From the #1 CRM to the smartest CRM: How Salesforce is bringing machine learning to the world of sales

Salesforce is changing the game of sales again with its predictive intelligence system “Einstein” that helps to boost the efficiency and efficacy of sales representatives. Learn how the company is innovating on this front and applying machine learning solutions to a business problem that is innately human and relationship-driven.
When Salesforce first launched in 1999, it fundamentally transformed the tech industry by pioneering the software as a service model. As its name suggests, however, Salesforce is not simply an enterprise software company. It is also involved in the business of sales since it disrupted the traditional model of how businesses thought about sales processes with its groundbreaking customer relationship management (CRM) product. As a company that caters primarily to salespeople, Salesforce’s own sales team is extremely strong, and its unique and effective way of selling has contributed significantly to the organization’s success [1]. In fact, Salesforce is world-renowned for its sales leadership – its annual Dreamforce conference attracts hundreds of thousands of the best minds in sales who flock to discuss best practices and learn how to “sell the Salesforce way” [2].
Unsurprisingly, marketing and sales expenses represent the company’s largest costs, and the company’s sales headcount is increasing at greater than 20% per year as Salesforce continues to invest in growing its sales capabilities [3]. In addition to investing in expanding its sales teams, Salesforce is also leveraging machine learning to develop innovative solutions aimed at improving sales productivity. One such solution, Einstein for Sales Cloud, is a predictive intelligence system that helps boost the efficiency and efficacy of sales representatives utilizing the product through [4]:
- Scoring and predicting which leads and opportunities are most likely to convert so sales representatives can prioritize those opportunities (Exhibit 1)
- Identifying opportunities that are “at risk” and require immediate attention
- Surfacing key business development insights of accounts by scanning external news sources in order to help sales representatives increase win rates (Exhibit 2)
- Improving forecasting accuracy and refining forecast drivers
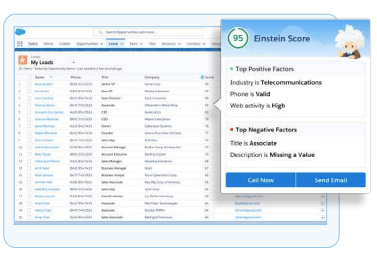

To support the development of additional machine learning initiatives, Salesforce has acquired several AI startups and has amassed a talented pool of machine learning experts to help improve Einstein’s existing functionality and add new features in the future [5]. Salesforce’s large sales team and immense repository of customer data provide an optimal environment to experiment and train the models underpinning its machine learning systems. The company understands that sales is a highly dynamic environment and has implemented measures to ensure that its models are updated frequently. For instance, forecast models are rebuilt each month, and forecasts are recalculated approximately every six hours [4]. Opportunity scoring models are updated every ten days and opportunities are scored every four hours using the latest model to ensure the most up to date predictions [4]. In addition to its internal use at Salesforce, Einstein has also been made available for customers to purchase. In the medium term, Salesforce founder and CEO Marc Benioff has articulated his vision that artificial intelligence will be the next wave of transformation for the CRM landscape [5]. The company envisions this solution expanding to all parts of the customer lifecycle funnel, including after-sales services, and sees Einstein integrated throughout the organization as it is used to power most of Salesforce products and functions.
At its core, sales is still an innately human and relationship-driven function. It will likely take time and significant behavioral changes for machine learning applications in the sales world to become widely adopted and seen as mainstream. It is thus important for Salesforce to continue gathering feedback from sales employees on which applications work well and which are counterproductive in the context of their daily lives. Such machine learning solutions can also be complicated and hard to understand. Salesforce has done well to make these tools simple and user-friendly. For instance, Einstein very clearly displays intuitive factors behind the model’s predictive scores for leads (Exhibit 3). Continuous training has to be provided to ensure that sales representatives trust the technology and know how to effectively leverage the tools provided to them. Ultimately, statistical methods lack the intuition and domain knowledge of human analysis, so steps also need to be taken to balance human-machine interaction in order to obtain optimal sales results [6].

(771 words)
References:
[1] Whitford, David. “Salesforce.com: The Software and the Story.” Inc., September, 2014. https://www.inc.com/magazine/201409/david-whitford/inc.500-sales-force-boot-camp-produces-modern-salesman.html, accessed November 2018.
[2] “The tower of Benioff.” The Economist, January 4, 2018. https://www.economist.com/business/2018/01/04/masterful-salesmanship-has-pushed-salesforce-to-ever-greater-heights, accessed November 2018.
[3] Salesforce. 2018 Annual Report. https://s1.q4cdn.com/454432842/files/doc_financials/2018/Salesforce-FY18-Annual-Report.pdf, accessed November 2018.
[4] Salesforce. Sales Cloud Einstein Data Sheet. https://c1.sfdcstatic.com/content/dam/web/en_us/www/documents/datasheets/Sales-Cloud-Einstein-Datasheets.pdf, accessed November 2018.
[5] “Nonstop Benioff: Inside The Master Networker’s Audacious Plan To Disrupt Salesforce — And The World.” Forbes, September 13, 2016, https://www.forbes.com/sites/alexkonrad/2016/08/24/nonstop-benioff-inside-the-audacious-plan-to-disrupt-salesforce-and-the-world/#4c5abf6a11d6, accessed November 2018.
[6] Fedyk, Anastassia. “How to tell if machine learning can solve your business problem.” Harvard Business Review. https://hbr.org/2016/11/how-to-tell-if-machine-learning-can-solve-your-business-problem, accessed November 2018.
The deployment of AI among CRM products has been a hot topic lately. Many have boasted their capability of identify, defect and surface hidden patten that are unobserved by humans. From your article, you’ve done a terrific job detailing the functionality of Einstein and how it adds value to Salesforce’s CRM products compared to its competitors. I found the idea that “Einstein surfaces relevant insights about an account based on sources such as external news websites ” to be a particularly interesting feature. It’s not necessarily AI-oriented, but definitely bringing value to customer through untapped resources. What I wish you could have included is the scoring mechanism that powers the predictive analytics, the engine of Einstein. I think by giving a specific example of how a deal is scored would be helpful to readers who are not familiar with the product and technology engine beind it.
This is an interesting insight – thanks for sharing! I agree with you that Einstein’ scope may be limited given the human engagement needed in a sales transaction. But I think that’s probably not the intent of Einstein. Einstein is/will be a great complement to the sales team’s efforts so they can prioritize their tasks and focus on strategic initiatives in the long run. Another limitation with Einstein is how it identifies “risks”. As markets change, risks will be “new” and “unknown” — such a situations make human involvement important.
One thing I would like to understand is what’s Einstein data source – is it primarily data input by the sales team or is data from the clients/ customers also incorporated?