Can Facebook’s Artificial Intelligence Kill Fake News?
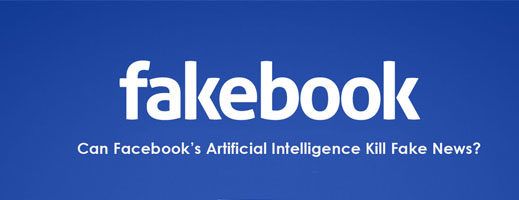
A exploration of the arms race to use Machine Learning by both those creating and stopping fake news.
“Man pardoned after shooting police officer”
“Clinton Foundation Behind California Wildfire”
“Caravan of Immigrants at Border Thought to Include ISIS Members”
***
If you live in certain places in the United States, these are the kinds of article titles that pervade your Facebook News Feed. In the last three years, social networks have experienced an explosion in false articles – or “fake news.” Facebook’s ability to eliminate this content from its platform is not only imperative for its survival, but will fundamentally shape our social fabric, politics, and collective belief in truth. Machine learning is at the heart of this task.
Fake news exists for two reasons. The first (and most publicized) is political gain, whether by domestic special interest groups or foreign governments. And second, according to Facebook Product Manager Tessa Lyons, “much of the false news we see on Facebook is financially motivated” [1]. Compensation for views gives content creators a powerful incentive to publicize click-bait in the form of fake news.
Because of the diversity, scale, and complexity of fake news, advanced Machine Learning techniques are necessary to identify it. In the last decade, “enormously increased data, significantly improved algorithms, and substantially more-powerful computer hardware” have ushered a renaissance in Machine Learning and Artificial Intelligence [2]. New techniques like deep neural networks and reinforcement learning show incredible results in topics traditionally thought to be hard or impossible for computers. According to MIT Professor Erik Brynjolfsson, “Ninety percent of the digital data in the world today has been created in the past two years alone” [2]. As the scale of social network data grows, monitoring cannot physically be done by humans. As described by Harvard Business School post-doctoral fellow Mike Yeomans, machine learning is a “branch of statistics, designed for a world of big data” – and sifting through the feeds of 2 billion Facebook users squarely fits this scope [3].
Facebook has already been highly successful in deploying machine learning in other places throughout its organization. For example, Facebook uses a tool called PhotoDNA to identify child pornography. Facebook’s algorithms have prompted over 1,000 calls to first responders after identifying users that may attempt suicide or harm themselves. And the natural language processing (NLP) system DeepText helped Facebook censor 2 million pieces of terrorist propaganda [4].
Looking forward, Facebook must employ similar techniques to address Fake News. In his testimonials before congress, CEO Mark Zuckerberg “references AI more than 30 times in explaining how the company would better police activity on its platform” [4]. However today, their algorithms are still powered by an army of fact checkers “who manually mark fake stories” [4].
When public criticism of Facebook intensified last spring, the company began devoting significant resources to external initiatives. They ran ads during the NBA Playoffs admitting mistakes and produced a thought-provoking 12-minute documentary called Facing Facts – on the challenges of fighting misinformation [5]. On the technical side, the company engaged Social Science One – a non-profit “partnership between academic researchers and private industry.” Through this collaboration, Facebook provides both funding and troves of anonymized internal data to academic researchers to pursue solutions to identifying fake news [6].
Sadly, Machine Learning isn’t just for the good-guys. A recent and exceptionally nefarious development is the use of machine learning to generate fake video (“deepfakes”). In this technique, a deep neural net (a common machine learning model) is fed hours of video of a person. Once trained, uses can produce video of that person doing or saying anything. In this way, machine learning can be used to create fake news.
The example video below shows a speech by Hillary Clinton, but delivered with President Donald Trump’s face and voice:
[7]
In July, Senator Mark R. Warner (Vice Chair of the Senate Intelligence Committee) published a white paper describing deepfakes as “poised to usher in an unprecedented wave of false or defamatory content” [8].
Machine learning can be used to create fake news, and it’s getting better, more difficult to detect, and easier to implement. Moreover, most people are unaware of deepfakes and would believe any video is a bonafide recording. Imagine deepfakes in a high profile election setting: millions of views would accrue before debunked, casting the legitimacy of the election into doubt [9]. Perhaps the worst side-effect of deepfakes is the destructive impact to the credibility of real news and real recordings.
Machine learning and AI are increasingly being used by companies to improve their product and police their platforms. However, these same methods are also available to those with more perverse objectives. How can Facebook get ahead of the curve in identifying fake news generated by machine learning programs? When censoring, how can they ensure political objectivity when so much content comes with a right-wing bend? Finally, how should Facebook change its approach to user content, censoring, and monetization?
(799 words)
______________________________
[1] Thompson, N. (2018). How Facebook Wants to Improve the Quality of Your News Feed. [online] WIRED. Available at: https://www.wired.com/story/how-facebook-wants-to-improve-the-quality-of-your-news-feed/ [Accessed 13 Nov. 2018].
[2] Brynjolfsson, E. and McAfee, A. (2017) ‘WHAT’S DRIVING THE MACHINE LEARNING EXPLOSION? Three factors make this AI’s moment’, Harvard Business Review Digital Articles, pp. 12–13. Available at: http://ezp-prod1.hul.harvard.edu/login?url=http://search.ebscohost.com/login.aspx?direct=true&db=bth&AN=124641872&site=ehost-live&scope=site (Accessed: 13 November 2018).
[3] Yeomans, M. (2015) ‘What Every Manager Should Know About Machine Learning’, Harvard Business Review Digital Articles, pp. 2–6. Available at: http://ezp-prod1.hul.harvard.edu/login?url=http://search.ebscohost.com/login.aspx?direct=true&db=bth&AN=118667151&site=ehost-live&scope=site (Accessed: 13 November 2018).
[4] Simonite, T. (2018). How Artificial Intelligence Can—and Can’t—Fix Facebook. [online] WIRED. Available at: https://www.wired.com/story/how-artificial-intelligence-canand-cantfix-facebook/ [Accessed 13 Nov. 2018].
[5] Neville, M. (2018). Facing Facts | Facebook Newsroom. [online] Newsroom.fb.com. Available at: https://newsroom.fb.com/news/2018/05/inside-feed-facing-facts-a-short-film/ [Accessed 13 Nov. 2018].
[6] Socialscience.one. (2018). Social Science One: Building Industry-Academic Partnerships. [online] Available at: https://socialscience.one/ [Accessed 13 Nov. 2018].
[7] Gilmer, D. (2018). A guide to ‘deepfakes,’ the internet’s latest moral crisis. [online] Mashable. Available at: https://mashable.com/2018/02/02/what-are-deepfakes/#ukmMTuyotqqb [Accessed 13 Nov. 2018].
[8] Warner, M. (2018). Potential Policy Proposals for Regulation of Social Media and Technology Firms. [online] Regmedia.co.uk. Available at: https://regmedia.co.uk/2018/07/30/warner_social_media_proposal.pdf [Accessed 13 Nov. 2018].
[9] Simonite, T. (2018). Will ‘Deepfakes’ Disrupt the Midterm Election?. [online] WIRED. Available at: https://www.wired.com/story/will-deepfakes-disrupt-the-midterm-election/ [Accessed 13 Nov. 2018].
Spencer – great essay. This is such a fascinating and meaningful problem given the polarity of the current political climate. I think your point about the erosion of trust in real media sources being a bi-product of the fake news phenomenon is spot on. Putting myself in Facebook’s shoes, I think it’s unreasonably aspirational to think they will catch all fake news before it can propagate across news feeds. That said, I think it is realistic to think that they should own the longitudinal responsibility of informing users of media they have interacted with which have been later flagged as fake. Whether it’s some sort of a notification several days later or otherwise, this may help lighten the burden they are putting on their AI technology.
One other thought is to what extent is it Facebook’s role to preserve political objectivity in this? Is a left-leaning AI fake news algorithm a bad thing? I think of more traditional businesses with explicit political or religious agendas (e.g. Chik-fil-A’s not open on Sunday policy) and wonder what’s different here. As a Facebook user, I tend to take for granted its utility as a communication tool, with little consideration that it is in fact a for-profit company with its own values, stances and perhaps social goals.
I loved this post – very poignant and interesting. Unlike Nick, I think it is important for Facebook to appear impartial in its efforts to detect/remove fake news articles from the site. However, I appreciate the assertion that many of the false stories are right-leaning and therefore if Facebook intervenes it will seem politically motivated. I wonder if it is possible for Facebook to be fully transparent with the community about its content-checking operations to both 1) educate the public on the power/applications of machine learning and 2) inspire confidence across the political spectrum that they are unbiased, yet serious about the implications of false content. As a user, I would love to feel like I am part of Facebook’s efforts to apply machine learning and would find confidence in knowing exactly how/what they are “censoring” from the site.
I agree with Allie that it makes strategic sense for Facebook to largely stay politically impartial (except in extreme circumstances when it believes it cannot compromise its values by not speaking out) since it is a mass market product.
Because of the inherent risk mentioned in this article about machine learning enabling bad hombres to create fake news just as much as it enables good hombres from stopping it, I wonder to what extent Facebook should publicly promise to be able to achieve the goal of stopping fake news (which it seems like they aren’t sure they’ll be able to do). I wonder what the other levers / pathways are in front of them — could they instead (or in addition) educate and empower their users to deduce fake news on their own?
Thanks for the essay Spencer! This is an important topic and a great use case for machine learning. Facebook has gotten into quite a bit of trouble recently over its lack of filtering when it comes to “fake news.” I think this case is mostly interesting because of the political implications behind the use of this form of machine learning. There are critics who I am sure would say that this sort of technology could be used not only to filter demonstrably fake news, but also to filter any sort of news that Facebook does not agree with politically.
There is also the interesting nuance that machine learning is being used on the other side of this equation as well. It is being used by people who are actively promoting fake news, so Facebook has the added challenge of combating this as well. Unfortunately, there are a number of challenges, and I’m not sure this is a problem that they can satisfactorily solve.
Great article Spencer! This article reminds me of the debate generated when Adobe revealed its excellent prototype for human voice manipulation that can generate speech through a very small set of voice recordings (See here: https://www.youtube.com/watch?v=I3l4XLZ59iw ).
The potential nefarious repercussions of such technologies are huge.
I also believe that Facebook has been mainly exploring cost conscious decisions (easy to scale and mainly requiring technology) to create its fact checking, whereas it could benefit from collaborating from international news agencies or create its own parallel structure to have an opinion on the veracity of information quickly.