmail, using Google searches to enhance its understanding of how people think and write. Integrating its existing data with expertise and code from its acquisitions should be a formula for success.