Can Machine Learning Save Innovation in Pharmaceuticals?
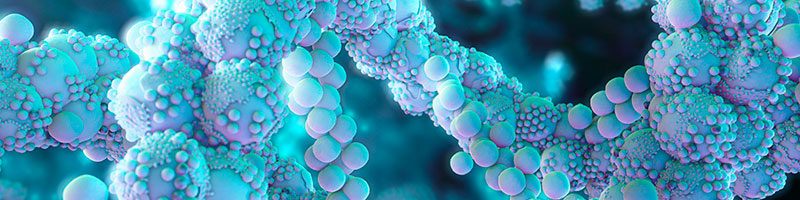
Have the economics of investing in new drug discovery and development become so unattractive that investment in new drugs will come to a halt? GlaxoSmithKline and other bio-pharmaceutical companies are turning to machine learning as a potential solution to make the drug discovery process more efficient, with the hopes of greatly reducing both the time and cost associated with R&D.
In 2015, Earnst Berndt of MIT published a paper exposing that the financial returns associated with new prescription drugs had been diminishing overtime, and came to an alarming conclusion: “if this level of diminishing returns persists… rewards for innovation will not be sufficient for pharmaceutical manufacturers to maintain the historical rates of investments needed to sustain biomedical innovation.”[1] Diminishing returns to the pharma industry are a result of the growing regulatory and pricing pressures, increased generic drug usage, and most importantly, climbing R&D costs.[2] Recent estimates show the cost of developing a new drug (new molecular entity) is approximately $1.8 billion.[2] These ongoing headwinds beg the question: have the economics of investing in new drug discovery and development become so unattractive that investment in new drugs will come to a halt?
GlaxoSmithKline (GSK) and other bio-pharmaceutical companies are turning to machine learning as a potential solution to make the drug discovery process more efficient, with the hopes of greatly reducing both the time and cost associated with R&D. The drug discovery process costs ~45% of the entire R&D budget and includes four major stages: target-to-hit, hit-to-lead, lead optimization, and preclinical (see Exhibit A Drug Discovery and Development).[2] GSK’s head of platform technology and science John Baldoni has said the company’s goal is to decrease the drug discovery process from an average of 5.5 years to one year.[3] A change of this magnitude would not only greatly reduce GSK’s cost of drug discovery, but also give consumers access to revolutionary drugs that could improve their lives at a much faster rate.
In the short-term, GSK’s primary strategy for unlocking the potential of machine learning in drug discovery is centered around partnerships with startups such as ExScientia and Cloud Pharmaceuticals that are attempting to use artificial intelligence to automate the drug design process, or in other words, bring the process “in-silico.”[4] Specifically, ExScientia is using experimental data on well-researched molecules to predict properties of new molecules using machine learning.[5] This helps them to identify hits and leads faster in the target-to-hit and hit-to-lead stages of drug discovery. Cloud Pharmaceuticals’ machine learning operation is also focused on improving the target-to-hit and hit-to lead processes, instead leveraging molecular modeling to find the best hits and leads.[6]
Because the technology of each of these startups is still unproven and in a nascent stage, GSK should continue to build partnerships with more of these companies focused on improving the target-to-hit and hit-to-lead process so that it can test the effectiveness of different algorithms and data sets. As these partnerships progress, GSK must decide if they want to bring this technology in-house through building internal capabilities, acquisition, or licensing the technology, or alternatively if they want to permanently outsource portions of their drug discovery process. This decision should hinge on whether they believe their partner is providing a differentiated service that is hard to replicate. If this is the case, GSK should try to bring the process in-house so that competitors cannot access it. Otherwise, a licensing deal may be more beneficial.
Longer-term, GSK should begin to explore the application of machine learning to later stages in the drug discovery process, first lead optimization and ultimately pre-clinical. The lead optimization and pre-clinical stages, however, traditionally involve testing drugs on animals to prove efficacy and safety. The data generated in pre-clinical trials is used in the application to the FDA to allow for clinical trials on humans to begin. For this reason, changing the later stage drug discovery processes to an in-silico method will require a much higher degree of trust in the machine learning methods as well as regulatory approval (e.g., FDA). As GSK starts to incorporate machine learning into more of its processes, the company should work with the FDA to ensure that it will be able to receive approval when it is needed in the future.
Although machine learning in drug discovery shows promise, it is too soon to tell if it is will solve the pharmaceutical industry’s core problem of growing R&D costs. If machine learning advancements do succeed in revolutionizing drug discovery, there are many interesting questions that arise surrounding the trust and benefactors of the technology. For example, how well do you think in-silico studies should have to perform in comparison to animal trials before companies should start skipping animal trials and relying solely on computer generated data before testing in humans? Additionally, do you think that pharmaceutical companies should be obligated to pass on the savings from automating R&D to the end consumer?
[751 words]
[1] Berndt, Ernst R., Deanna Nass, Michael Kleinrock, and Murray Aitken. “Decline In Economic Returns From New Drugs Raises Questions About Sustaining Innovations.” Health Affairs 34, no. 2 (2015): 245-52. doi:10.1377/hlthaff.2014.1029.
[2]Paul, Steven M., Daniel S. Mytelka, Christopher T. Dunwiddie, Charles C. Persinger, Bernard H. Munos, Stacy R. Lindborg, and Aaron L. Schacht. “How to Improve R&D Productivity: The Pharmaceutical Industrys Grand Challenge.” Nature Reviews Drug Discovery 9, no. 3 (2010): 203-14. doi:10.1038/nrd3078.
[3] Hirschler, Ben. “Big Pharma Turns to AI to Speed Drug Discovery, GSK Signs Deal.” Online article. July 01, 2017. Thomson Reuters. Accessed November 10, 2018. https://www.reuters.com/article/us-pharmaceuticals-ai-gsk/big-pharma-turns-to-ai-to-speed-drug-discovery-gsk-signs-deal-idUSKBN19N003.
[4] Tyer, Dominic. “GSK Collaborates on AI-driven Drug Design with Cloud Pharmaceuticals.” Pharmaphorum. October 03, 2018. Accessed November 13, 2018. https://pharmaphorum.com/news/gsk-ai-drug-design-cloud-pharmaceuticals/.
[5] McConaghie, Andrew. “GSK to Accelerate Drug Discovery with Artificial Intelligence Company.” Pharmaphorum. July 03, 2017. Accessed November 13, 2018. https://pharmaphorum.com/news/gsk-accelerate-drug-discovery-artificial-intelligence-company/.
[6]Keinan, Shahar, Elizabeth Hatcher Frush, and William J. Shipman. “Leveraging Cloud Computing for In-Silico Drug Design Using the Quantum Molecular Design (QMD) Framework.” Computing in Science & Engineering 20, no. 4 (2018): 66-73. doi:10.1109/mcse.2018.042781327.
I would hope there’s an economic incentive to pass on R&D savings to the consumer. Though the problem is systemic, I also wish the barriers to new drugs were lower, as I think the harm they prevent through thorough testing is outweighed by the harm they FAIL to prevent by making new drugs so hard to get to market.