EasyJet: Reducing Delays with Machine Learning
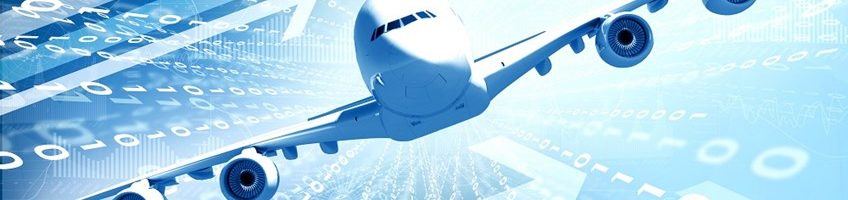
EasyJet is struggling to keep up its operational performance, and customer satisfaction is decreasing significantly. How can machine learning help?
EasyJet, the second largest low-cost carrier in Europe by number of passengers [1], is having difficulties to deliver good operational performance. On-time performance has been worsening every year during the last 5 years, and in its fiscal year 2017 only 76% of its flights arrived within 15 minutes of scheduled time, down from 87% in 2013 [2]. Increased disruption has had a very negative impact on customer satisfaction, which was 71% in FY2017, down from 80% in FY2013. Improving operational performance has become a key strategic priority for EasyJet in order to survive in an increasingly competitive market.
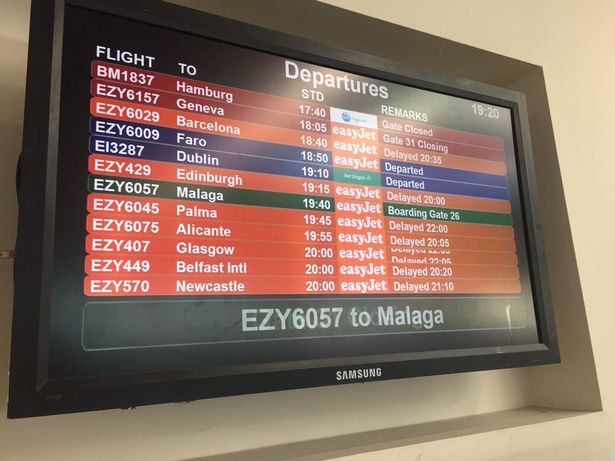
Why is machine learning important to improve delays?
Flight delays can be caused by factors that are not controllable by airlines, such as air traffic control, airport operations, or weather. However, the main cause of flight delays in Europe in 2017 was airline operations [4]. In turn, one of the main causes for airline operations disruption is aircraft maintenance problems. Therefore, improving the aircraft maintenance process is a key lever to improve punctuality.
Airlines typically follow reactive maintenance programs, meaning that they fix aircraft faults after they occur. By analyzing aircraft sensor data and using machine learning algorithms, airlines could potentially move to predictive maintenance [5], fixing problems before they are likely to happen. Machine learning can discover meaningful fault patterns in aircraft data that people would otherwise be unable to find [6]. Identifying potential faults before they become actual problems can improve aircraft reliability and limit delays.
How will EasyJet take advantage of machine learning to improve delays?
EasyJet has recently started to explore the use of predictive maintenance technology on its Airbus A320 aircraft family. EasyJet has launched strategic initiatives derived from its findings. For example, spare parts have been distributed around the network to support a faster response when machine learning algorithms predict that a fault could happen.
More broadly, EasyJet is also applying machine learning to improve other processes, such as optimizing in-flight food and drink choices [7].
With the ambition to become the most data driven airline in the world [8], EasyJet hired its first Chief Data Officer in August 2018. The new Chief Data Officer has broad experience in applied predictive technologies in other B2C organizations.
More importantly, EasyJet announced a five-year predictive maintenance partnership program with Airbus [9]. EasyJet will use Airbus’ Skywise aviation data platform, which aims to become the platform of reference used by major aviation players. Skywise is bringing together data from multiple airlines, and is enriching this data with Airbus’ aerospace expertise [10]. This platform is empowering airlines to develop machine learning algorithms to effectively implement predictive maintenance, with a data set that is much broader than the data set that airlines would own individually. Skywise is currently collecting data from more than 2,000 aircraft [11]. Having this broad and diverse amount of data is critical to properly assess the quality of the machine learning model through meaningful cross-validation [12]. This project has been launched by Airbus in collaboration with Palantir, a leading company in big data and machine learning. This tool will also help airlines to improve other processes, such as the decision-making process after unexpected events.
During EasyJet’s trial of the platform, Skywise successfully predicted 31 technical failures, which enabled EasyJet to remove components before a fault occurred. This meant that 31 flights would have been delayed, and this would have affected more than 4,400 passengers. EasyJet has now expanded the program to its entire A320 family fleet.
Moving forward, what else can EasyJet do?
First, EasyJet needs to develop personalized algorithms to adapt Skywise data to its own operations. Skywise incorporates data from dozens of airlines – and some of them have different operations than EasyJet (in terms of meteorology, length of routes, aircraft utilization, etc.). EasyJet needs to be able to adapt this data to its particular context. Therefore, EasyJet needs to continue building a strong data team and continue transitioning to become a data-driven organization.
On another note, EasyJet also needs to readapt its maintenance organization and processes. Way of working for predictive maintenance is very different than for reactive maintenance. EasyJet needs to adapt inventory management, culture, and procurement processes to benefit from Skywise data.
Lastly, there are some open questions that EasyJet’s leadership team need to address. One of them is to what extent should EasyJet share its data with Airbus. Being part of Skywise will allow EasyJet to access a vast pool of data that will enable enhanced predictive maintenance capabilities. However, this comes at a cost for EasyJet: it will need to share its valuable aircraft data with Airbus and with other competing airlines. Is participating in Skywise data benefiting more EasyJet or its competitors?
(780 words)
References
[1] CAPA – Centre for Aviation. “Europe’s Top 20 airline groups by passengers 2017”. January 20, 2018. https://centreforaviation.com/analysis/reports/europes-top-20-airline-groups-by-passengers-2017-lufthansa-wrests-top-spot-from-ryanair-394211, accessed November 13, 2018.
[2] EasyJet. 2017 Annual report. http://corporate.easyjet.com/~/media/Files/E/Easyjet/pdf/investors/results-centre/2017/2017-annualreport-and-accounts-v1.pdf, accessed November 13, 2018.
[3] Bristol Post. “Anger at Bristol Airport as EasyJet customers made to wait hours after multiple flights delayed and cancelled”. May 21, 2017. https://www.bristolpost.co.uk/whats-on/family-kids/anger-bristol-airport-easyjet-customers-69412, accessed November 13, 2018.
[4] Eurocontrol. “Coda Digest 2017”. May 31, 2018. https://www.eurocontrol.int/sites/default/files/publication/files/coda-digest-annual-2017.pdf, accessed November 13, 2018.
[5] Aircraft Maintenance Technology. “Connecting the data”. October 2018. ABI/INFORM via ProQuest, accessed November 13, 2018.
[6] J. Wilson, S. Sachdev, and A. Alter. “How companies are using machine learning to get faster and more efficient”. Harvard Business Review Digital Articles. May 3, 2016.
[7] Financial Times. “EasyJet deploys big data to tackle delays and cut costs”. November 17, 2015. ABI/INFORM via ProQuest, accessed November 13, 2018.
[8] EasyJet. “Executive management team”. EasyJet website, http://corporate.easyjet.com/about/management/airline-management-board, accessed November 13, 2018.
[9] Contify Aviation News. “EasyJet to use big data to reduce delays”. March 27, 2018. ABI/INFORM via ProQuest, accessed November 13, 2018.
[10] Airbus. “Airbus launches Skywise – aviation’s open data platform”. Press release, June 20, 2017. Airbus webpage, https://www.airbus.com/newsroom/press-releases/en/2017/06/airbus-launches-new-open-aviation-data-platform–skywise–to-sup.html, accessed November 13, 2018.
[11] Asia News Monitor. “Airbus Skywise – a revolution in the clouds”. July 20, 2018. ABI/INFORM via ProQuest, accessed November 13, 2018.
[12] Yeomans. “What every manager should know about machine learning”. Harvard Business Review Digital Articles. July 7, 2015.
I think this is a great application of machine learning but it is definitely important to get to build a database that is large enough to be reliable. Perhaps the way of solving the concern about sharing data is by avoiding disclosure of the name and other specific details that could potentially give an indication to competitors of the specific name of the airline. The one thing that in my opinion is relevant to consider is the fact that because safety is a very sensitive topic in the aviation industry, as the use of Skywise gets more popular and while the database becomes large enough, airlines need to find the right mix of resource allocation for predictive maintenance guided by data and traditional maintenance. It is key that airlines make sure that the traditional maintenance made by experts is still taking place. Since an error in maintenance can have fatal results, it is important that airlines do not over rely on data and neglect traditional maintenance.
Great topic! I think the tenuous nature of EasyJet’s partnership with Airbus is really interesting – on the one hand, as they ramp up their machine learning capabilities, this training data from a wide range of airlines will be critical in building out their predictive maintenance capabilities. On the other hand, relinquishing their own data to Airbus over the long term might hurt the company’s competitive edge. Furthermore, since aircraft data can differ so significantly based on airline, geography, weather patterns and other variables, I worry about how much “noise” is in this data that might lead the predictive maintenance algorithm astray. Beyond maintenance, I wonder how other airline-related ‘headaches’ could be solved by machine learning – the prospect of a better customer experience (through better food and beverage options or seat selections) is extremely appealing – could this lead to a higher end experience across the board, or will the benefits of these learnings just be reserved for business/first class travelers?
Really interesting essay that I enjoyed reading. Much like previous comments, I am curious to know more about the iterative nature of the systems. More importantly, the size of the dataset here seems critical in creating really valuable information across all airlines. However, it seems as if airlines are only developing these systems with their own proprietary data. Is this the case? If so, what limitations and shortcomings are there? Is there anyway to encourage more sharing of flight data and incident reports across airlines and even across airplane manufacturers that may help us get a better understanding of delays.
This is such an interesting application of machine learning. I agree with the aforementioned comments that shared data could be anonymized to remove conflicts of interest; however, this may reduce the specificity and the quality of the dataset that would otherwise make it “filterable” and valuable. I don’t see the risks of sharing data as a competitive disadvantage, as it is a highly commoditized industry in which competitive edges are easy to recognize but hard to implement. It is truly how Easyjet decides to implement the findings of the data that will be interesting to observe.