Digitalization of maintenance systems in capital-intensive heavy industries
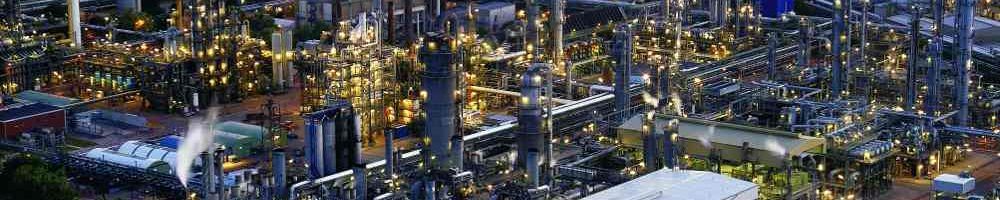
Based on a consulting project done for one of the largest global chemicals’ corporations – names and specifics sanitized to maintain client confidentiality.
Everyone who has ever worked at or with heavy industry facilities knows just how important the equipment maintenance is for plant economics. Efficient maintenance is one of the most significant drivers of both the top line (through increased capacity utilization) and bottom line (through cost savings on maintenance labor and spare parts/materials) of a manufacturing plant, especially in industries such as O&G, mining, chemicals, metals etc. that rely heavily on continuous processes often running 24/7 and involving highly destructive environments leading to rapid equipment degradation. Equipment breakdowns in these industries are not unexpected emergencies, but a daily reality they are dealing with. Yearly maintenance materials’ costs can reach 3-5% or more of the total asset base, and maintenance labor might contribute 30-50% of total labor costs on the plant. Efficient maintenance can also easily make a 5-10% difference in equipment utilization and the plant output. Coincidentally, many of these industries also produce commodity products which compete mainly on cost, and output volume is the only revenue driver the plant can control.
Digital revolution has enabled the Client to dramatically increase both maintenance effectiveness (as measured by equipment uptime) and efficiency (cost savings) through a number of initiatives, of which I will focus only on the most important two: the “reliability-centered maintenance strategy” (RCM) managed by an automated planning application, and the Industrial Internet-enabled predictive/condition-based maintenance.
Where old pre-digital maintenance function relied on either reactive maintenance (“fix it when it breaks down”, which leads to massive losses of productive time and possible spread of the damage to other valuable equipment) or ad hoc preventive maintenance scheduled by the supervisors based on their personal experience and/or observations of the production staff (“this sounds like it’s gonna blow!”), the automated RCM operates in a much more systematic way. The entirety of equipment in the facility was cataloged in the application (in this case, SAP-based) in a hierarchical manner, from the entire production shops down to individual pieces of equipment and further down to individual components such as bearings and mechanical seals. For each component, equipment piece and so on, a list of potential failure types was compiled, and each failure type was assigned a criticality level, ranging from unimportant failures that do not lead to lost productive time and/or additional costs, to those that can have catastrophic consequences for the facility and its workers and cannot ever be allowed to happen. Depending on the specifics, an equipment component can be assigned through a decision-tree algorithm to one of the maintenance types:
- “Reactive-by-design” maintenance, where preventive or predictive maintenance is not worth the effort (and labor)
- Regular preventive maintenance, where a component is simply replaced every X days depending on the historical statistical data on failure rates and a probabilistic cost-benefit analysis.
- Condition based-maintenance through equipment check-ups done by the previous worker shift or, importantly, data obtained from sensors installed on the equipment and digitally linked to the planning system. The sensor complexity can range from a basic thermometer to elaborate systems of vibration and pressure detectors, capable of identifying dangerous metal fatigue or vibration patterns associated with specific failures. Depending on the cost efficiency and criticality, sensor systems can be installed on the equipment itself and report monitoring data 24/7, or built into the workers’ handheld devices and used during equipment check-ups scheduled depending on the “P-F interval” (period of time between the first instance when future failure can be detected and the expected moment it occurs, which is also programmed into the planning system).
- Predictive maintenance capable of synthesizing data from the sensors mentioned above with the production statistics and other information, and identifying correlation between various system states and failures caused by them after a period of time.
The system then generates maintenance orders automatically at the beginning of every shift, compiles them into working plans for the shift and distributes them to individual maintenance teams. As a nice add-on, it allows monitoring the current production parameters, equipment downtime statistics and other important information which is helpful in managerial decision making with regards to those calls that cannot (yet) be made by a machine.
(683 words)
I wrote about Siemens, and your post just confirms how the new proposition of companies like Siemens will add substantial values for their clients. Siemens has recently launched Sinalytics, a platform that aggregates data from Siemens systems installed across the globe to help clients optimize the performance of such systems. One critical feature of Sinalytics is exactly its ability to optimally forecast preventive maintenance needs and minimize equipment downtime. The algorithms and heuristics behind Sinalytics implement cutting-edge concepts to maximize equipment availability, while minimizing maintenance and power costs.
One of my main concerns with this type of solution comes from looking at the client side: to which extent does the client loses bargaining power – and even the ability to switch to a different provider – once Siemens starts to monitor its operations in such a high level of details? In your specific case, did your client (potentially, also a Siemens’ client) design the algorithms and standards to optimally forecast preventive maintenance? If so, do you think that partnering with the supplier (e.g., Siemens, SAP, GE) could help improve even more the outcomes?
Indeed digitisation helps preventive maintenance in industries which are capital intensive. A new step going forward is linkage of SAP based plant maintenance schedule with 5D model. A 5D model captures minutest details of an equipment , including wearing patterns, replacement frequency. It talks with SAP PM and generates maintenance schedules for various equipment parts .
Thanks for the post, DS. The advent of condition-based maintenance also has a number of interesting downstream effects. First, by pulling real-time data off of sensors embedded in their equipment, OEMs are actually able to optimize the design of future generations of the product. Said differently, OEMs are able to strengthen the feedback loop on products in the field. Second, armed with the data, service companies are better equipped to diagnose underlying issues, maintain inventories of replacement parts and determine if they need to roll a truck (i.e., send a costly technician into the field). Finally, companies like PTC (link below) are leveraging the sensor data to deliver augmented reality applications/experiences that can effectively walk service technicians through repairs (think: digital training manual).
http://www.ptc.com/creopage
Interesting post DS – as someone who has spent some time in operating environments, the “sounds like it’s going to blow” comment makes me chuckle. I agree that a systematic maintenance program based on IOT and predictive analysis can be extremely powerful but it is important to note two very significant additional items when having this conversation:
1) The hardest part about adopting any new technology is adoption at the frontline. In my experience, folks are very skeptical of this technology and sometimes resist adoption. Any roll-out of this type of next-gen maintenance system must be accompanied with money and robust plans for training the workforce that will make it work.
2) These capitally intensive heavy industries that your post describes are typically critical infrastructure and may be managing potentially dangerous materials. If we tie all devices together and then connect them to a network, we have to be sure we have very secure cyber security in place to prevent malicious actors from getting into the system and causing havoc. This type of attack has already occurred at Saudi Aramco and will no doubt happen again.
http://www.nytimes.com/2012/10/24/business/global/cyberattack-on-saudi-oil-firm-disquiets-us.html
Thanks for the interesting post DS.
I am curious to learn a bit more about the economics of installing / operating a system like you describe, and what the front line change process looks like.
Having had some similar experience in a heavy manufacturing environment, I share Rocky’s number one concern above. I think that the front line (and consequently much of the management who came from the front line) frequently believe that “it sounds like its going to blow” is more reliable than the data they are getting out of this computer, and if they don’t inherently believe in the system, they are unlikely to make the tremendous capital and resource commitment required to get a program like this off the ground. I fundamentally believe that an IOT system is probably the right answer in applications where maintenance failures are very expensive, but do we have some good publicly available case studies from equipment manufacturers to help us sell that story to the front line?