Cyft: The Promise of Machine Learning to Deliver Health Care Over Sick Care
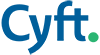
Cyft, a cloud-based predictive analytics platform, is making big bets that it will be able to predict your health in the future, and ultimately improve health outcomes and bring down costs.
The Evolving State of the US Healthcare System
The US healthcare system is slowly shifting the way it delivers care from reactive to proactive, placing greater emphasis on primary care, chronic disease prevention, and averting hospital readmissions. This represents a significant paradigm shift. Under traditional fee-for-service payment schemes, hospitals were reimbursed for each service provided, with higher rates attributed to acute hospitalizations and procedures, without regard for patient outcomes. Now, under value-based healthcare arrangements, health systems work with fixed budgets and are incentivized to keep patients healthy and out of the hospital. This means that for the first time, healthcare organizations are finally held financially accountable for the quality of care they deliver.
In order for this new arrangement to be successful though, healthcare systems need new tools to identify patients at high risk for developing disease and other adverse events—only then can they deploy the right resources for the right patients at the right time. This information gap has spurred intense competition between data analytics companies, seeking to answer questions such as:
- Which patients are most likely to return to the hospital after discharge?
- Which patients are most likely to come into the emergency department for low blood sugar or worsening heart failure?
- Which patients are at high risk for falling in home and fracturing their hip?
Of these, Cyft stands out for its ability to synthesize several unique data sources in order to improve patient care.
Cyft: Where Machine Learning Meets Patient Care
Cyft is a cloud-based predictive analytics platform that aggregates and analyzes multiple streams of historical data in order to predict the likelihood of future adverse events. Powered by machine learning algorithms and natural language processing, Cyft considers over 30 different data sources and both structured and unstructured data elements—such as claims data, free text from electronic medical records, call center transcripts, and patient-generated data (from continuous glucose monitors, for example)—to improve care management practices. Its goal is to provide healthcare organizations with real time information to anticipate and prevent adverse events.
In the short term, Cyft has focused on partnerships with health plans, hospitals, and pharmaceutical companies to drive value and improve outcomes. Last year, Cyft announced a partnership with Joslin Diabetes Center to improve the care of children diagnosed with type I diabetes, the second most common childhood chronic disease after asthma [1]. Cyft has also bolstered its predictive analytics capability by partnering with MedMinder—a company that helps patients manage their complex drug regimens via an electronic pill dispenser—to integrate in-home medication adherence data into its algorithm. The hope is that this will allow for organizations to better prioritize which patients to target with care management resources [2].
In the long term, Cyft is focusing on building credibility among healthcare providers by testing several use cases and publishing its research in high impact journals. Its efforts thus far have been successful: in 2017, Cyft demonstrated value in detecting variation in surgical treatment of young women with breast cancer [3], classifying patient acuity in an inpatient setting [4], and anticipating falls during hospital admissions [5].
Looking Towards the Future
As Cyft evaluates additional growth opportunities, it should consider partnering with state and federal authorities to help Medicare and Medicaid effectively target interventions, anticipate epidemics, and prevent adverse events. As the largest payers for medical care in the country, collaboration with government agencies could add tremendous value to the company and patients alike.
Cyft should also consider adding additional data into its analytics model, particularly social factors that drive health outcomes such as housing and food instability, access to transportation, and social stressors. Given that social determinants drive a large part of an individual’s health outcomes (40% of health outcomes, whereas medical care only accounts for 10-20%), adding these elements will strengthen their predictive capabilities [6].
Finally, as Cyft grows, it should continue to prioritize data privacy and security. Ultimately, the company must decide the degree to which it will allow patients themselves to control access to, and distribution of, their own data. Patients will also need to give input into how much they want to know about their future outcomes. Without appropriate safeguards, there is highrisk for data misuse and patient harm.
Looking toward the future, there are a few critical considerations for the company:
- The more streams of data available, the more powerful Cyft’s predictive analytics will be. Are there any data streams that are off-limits to predict future health (e.g. credit purchase history, genomic sequencing data)? As long as an individual gives permission, does it matter?
- Patients that are disengaged with the healthcare system are less likely to appear in hospital training data sets. What are the risks associated with using training data that may exclude the most marginalized populations and how can Cyft mitigate them?
Word Count: 800
References
[1] Trust, C. (2018). Leading Institutions to Focus on Improving Type 1 Diabetes Care with Machine Learning. [online] Prnewswire.com. Available at: https://www.prnewswire.com/news-releases/leading-institutions-to-focus-on-improving-type-1-diabetes-care-with-machine-learning-300472183.html [Accessed 12 Nov. 2018].
[2] Prnewswire.com. (2018). Cyft and MedMinder Partner to Improve Care Management. [online] Available at: https://www.prnewswire.com/news-releases/cyft-and-medminder-partner-to-improve-care-management-300512297.html [Accessed 12 Nov. 2018].
[3] Dodgion, C., Lipsitz, S., Decker, M., Hu, Y., Quamme, S., Karcz, A., D’Avolio, L. and Greenberg, C. (2017). Institutional variation in surgical care for early-stage breast cancer at community hospitals. Journal of Surgical Research, 211, pp.196-205.
[4] Brennan, C., Meng, F., Meterko, M. and D’Avolio, L. (2016). Feasibility of Automating Patient Acuity Measurement Using a Machine Learning Algorithm. Journal of Nursing Measurement, 24(3), pp.419-427.
[5] Shiner, B., Neily, J., Mills, P. and Watts, B. (2016). Identification of Inpatient Falls Using Automated Review of Text-Based Medical Records. Journal of Patient Safety, p.1.
[6] Hood, C., Gennuso, K., Swain, G. and Catlin, B. (2016). County Health Rankings. American Journal of Preventive Medicine, 50(2), pp.129-135.
I agree that there is great potential in using data to keep people healthy, thereby minimizing medical interventions. The question then becomes, as you point out, how do you protect people’s right to privacy and ensure their data is safe, especially since, in order to generate individual forecasts, the data cannot be truly anonymized (i.e. there will always be a key to link it back to the original subject)
Cyft will be able to shed light on correlations the medical community may not have thought of, but to do so will require plenty of “training data” where scientist and doctors will fine tune (or correct) the results. To this end, can companies like MedMinder provide their customers data freely? and even if they legally are able to, is it ethical to do so?
This was super interesting – thank you! I very much agree with your critical considerations, but on the privacy point I’m inclined to think that the success of direct-to-consumer health diagnostic tools, such as 23andme, suggests a willingness for patients to provide data in exchange for better / more preventative health outcomes. One concern I have is the extent to which medical providers will use this data to drive care decisions and resource allocation – as we’ve learned, machine learning can create blind spots based on its training data (which you allude to in your second consideration), so could you end up neglecting the anomalous, very dangerous / costly cases that go less noticed because resources are focused on other patients Cyft points to? Additionally, I wonder if Cyft could partner with more tangible medical tracking devices (even something like FitBit / Apple Watch) to gain data and become more visible to the consumer, so consumers could also actively learn to care more about tracking and understanding their health.
Interesting, thought-provoking article! As for the questions you pose, I am actually wary of some of the data sources that they already use such as claims data, call-center transcripts as well as credit purchase history and genomic sequencing data. While I can see the value of the predictive analytics in promoting preventative care, these data sources can be very easily exploited were they to reach the wrong hands. I believe medical records from healthcare institutions can be shared with the consent of the patient to share information on past health conditions, current state, and potential future risks, but going beyond that is too risky. Perhaps an alternative way to use predictive analytics for preventative care is to provide users with a Fitbit, or another health-tracking wearable, which can track basic health such as heart rate and monitors exercise levels, and transfers the data to medical institutions when abnormalities are observed.
I am curious to see if Cyft can take off in other countries, as I can imagine they will meet significant regulatory constraints related to data protection.