Data-Driven Dress Dealing
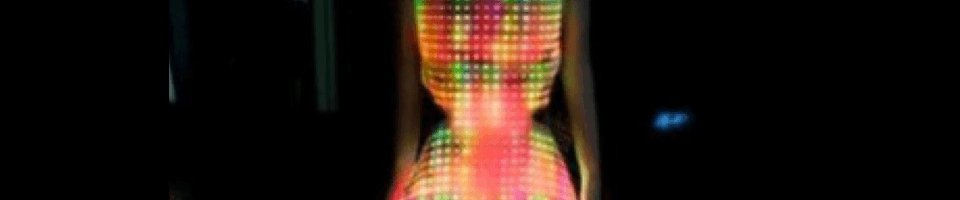
Retailers have historically made decisions based on intuition, but what happens when companies like EDITED begin leveraging Big Data to track runway trends, analyze product movement, and recommend pricing and promotions?
Merchant Monarchs
Since its early inception, the world of retailing has been dominated by a single role: the merchant. This person is not only responsible for selecting which products to manufacture and sell, but s/he is also usually in charge of determining pricing and promotion cycles. Having an eye toward potential trends and being able to anticipate what the market will want (in addition to how much and when) are crucial skills that most merchants believe are innately imbued: one may able to hone them over time, but taste cannot be taught. In this world, the merchant reigns supreme—so much so, in fact, that the CEO of J. Crew, Mickey Drexler, has long been known as the “Merchant Prince” for his ability to see the future of fashion and sell it to the American people [1].
Digital Disruption
Retailers have always captured some data on product performance, tracking sales by department over time and discounting merchandise based on history and customer psychology [2]. But with the advent of digital information storage and ecommerce, the availability and accuracy of this data has radically shifted. Everything from purchase patterns to product specifications is now trackable and analyzable, allowing companies like EDITED to capitalize on this information. This web-based platform creates tools and dashboards from information that retailers and merchants have long undervalued, utilizing a proprietary machine learning and natural language processing system [3]. By tracking over 330 million SKUs at 90,000+ online retailers and brands with a sophisticated network of web crawlers (akin to Google’s Googlebot system), EDITED gathers the raw data that is used as inputs for its IBM-Watson-like system [3, 4]. This platform then produces dashboards that can be used by retailers to enable decision-making in three main areas: product assortment, pricing/discounting, launch timeline, and trend analysis.
Screenshot from EDITED’s Dashboard Platform
Predicting Products
Through assessment of competitors’ ecommerce sites, merchants can obtain a clearer, real-time picture of what their market looks like—understanding who is carrying what products and when gives retailers ideas about potential under- or over-stocking issues [3]. EDITED’s tool allows for optimization of assortment to prevent either the cost of missed revenue from lack of supply or the cost of forced discounting to sell off excess volume.
Profitability Plays
By scanning websites of both brands and retailers, EDITED can generate a price map that indicates where a merchant’s price is relative to the market. This gives the merchant the power to raise or lower prices and maximize profits in the long run. Not only can it help with initial pricing, but the system can also determine when the best time is to discount, instead of relying on antiquated seasonality-driven methods that often do not consider real-world circumstances or current demand [2]. Given the proliferation (and potentially reputation-damaging nature) of markdowns in the market, ensuring that retailers are not leaving money on the table by discounting too early is a critical measure of success.
Trending Topics
As the world’s consumption of media in all its forms increases in both speed and volume, brands are now generating an overabundance of content to satiate their customers. EDITED’s platform ingests these images and text from sources like Instagram, Facebook, and style blogs that it then feeds into its system [3]. The output is trend tracking and analysis that, when combined with human intelligence, can provide retailers with a better representation of what cuts or colors are en vogue.
Optimization Opportunities
There is no doubt that data will continue to play an ever-increasing role in the job of merchants and retailers, supplanting much of the intuition and art-based thinking that has guided these professionals in the past. However, fashion companies today have neither the in-house capacity nor the resources to develop these tools themselves [4]. Analytics firms like EDITED can continue to sell their SaaS platforms to retailers for the time being, but for their model to succeed in the future, they should focus on two points of differentiation.
- Determine data ownership: As with other applications of AI, data ownership and privacy concerns are high when utilizing a shared database system. EDITED currently works with publicly accessible data from websites, but to strengthen their product offering, they may want to consider approaching retailers for proprietary access. At that point, integration of said data into the system may present a conflict of interest that EDITED should figure out now.
- Focus on the future: EDITED’s product is great for determining what to do in the present situation—e.g. what are today’s hot products and how should they be priced on the market today. However, a lot of the value of this data is in forward-looking analyses—how can a fashion company know what future trends will be a hit and which SKUs will sell. This would also allow EDITED to position itself better against its main competitor in the fashion analytics space, WGSN [5].
Word Count: 797
Sources
[1] N. Paumgarten, “The Merchant,” The New Yorker, September 20, 2010. http://www.newyorker.com/magazine/2010/09/20/the-merchant, accessed November 2016.
[2] V. A. Kansara, “The Long View | How Realtime Data is Reshaping the Fashion Business,” August 3, 2011. https://www.businessoffashion.com/articles/long-view/the-long-view-how-realtime-data-is-reshaping-the-fashion-business, accessed November 2016.
[3] https://edited.com, accessed November 2016.
[4] K. Abnett, “Is Fashion Ready for the AI Revolution?,” April 7, 2016. https://www.businessoffashion.com/articles/fashion-tech/is-fashion-ready-for-the-ai-revolution, accessed November 2016.
[5] K. Noyes, “What’s on trend this season for the fashion industry? Big Data,” Fortune, September 22, 2014. http://fortune.com/2014/09/22/fashion-industry-big-data-analytics, accessed November 2016.
Genius idea! really liked the leverage of public information to create business value. Agree with you 100% that in order to develop their business, EDITED will have to get retailers data and that might create a conflict of interest.
Another thing that crossed my mind is that this is a classic example of Machine Learning system that might make some marketing functions redundant in the near future. However, it seems the main consumer of this platform is Marketing and Sales functions, I wonder how they will resonate this matter?
Another developing area can be using real time pricing to update online ads. For example, connect the system with Facebook or Google advertising platforms and adjust the ads on real time in order to offer the most relevant price to the relevant consumer in any given moment.
Interesting read! I’m intrigued by the idea that this analysis is done solely on publicly available information and appears to be independent of any demographic data to formulate trends. Does EDITED work on a global scale, and does it geographically segment? It seems that EDITED works best on mass market retailers with similar types of products, but would you see an equivalent amount of value to ’boutique’ fashion houses that look to be unique?
In looking at a fashion-prediction model, I would be curious to know how EDITED integrates with fashion magazines (who claim to know everything that’s hot) on predicting next season’s looks. I also think there’s a huge application here in the consumer market for those who want to be ahead of the curve in the fashion world once the prediction model is created.
Great post!
I agree this is a natural next step for fast fashion retailers such as Zara and H&M and potentially other fashion retailers to incorporate in their operating model and compete more effectively going forward. Adoption of this technology will impact the operating model of fashion brands and retilers in several ways:
♣ Less labor investment in designers and ideally less variability in the hit/miss of styles selected to go to production, given the machine-learning algorithm will analyse trends and what items people are searching for currently
♣ Faster idea generation time and overall shorter throughput time from idea generation to sale of product to end consumer through higher levels of automation in the operational design, testing and sale process
♣ Minimize inventory markdowns and waste from unpopular designs
________
Nancy Laws, ‘Can this new industry disrupt fast fashion?’, Huffington Post, March 2, 2016, http://www.huffingtonpost.com/nancy-laws/can-this-new-industry-dis_b_9362344.html, accessed on November 20, 2016.
Seems like a ton of upside for the retailer by leveraging this data! Makes complete sense, especially for the retailers who have already embraced more advanced data solutions in their supply chain management system (e.g., H&M).
One potential extension of this application could be to allow customers to add in their preferences to help them determine what type of clothes they may want to buy. Several start-ups such as trunk club aim to predict what a customer would want to buy from basic descriptions. Perhaps if a customer was willing to share their purchasing habits more openly, more retailers could reach them with targeted marketing. Who knows, maybe people would be more reluctant to delete “spam” emails if they were filled with products that they actually want!
After reading this, I cannot imagine how much work retailers put into tracking promotions, pricing, and competition before the advent of this technology. While this seems like a very efficient way for retailers to aggregate industry data and assess themselves relative to the market, I wonder whether EDITED will ever provide this information in consumer form. Specifically, the ability to filter similar products and compare sale prices and promotions across the industry is a feature I’d pay for. While some online clothing search platforms like Shopstyle and Polyvore help with aggregating similar products and comparing price, they often partner with certain retailers and only promote specific brands. However, I would pay for an app like this that were agnostic and allowed me to scan almost any retail product SKU before I made my final purchase.
EDITED is providing a truly unique service. As we discussed in class, the quality of the output here is truly dependent on the quality of the data that is provided. Interestingly, on EDITED’s website they highlighted while anyone can provide this service in theory, not everyone can provide the same level of quality data. What measures does EDITED have in place to ensure the quality of the data? Additionally, how helpful is this information when analyzing historical patterns given the time period of data that EDITED has? Finally, retailers are increasingly working to utilize omnichannel and provide a more seamless integration between their online offerings and in-store offerings. But I wonder, if analyzing websites provides the most representative picture of a retailers offerings? Do all retailers show the full catalog of offerings on their websites and how much flexibility do individual stores have in determining prices / when to promote? If there is a meaningful difference between in-store offerings and online offerings, is the data that EDITED uses for its analysis truly compelling?
Additionally, are customers able to customize the data that they view by retailer? In other words, can customers request data from a curated list of competitors. And as you noted, what problems may this present if EDITED moves towards requesting proprietary data from retailers in the future? How will EDITED resolve the potential conflicts of interest that may result from having access to data from competing retailers.