Foxconn: Large Scale Manufacturing in the Machine Learning Age
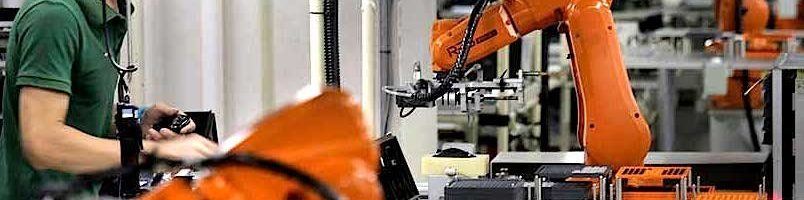
As Foxconn, the largest contract manufacturer in the world, faces increasing profit pressures, can it leverage machine learning to turn its business around?
Throughout the last decade, the field of machine learning has witnessed achievements such as Watson, AlphaGo Zero and Dota 2, widely covered breakthroughs that raised both awareness and expectations for machine learning applications. As of today however, effectively all commercial machine learning algorithms are still performing very narrow tasks only, leveraging ever improving computer vision and voice capabilities to process large amounts of data and solve a specific set of problems including prediction, optimization and anomaly detection[1].
While all industries are likely to be impacted by machine learning, the manufacturing industry seems especially ripe for disruption. Machine learning’s current narrow abilities can already meaningfully improve manufacturing processes: Accurate prediction of customer demand drives down inventory holding costs; optimization of process flows improves cycle times and output rates; and early anomaly detection prevents costly factory shutdowns. For Foxconn, the largest contract manufacturer in the world, these potential process improvements could come at a critical time. As the main supplier of Apple iPhones, Foxconn’s recent profits have suffered as iPhone sales have plateaued[2]. Additionally, production facilities in China increasingly face labor cost pressures as more young people shun factory work[3]. With already thin margins at risk of eroding further, Foxconn Chairman Terry Gou announced in early 2018 that the Company’s goal is to become “a global innovative AI platform rather than just a manufacturing company”[4].
To turbo charge this pursuit in the short term, Foxconn partnered with Landing.AI[5], an organization led by AI pioneer Andrew Ng that aims to transform enterprises with machine learning solutions. One of the first manufacturing steps Landing.AI is looking to tackle is quality control. Instead of humans visually inspecting each manufactured component manually, Landing.AI has developed a classification algorithm that detects errors more quickly and accurately than humans[6]. Foxconn is also looking to advance academic research focused specifically on the industrial space and has signed a cooperative research agreement with the University of Cincinnati for that purpose. In the medium to long term, the Company recognizes that external expertise alone will not suffice. Hence, it committed to invest ~$350m into AI R&D over the next five years and set up a new company called Industrial AI System in Silicon Valley, with the objective to recruit 100+ engineers and deep learning experts in Silicon Valley alone[7].
While these initial steps are promising, going forward, Foxconn needs to keep several challenges in mind as it looks to execute on its machine learning strategy. Firstly, Foxconn currently employs more than 800,000 people[8], the vast majority of which will not be familiar with machine learning. As a 2018 working paper by HBS professor Prithwiraj Choudhury highlights, a skilled operator is key to maximizing the impact of machine learning applications[9], which makes the immediate retraining of its current workforce a priority for Foxconn. That being said, a potentially large portion of Foxconn’s current workforce is at risk of becoming obsolete as machine learning takes over tasks previously performed by humans. With regards to these individuals, Foxconn has the duty to be transparent and proactive in its communication to make any impending transition as painless as possible, rather than reciting cryptic rhetoric that is often used nowadays when companies are confronted with the threat of machine induced job losses. Secondly, Foxconn will need to rethink its organizational structure. Should there be a separate machine learning vertical that works in collaboration with other divisions at the Company? Or should machine learning experts be integrated within the individual divisions? Lastly, looking at Foxconn’s long-term strategy, it needs to resist the temptation to view machine learning as the magic wand that fixes all challenges, and rather consider carefully which problems machine learning can address. For instance, while machine learning will likely increase manufacturing efficiency, it is unlikely to address the top line pressures from stagnating iPhone sales, a critical challenge that could require the Company to reassess its strategic priorities.
To conclude, while machine learning’s capabilities today certainly have the potential to markedly improve Foxconn’s manufacturing processes and bottom line, implementing machine learning goes far beyond simply applying a new technology solution, but rather entails a series of different challenges that will ultimately determine the success of Foxconn’s machine learning ambitions.
(713 words)
[1] M. Chui, J. Manyika, M. Miremadi, N. Henke, R. Chung, P. Nel, S. Malhotra. Notes from the AI Frontier: Insights From Hundreds of Use Cases. McKinsey Global Institute (April 2018)
[2] J. Condliffe. Foxconn Is Feeling the Sag in Smartphone Sales. Apple? Not So Much. New York Times (August 2018)
[3] B. Bland. Young Chinese shunning factory jobs, says Foxconn founder. Financial Times (October 2013)
[4] N. Lomas. Foxconn to plug at least $340M into AI R&D over five years. TechCrunch (2018)
[5] R. Metz. Andrew Ng Says Factories Are AI’s Next Frontier. MIT Technology Review (December 2017)
[6] A. Ng. Landing.ai – Transforming manufacturing through AI. Landing.AI (December 2017)
[7] L. Li. Foxconn’s Terry Gou pushes ‘industrial AI’ in Silicon Valley. Nikkei Asian Review (July 2018)
[8] Foxconn 2017 Annual Report
[9] P. Choudhruy, E. Starr, R. Agarwal. Machine Learning and Human Capital: Experimental Evidence on Productivity Complementarities. (February 2018)
C. Xu – Thank you for the thoughtful essay. It was interesting to read how machine learning can be adopted into the manufacturing industry. I think you raise a valid point around the need to have skilled operators who understand how machine learning works and can apply it to the solve the challenges an organization faces. Lack of skilled workers may work as a hindrance against wide usage of machine learning not just in the manufacturing industry but in any other industries where machine learning can be used. To avoid this issue, we should try to have more programs dedicated to teaching people about machine learning.
It was also surprising to know that Foxconn will be investing ~$350m into AI R&D over the next few years. Even for Foxconn, this is not a small investment and it would be interesting to see how the investment goes. At the end of the investment, Foxconn may prove to be a pioneer in machine learning in the manufacturing space. Or, in the worst case, it may prove that the manufacturing industry is not “ripe for machine learning disruption.”
Thanks for the perspective, Cong. I think you raise good points on the workforce-related challenges of Foxconn making such a radical change to its business model. I would anticipate that it will be easier to deal with the need for specialised technicians than it will be to handle the negative repercussions of potentially significant workforce reductions, at least in the short term. It’s far more attractive to be a worker being asked to upskill, or a new hire brought onboard to build-out new capabilities, than it is to be the worker being let go. I’m interested in your thoughts on how you think a nation’s social safety net and industrial relations architecture will affect the character of its adoption of disruptive technologies such as machine learning. I can easily envisage the strong role of unions and labour bodies in nations such as Germany standing as an impediment to the rapid adoption of these kinds of advancements.
Cong, this was a great read and a topic that should be highly relevant to business leaders and policy makers alike as machine learning and automation becomes an ever important part of large scale manufacturing processes. To address the question you posed at the end of your article, where should machine learning experts be placed within FoxConn as an organisation? I suspect it makes the most sense to integrate these machine learning experts into each existing division directly, as opposed to one centralized machine learning division. I believe that direct integration of machine learning experts will go a long way towards increasing the level of engagement needed between line workers and machine learning experts and developers which in turn can help reduce the understandable anxiety held by line workers towards automation, and also accelerate product development of machine learning products as line workers themselves can provide insights on manufacturing processes.