Can Machine Learning Deliver Airbnb’s Next Leg of Growth?
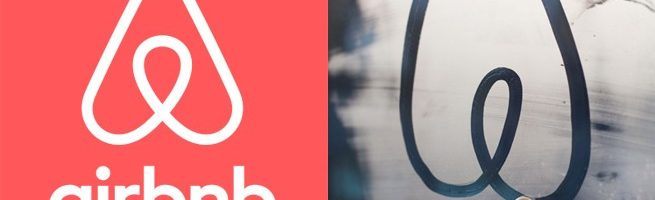
Machine learning has revolutionized the way Airbnb matches guests with hosts. Now, can the company use this technology to maintain its growth and address its growing pains?
Launched in 2008, Airbnb has become a $31 billion behemoth in the hospitality industry. [1] A large part of that success is driven by a 2014 change: the introduction of its machine learning search ranking model. [2] Airbnb operates in a two-sided market, where it must match guests (people looking for accommodations) with hosts (people renting out spaces). Machine learning has been able to improve Airbnb’s match rate and deliver significant growth. But, can Airbnb continue growing at the pace it does today?
How Airbnb Uses Machine Learning
With 5+ million unique places to stay and 15,000+ activities, Airbnb uses machine learning to generate personalized rank search results. [3] When trying to find the best listings for each guest, Airbnb looks at over 100 factors, taking into account not only explicit requests, like length of stay and number of rooms, but also implicit signals like décor, setting, and preferences of similar guests. [2]
But, Airbnb’s machine learning capabilities are not just used to service guests. The company also offers a number of host services. One tool is its pricing algorithm, based on an open-source machine learning tool called Aerosolve. Offered to hosts through pricing tips and Smart Pricing, Airbnb’s pricing algorithm constantly iterates based on key attributes (like size, location, and amenities), demand, and seasonality. [4] Airbnb also uses machine learning to understand host preferences, studying occupancy preferences (i.e. do hosts want gap days between requests?) and notice time preferences (i.e. how early do hosts prefer to receive requests?). [5]
By continuously improving its algorithms, Airbnb has rapidly grown its Homes segment. Going forward, the company should continue thinking of creative ways to leverage its data to offer new services to its hosts and guests.
The “End-to-End” Travel Company
Airbnb hopes to one day become an “end-to-end” travel company. CEO and co-founder Brian Chesky has stated that by 2021, the majority of Airbnb’s offerings “will be the new things that we are doing as of 2017 on.” [6] A recent report from Morningstar states that Airbnb’s future growth will likely come from global expansion, new product categories (i.e. Airbnb Experiences, transportation, and restaurant bookings), new accommodation offerings (i.e. luxury homes and boutique hotels), corporate travel business, and an Airbnb Superguest loyalty program. [7]
As demonstrated by Airbnb Experiences, machine learning will continue to play a key role. Launched in November 2016, Experiences uses machine learning to generate personalized results for each guest through tracking experiences that guests have shown interest in, location, dates, and group size. The new product line has seen 2500% growth during 2017. [8]
With the launch of “Airbnb Corporate & Business Travel” and “Airbnb Experiences” and the acquisition of Luxury Retreats and Resy, the company has more data on its guests than ever before. Airbnb should use that data to develop new offerings. For example, as Airbnb continues to formulate its loyalty program, the company could consider creating a loyalty program that offers personalized benefits. Using the data gathered on each user, the company could offer access to unique rentals or difficult restaurant reservations, concierge services, or even gym benefits.
Growing Pains
While it’s data-driven approach has enabled Airbnb’s growth, the company is also facing growing pains. A few to be considered:
- Competition from OTAs – Online Travel Agencies (OTAs) like the Booking Holdings and Expedia have also expanded into the home sharing business. Given the OTAs’ wide selection of hotels in addition to their home share offerings, these businesses may become a threat. Airbnb should focus on rolling out its loyalty program and build out a comprehensive offering to become a one-stop shop, using machine learning to discover why guests may book elsewhere. However, will that be enough?
- Regulatory challenges – In 2016, the American Hotel and Lodging Association backed FTC efforts to investigate Airbnb’s impact on local housing prices. The company continues to face ongoing local and state regulatory challenges. [9] Airbnb’s expansion into new areas (i.e. hotels and Experiences) could help alleviate some of its regulatory pressures. But, what other steps should they be taking?
- Racial and discrimination claims – In 2014, HBS professor Ben Edelman released a paper stating that black users were 16% less likely to be accepted than identical guests with white names. Airbnb has since seen a number of discrimination complaints and lawsuits. [10] Although Airbnb has now launched Instant Book, which allows guests to book identity-free, discrimination remains an issue. Airbnb could use its data to identify other communities with low acceptance rates and reach out to them. Similar to its NAACP partnership, Airbnb can work with other community groups to increase the number of hosts servicing that community. [11] Are there other steps Airbnb can take to prevent discriminatory actions?
Ultimately, can Airbnb’s data-driven approach help it maintain its growth trajectory? Or will Airbnb fail to overcome its growing pains?
(Word Count: 800)
[1] Greg Bensinger, “Airbnb Valued at $31 Billion After New Funding Round,” The Wall Street Journal, March 9, 2017, [https://www.wsj.com/articles/airbnb-valued-at-31-billion-after-new-funding-round-1489086240], accessed November 2018.
[2] VB Staff, “Airbnb VP talks about AI’s profound impact on results,” VentureBeat, June 14, 2017, [https://venturebeat.com/2017/06/14/airbnb-vp-talks-about-ais-profound-impact-on-profits/], accessed November 2018.
[3] Airbnb, “About Us,” [https://press.airbnb.com/about-us/], accessed November 2018
[4] Dan Hill, “The Secret of Airbnb’s Pricing Algorithm,” IEEE Spectrum, August 20, 2020, [https://spectrum.ieee.org/computing/software/the-secret-of-airbnbs-pricing-algorithm], accessed November 2018.
[5] Bar Ifrach, “How Airbnb uses Machine Learning to Detect Host Preferences,” Medium Corporation, April 13, 2015, [https://medium.com/airbnb-engineering/how-airbnb-uses-machine-learning-to-detect-host-preferences-18ce07150fa3], accessed November 2018
[6] Maggie Overfelt, “It’s been a trip to $31 billion. Now Airbnb wants to remake the entire travel industry,” CNBC, May 15, 2017, [https://www.cnbc.com/2017/05/15/its-been-a-fantastic-voyage-to-31-billion-what-airbnbs-next-big-trip-isnt-booked.html], accessed November 2018
[7] Dan Wasiolek and Robert Le, “Airbnb’s Network Effect Offers Investors a Unique Stay,” Morningstar Pitchbook, July 17, 2018, [https://files.pitchbook.com/website/files/pdf/3Q_2018_Morningstar-PitchBook_Airbnbs_Network_Effect_Offers_Investors_A_Unique_Stay.pdf?pdf=repromo], accessed November 2018
[8] “Airbnb Doubles Down on Experiences, Expanding to 1000 Destinations and Adding New Passion Categories in 2018,” Airbnb press release (San Francisco, CA, February 23, 2018)
[9] Katie Benner, “Inside the Hotel Industry’s Plan to Combat Airbnb,” New York Times, April 16, 2017, [https://www.nytimes.com/2017/04/16/technology/inside-the-hotel-industrys-plan-to-combat-airbnb.html], accessed November 2018
[10] Elaine Glusac, “As Airbnb Grows, So Do Claims of Discrimination,” New York Times, June 21, 2016, [https://www.nytimes.com/2016/06/26/travel/airbnb-discrimination-lawsuit.html], accessed November 2018
[11] Tracy Jan, “Faced with complaints of discrimination, Airbnb partners with NAACP to recruit black hosts,” Washington Post, July 26, 2017, [https://www.washingtonpost.com/news/wonk/wp/2017/07/26/faced-with-complaints-of-discrimination-airbnb-partners-with-naacp-to-recruit-black-hosts/?noredirect=on&utm_term=.d7a234f21737], accessed November 2018
Nicely written and really interesting article. I use airbnb a lot, but I did not know about their approach to machine learning. In terms of the risks that the company faces, I think they are in a good position to defend against competition. Their network effect and first-mover advantage make it hard for others to compete with them in the sharing economy. I think they might have larger problems as they try to bridge into the traditional hotel space though. In that context, I think any regulatory pressure that limits their home sharing is a major concern.
Looking at machine learning in particular, I can see how they might use tracking and language processing algorithms to refine their travel and experience products. I agree that that could be really productive for them. I think there is a geographic element to this that could pair really well with the company’s existing model. For example, focusing first on neighborhood services like gyms could be especially useful. Right now, there is no one who aggregates and offers that kind of access to travelers. It would be easy and complementary for airbnb to pair people with services close to where they are staying, and machine learning can help them decide which services to pursue and which to offer to each individual traveler.