Developing Machine Learning and Proprietary Data Sets at Goldman Sachs
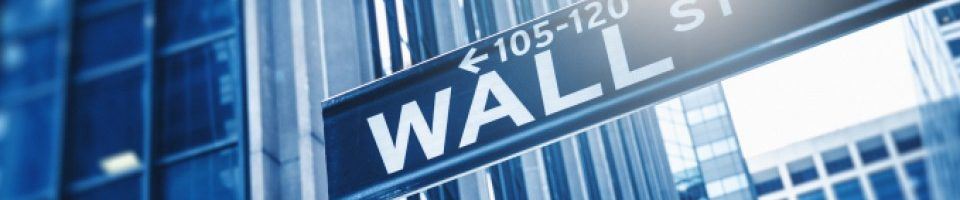
Goldman Sachs is a global investment bank, struggling to monetize customer data in a highly regulated environment. How are they getting around these restrictions?
Few industries are as ubiquitous and digitized as banking, which sits on massive amounts of clean, structured, financial data. Yet by and large, banks have maintained the same business model for centuries. The opportunity for disruption has enabled startups to flood the sector: Stripe, Apple and Square are changing how we pay for things, while digital currencies, peer-to-peer lending, ‘alternative-data’ providers and pay-as-you-go insurance platforms are threatening the universal bank model. In this essay, I investigate how an investment bank like Goldman Sachs can harness the power of its data through machine learning (ML). Due to its breadth of revenue streams, Goldman has the ability to address almost any area of ‘B2B’ finance, from investment banking, investment management, securities and lending. Nevertheless, monetizing B2B client data will be difficult given strong data protection and privacy agreements. As a result, I see Goldman Sachs making a strategic pivot towards consumer finance, where data constraints are less stringent.
In investment banking, strict NDA agreements limit the amount of proprietary data collected in any M&A transaction. As a result, the next frontier of process innovation will come from incorporating “alternative data” (e.g. credit card transactions, satellite images or weather forecasts) to develop proprietary insights on M&A opportunities. An investment bank armed with such insights would become a more strategic advisor to its clients and grow its market share as a result. A recent FinTech unicorn, Dataminr, is using big data from a variety of non-traditional sources to develop such insights, but it is struggling to incorporate these into the habits of professional advisors (i.e. 59% of Dataminr’s customers claim that ‘workflow integration’ is their biggest challenge). Given Goldman’s relationships with professional investors, where it is strongly embedded in their investment workflow, it has all the structural advantages to monetize this market.
When it comes to customer service, self-conversing bots are a major opportunity for ML to increase convenience and decrease SG&A cost. In private wealth management, Goldman’s clients are some of the wealthiest individuals in the world, but that is not a reason to overlook automation and bots. Using customer service bots would allow private bankers to spend more time with customers by automating basic support, data collection and automatic portfolio rebalancing based on customer requirement. Finally in lending, Goldman has proprietary data on millions of clients and their lending results. The potential of ML is to automate the underwriting process through stronger prediction algorithms that reduce bad debt expense, extend credit to higher-risk clients, and decrease administration costs.
In the short and medium term, Goldman will continue to expand its internal center of excellence of ML, set up in early 2018. So far, it hired the head of ML from Amazon, acquired dozens of FinTech companies with consumer data, and replaced its infamous equity trading team with computer engineers (i.e. The 600 equity traders from the early 2000s are all gone). In trading, ML algorithms predict which clients might be interested in specific investments and send quotes in real time. To succeed in the long term however, Goldman has recognized that it needs to create more proprietary data. Indeed, most machine learning algorithms are open source today, and anyone can use them free of charge against public data. Consequently, Goldman is incubating several initiatives aimed at collecting data from consumers, who are often less reticent than institutions to share data. It acquired three consumer startups in 2018 and developed an open trading platform called Marquee, which will be released to retail investors in Q4 2018. In addition, the firm recently released a new consumer lending platform, Marcus, to help consumers consolidate their credit card balances under one contract. Both platforms are run entirely by software with no human intervention.
For sustainable success in the next century, Goldman must not only develop proprietary insights from its proprietary data sets, but also incorporate these insights into the workflow of decision makers. Whereas machines are better at predicting very short term opportunities in reaction to tweets, earnings statements, or news, humans will maintain an edge in making long term predictions. As a result, humans will not be totally replaced and will need the ability to ‘overrule’ whatever the computer is saying.
To conclude, I would like to share some open-ended questions for the industry. We have seen how banks like Goldman are developing new consumer services in view of getting usage data, but how can they similarly leverage their B2B data? In M&A advisory for instance, Goldman mapped 146 distinct steps taken in any IPO, “some of which are begging to be automated”.
Bibliography:
- How to tell if machine learning can solve your business problem (Links to an external site.)Links to an external site. Harvard Business Review Digital Articles (November 15, 2016).
- Wilson, S. Sachdev, and A. Alter. How companies are using machine learning to get faster and more efficient(Links to an external site.)Links to an external site. Harvard Business Review Digital Articles (May 3, 2016).
- Daniel Faggella. Machine Learning in Finance: Current and Future Applications. com (November 5, 2018).
- Ed Oliver. The New Data Paradigm for Traders: Alternative Data in the Mainsteam. Dataminr (Q3 2018)
- Ayn de Jesus. Robo Advisors and Artificial Intelligence. com (July 6, 2018)
- Paul Clarke. Goldman Sachs Hunts AI Experts for Quant Team. Financial News London (January 30, 2018).
- Rob Daly. Goldman Preps Marquee Upgrade. com (October 16 2018)
- Liz Hoffman and Telis Demos. Wall Street Erases the Line Between its Jocks and Nerds. The Wall Street Journal (August 18, 2018)
- Nanette Byrnes. As Goldman Embraces Automation, Even the Masters of the Universe Are Threatened. MIT Technology Review (February 7, 2017)
- Charles Elkan. The Present and Future of AI for Financial Services Firm. Goldman Sachs website (September 7, 2018)
I think your point on Goldman’s need to integrate proprietary insights into its decision-making process is spot on. Having worked in Goldman’s corporate lending, I think part of the reasons why it is so difficult to gather data is because there are still so many groups of people passing data on manually at each decision-making step. The investment team also worked separately from those who ultimately booked the lending onto the firm’s balance sheet. Goldman indeed recognize the need to improve its information flow and have been recently implementing centralized data-collecting systems. I am optimistic about the potential for machine learning to work alongside humans to improve the quality of the firm’s lending practices.
While I would agree that there are a lot of routine, repeatable steps in investment banking, I would want to know more about ML’s applications in an M&A setting. In contrast to equity trading and other capital markets transactions, I would argue that M&A is too bespoke to effectively teach a machine. Every M&A situation is very unique to the company, and I’m not sure that there are a definable mix of variables that one can insert to get an answer as to how one should act in a deal situation. There are so many stakeholders involved with a transaction that it may be impossible to have a tool that optimizes outcomes for all involved. And per your earlier point, I don’t believe companies will be willing to open up that information to being shareable with respect to any ML tool so that it can ‘learn’. In summary, by my read ML will be most useful in investment banking contexts when it is deployed to make a routine workstream more efficient, but its ability to be a strategic advisor in sophisticated corporate transactions remains up for debate.
I would have to say that M&A and IPOs are very different. The capital markets part of investment banking – where IPOs will fall under – have routine steps that bankers take in order to execute the deal. I definitely agree any process that has measurable, predictable steps can be automated and even better so with ML. On the other hand from my experience in investment banking, M&A tends to have some steps that are the same regardless of the situation, but most are not. I would be curious to learn more as to how the author thought about the potential of ML in M&A situations.
On the consumer banking part of the article, I didn’t think about the data play with this strategy. I just thought that GS wanted to get more deposits in the door through Marcus so it can grow its balance sheet and lend against it, but the data angle makes complete sense. I’m going to check out Marquee when it comes out and see what type of data GS may be collecting from its retail investors.