Flotek – Drilling Into The Fracking Data With Machine Learning?
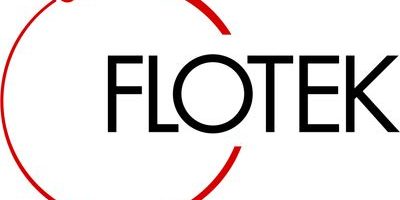
The management team of Flotek is facing pressure from public market short-sellers and using fracking data to build a new machine learning tool that helps differentiate its specialty chemicals product in the marketplace.
Introduction to Flotek
Flotek is a well-established company based in the United States (“U.S.”) and focused on designing and supplying specialty chemicals to oil & gas operators. Flotek’s primary driver of profitability is a suite of chemical products called complex nano-fluids (“CnF”). CnF is comprised of high-value compounds that are used in hydraulic fracturing to enhance oil and gas production. Flotek marketed CnF among operators by promoting an oil & gas production uplift of 30% [1]. In order to fund the expansion of CnF product offerings, Flotek decided to go public in 2007 [2].
The specialty chemicals industry in the U.S. was highly commoditized and there was little differentiation between specialty chemicals used in hydraulic fracturing. As the fracking boom began to unfold in the U.S., the players in the marketplace faced a much higher level of competition. Moreover, Flotek experienced sales decline, profit margin degradation, and share price decline. A group on hedge funds on Wall Street, led by FordWorld Capital Management, decided to short-sell the stock and publish reports criticizing Flotek’s CnF product in the public investor community. The short-sellers analyzed publicly available data and concluded that revenues would fall 20-30% in the near-term [3], contributing to a market capitalization drop of over 50% [4]. Flotek’s management team reacted to the share price drop by redefining the value proposition of the CnF product. John Chisholm, CEO of Flotek, decided to pivot CnF product development strategy from a pure-play chemical product to a technology-driven product. Given the rise in real-time data collection in the oil & gas industry and the decrease in computer processing power cost, Flotek identified machine learning (“ML”) as a potential tool to improve CnF and provide a value-add solution to its customers [5].
Flotek’s Machine Learning Strategy
Flotek’s management team understood that the public markets demanded a change in the company’s product development strategy and a higher level of differentiation in the CnF product offering. To drive innovation in the short-term, Flotek opened a new Global Research & Innovation Center and partnered with oil & gas thought-leaders and researchers to incorporate ML into Flotek’s CnF product offerings [6]. The research & development team used drilling equipment to collect real-time data from oil & gas wells in specific geological formations. The localized data was analyzed by the researchers through the ML tool to understand the relationship between CnF’s chemical mixture and oil & gas well production rates. This allowed Flotek to design a customized combination of high-value chemical compounds and maximize oil & gas well production rates in various geological formations [7]. Operators reacted quite favorably after using the new CnF product given the increase in oil & gas well production rates and yield of existing oil & gas resources. To further develop the state-of-the-art ML technology and create a long-term competitive advantage, Flotek decided to independently work with IBM [8]. By evaluating the existing in-house ML tool and IBM’s Watson capabilities, the researchers were able to fine-tune its customized CnF product and provide a differentiated value-add solution to oil & gas operators. Flotek was able to successfully take advantage of the ML mega-trend in the oil and gas industry to transform and differentiate its CnF product.
Recommendations
While the ML tool seems to enable Flotek to provide a differentiated product in the oil & gas sector, the incorporation of this tool faces a number of challenges that should be considered in the product development process. If Flotek aims to enhance its top-line growth, profitability, and stock price performance, then the management team should consider the following actions as part of its strategy:
First, Flotek should allow both the Global Institute of Research & Innovation Center and IBM Watson to combine their technologies and share the learnings associated with their product development process. This will allow the company to realize the synergies between the two technologies and optimize its CnF product development process.
Second, Flotek should provide free trails of its CnF product and ML tool to potential customers in the prolific geologic areas. The oil & gas industry has a high trialability cost and free samples will allow the company to capture large-scale operators in high-profile geologic areas.
Third, Flotek should promote its product suite of product internationally in order to scale is client base and increase the data set size. This would allow the company to have a much richer data set and enhance the ML tool’s effectiveness.
Open Questions
Will Flotek be able to scale its ML tool fast enough to maintain profitability and recover share price value? What other steps should Flotek take in order to propel innovation throughout its product development process?
(Words 773)
References:
[1] Flotek press release as of February 23, 2015.
[2] Capital IQ company data, accessed November 9, 2018.
[3] FourWorld Capital Management, “Drilling Down To Zero Report”, accessed November 9, 2018.
[4] Bloomberg market capitalization data, accessed November 9, 2018.
[5] Frost & Sullivan, “The Future of Drilling Systems Industry Research Analysis”, accessed November 10, 2018.
[6] Flotek annual report as of December 31, 2017.
[7] Capital IQ Flotek earnings call transcript for 4Q’17, accessed November 10, 2018.
[8] IBM, “Transforming Oil & Gas with IBM Watson Presentation”, accessed November 10, 2018.
Thank you for this very interesting article. Being in the oil and gas industry for 8 years now, I never knew that there was a company that could utilize machine learning to develop customized specialty chemical chemicals for fracking. That being said, I understand how difficult it is to scale a breakthrough technology in a relatively conservative industry. Based on my own experience in commercializing technologies, I would recommend Flotek to partner up with several large oil and gas companies that are well known in hydraulic fracturing. These partners would receive a discounted price for products. In return, Flotek would receive access to a larger number of fields, which would help them mature their technology faster. Furthermore, having a well known partner would help Flotek build brand equity and credibility in the industry, which would be beneficial for future sales prospects.
I am interested to learn about the application of ML to an industry whose operations are not in the digital/technology space. But of course this makes sense! I wonder what variables they are capturing about the CnF and what (at present) is the best-predicting variable of the production rate. Is it the structure of particular wells? Or the material they are ‘made of’? Or is it the chemical makeup of the oil itself? I would be curious to learn where their analysis started and where they ended up after recruiting ML to the problem? What other variables do you think they should consider incorporating into the ML to improve the predictive power of their model?