Enabling patients to get innovative treatments faster through machine learning at GSK
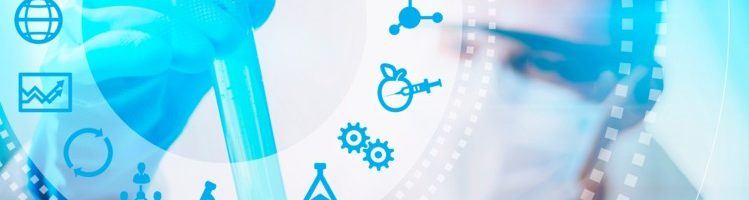
This article explains how GSK is using machine learning to increase R&D productivity and accelerating drug development timelines.
Healthcare today faces extraordinary challenges with aging population and increasing burden of chronic diseases in developed countries in addition to growing demand from the middle class in emerging countries. At the same time the pharma industry is facing a significant challenge in terms of R&D productivity[1]. WhileR&D expenditures have been going up, the number of drugs approved per year has been largely decreasing over the past 50 years.[2]
The challenge of R&D productivity at GSK
GSK, a global pharmaceutical company, is facing this very challenge. GSK spent GBP 3.9B in 2017[3]– close to 20% of their operating expenses – in R&D. Increasing productivity is thus critical in order to maximize value for shareholders and ultimately enable patients to get innovative treatments faster.
GSK led by its top R&D executive (John Baldoni) and CEO (Emma Walmsley) is probably the most active[4]of all pharma companies in applying artificial intelligence and machine learning (AI/ML) to the challenge of R&D productivity. Their journey started in 2013 with three core initiatives.
1 – Shared a bold vision
In 2013, Witty then CEO and Baldoni, seeing what happened in other industries, realized that machine learning would transform pharma. In 2014, Baldoni officially shared his bold vision and an ambitious target with the rest of the R&D organization: “Within 3 years, I want to go from a drug target to a molecule in 12 months. That takes 5 to 6 years now. That’s what success looks like.”[5] Following this, GSK reorganized itself with the creation of an in-house artificial intelligence unit. The first of its kind in the pharma industry.
2 – Leveraged partners to experiment
Not having the ML/AI capabilities in-house, Baldoni leveraged external partners to innovate and develop ML/AI applications. Baldoni’s team built partnerships with innovative startups – including Exscientia[6](announced in July 2017) and Insilico Medicine[7](Aug 2017) – to discover novel biological targets and pathways. GSK also worked with Google on applying AI to drug discovery. In July 2018, a paper in PLOS One described how researchers from the two firms developed a machine learning algorithm to identify protein crystals.
3- Structured GSK’s internal data
GSK also understood that data would be key to its future. The R&D department had close to 200 different databases. Answering any question required the scientists to search ~20 databases.Thus, GSK piloted consolidating data with Palantir’s help. They then created an internal R&D Data Centre of Excellence to bring additional data sources together. This resulted in a faster drug discovery process.
These three initiatives resulted in increased organizational focus and shorter development timelines. However, two other initiatives could further enhance current efforts both in the short-term (2018-20) and longer term (beyond 2020).
Looking ahead
1 – Strengthen the Culture of Experimentation
In the short-term, GSK should focus on changing their culture to encourage experimentation. Pharma is heavily regulated and requires large investments which historically has inhibited cultures of ‘trial and error’. GSK should take example on IDEO[8]with their unique culture and their three-step ideation process. Using prototypes and qualitative user research would help GSK develop ML/AI applications faster and better meet the needs of their researchers.
2 – Formalize the development process for ML applications through stage-gate
In the meantime, GSK should also develop a stage-gate process. Like Indigo[9], this would help better allocate resources and scale ideas in a more efficient way. Today, GSK is relying heavily on external partners for its development of ML/AI applications. This result in a costly development. The use of a stage-gate process could increase focus and enable the organization to develop machine learning applications in-house in the longer term.
Questions in suspense
To conclude ten years from now, drug discovery will be largely driven by machine learning. It will enable GSK to predict in silico a molecule’s likelihood of success thus lowering the costs of bringing a new drug to market. However, after 5 years of strategic focus on artificial intelligence a few questions remain open for GSK:
- How to attract, retain and develop the right talent (e.g., data scientists, cloud architects)? And more broadly how to build in-house a new innovation capability?
- How can GSK (and other pharmacos) better identify and prioritize most impactful ML initiatives?
(795 words)
[1]Digital in R&D: the 100 billion opportunity, McKinsey & Company
[2]Tufts Center for the Study of Drug Development, “Briefing: Cost of Developing a New Drug,” November 2014
[3]GSK Annual Reports (2017)
[4]Pharma companies using machine learning in drug discovery, blog Benchsci
[5]6 steps to AI leadership – Interview with John Baldoni from GSK, Forbes
[6]Exscientia website
[7]Insilico Medicine
[8]Ideo: Human-Centered Service Design (case 9-615-022), Harvard Business School Publishing (2016)
[9]Indigo Agriculture (case 9-617-020), Harvard Business School Publishing (2017)
Really interesting questions for big pharma. On the discovery side, computer aided drug design (CADD) has only played a small and supporting role in small molecule selection for preclinical development, but in the future hopefully AI will allow CADD to be more impactful. Main issues include lack of computing power to simulate the billions of molecular interactions between drug and target, and then we often don’t know the structure of the target, which is required for these simulations. Protein structure information has to be obtained using expensive and time-consuming techniques. In some cases it takes years to determine the structure of a protein, and even in those cases they are not always biologically relevant structures. In the future, researchers hope to be able to determine protein structure with the push of a mouse, but we are still years away.
One of the main issues with CADD is that once you have a target in mind, and can demonstrate that your molecule binds to that target, it is currently impossible to determine in silico whether that molecule will cause toxicity if it binds to additional “off-targets”. These off-targets effects can stop heart beats, or even inhibit essential liver enzymes that lead to systemic tox. Without protein structures for all of these vital proteins, numbering in the thousands, in silico CADD can only point us in the right direction for small molecule design. In the near term (10 years) there will definitely be a need to test these molecules in cells, animals, and eventually people to determine safety and efficacy.
The sheer scale of pharma- and therefore of of the opportunities like ML in this space- are astounding. I actually just read Patrick’s post about open innovation in drug discovery, and I’m curious whether (and if so, how) both of these transformative approaches could work together within a single company.
I’m glad that you call out data consolidation and structuring as a key pillar. It is such a simple but critically important point. Any company, whether they are currently leveraging AI or not, would be well advised to capture and store data in a way that will save them the painful retrofit down the road!