Blue Apron: Can machine learning be effective in an omnichannel company?
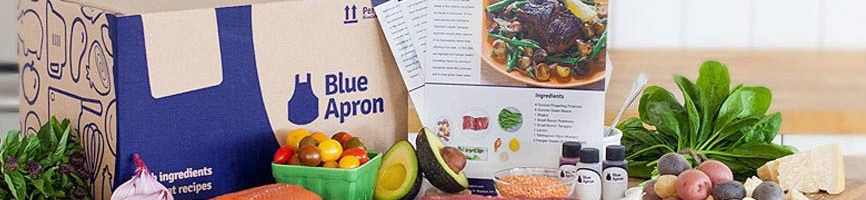
The business world is captivated by direct-to-consumer companies because of its fascination with machine learning. However, what if you cannot capitalize on this data and are forced to turn to wholesale channels for scale, therein “diluting” this data that made the company so attractive to begin with? Is machine learning from only a subset of customers still valuable and informative?
Blue Apron is one of the most high-profile direct-to-consumer companies due to its recent IPO. One of the first in its category, Blue Apron is a meal-kit delivery service with culinary-driven recipes and highest-quality ingredients[1]. Since its IPO in June 2017[2], Blue Apron has opened its books and therefore also itself up to public scrutiny over issues, such as the performance of its machine learning capabilities. Machine learning is used at Blue Apron for coordinating supply and demand, including “demand planning, recipe creation, recipe merchandising, and marketing.” Through website data and customer orders, it determines what behaviors drive demand and optimize its supply chain around these decisions. Data is also used to create, recommend and market recipes to customers, therein driving product development as well.[3]
Blue Apron has faced issues with the quality of its acquired customers though. The initial business model of “casting a wide net of acquiring a bunch of customers at the top of the funnel and hoping that many of them stick”[4] resulted in less profitable customers that dropped out of the subscription service very quickly. Data was not used to attract the most high quality customers.
To address this, Blue Apron has pushed to refocus machine learning on not just growing, but growing wisely: “we’ve really learned a lot over the last year or so on how to leverage the data and the knowledge we have about our current customer base and our lapsed customer base to really understand what a sticky or what a high-value customer to us is going to look like.”[5]
However, the recent entry into wholesale seems counter to this new “efficient” customer focus. In May and October 2018, it announced the selling of single-meal-kits in both Costco[6] and Jet.com respectively to “boost those sluggish sales.”[7] With this channel decision though was also the decision to begin losing customer data. The machine learning achieved through collecting online data – including click-through rate (ratio of users who click on a specific link versus viewing the page) or customer demographics – therefore started to become incomplete as customers in the Blue Apron ecosystem no longer were tracked in these wholesale channels. Wholesalers will not usually provide such detailed data to suppliers. An example of how this could be detrimental to Blue Apron’s focus on “sticky” customers would be if a customer cancelled the website subscription to then purchase from Costco. While this customer with a high lifetime value might be very worth acquiring, its machine learning will be blind to them because of its lack of visibility into wholesale channel purchasing.
While I do think the decision to go to wholesale channels was the right decision from a topline growth and profitability perspective, it does beg the question whether Blue Apron should have moved to the relative “black box” of selling through wholesale. Management should be focusing on understanding how to bridge the data gap between the channels as understanding the whole customer ecosystem will be paramount in its future sustainability, success and value as a company, especially as a company that had initially billed itself as an e-commerce company that excelled at using machine learning to drive and optimize demand.
Should Blue Apron have waited until a better system was in place to be able to track this data? And is there a scenario where customer data preservation for long-term machine learning optimization – in the pursuit of the “best” or most “efficient” customer – be prioritized over near-term growth and profitability?
(Word count: 796)
[1] Blue Apron, “Vision,” https://www.blueapron.com/pages/vision, accessed November 2018.
[2] Anita Balakrishnan and Sarah Whitten. “Blue Apron shares are basically flat in their first day of trading.” CNBC, June 29, 2017, https://www.cnbc.com/2017/06/29/blue-apron-ipo-opening-trade.html, accessed November 2018.
[3] Blue Apron Holdings, Inc. Amendment No. 4 to Form S-1 (filed June 28, 2017), https://www.bamsec.com/filing/104746917004287?cik=1701114, accessed November 2018.
[4] Bradley J. Dickerson, CEO, President & Director of Blue Apron, remarks made at Goldman Sachs 25th Annual Global Retailing Conference, September 5, 2018. From transcript provided by S&P Capital IQ, accessed November 2018.
[5] Timothy Bensley, CFO of Blue Apron, remarks made at Goldman Sachs 25th Annual Global Retailing Conference, September 5, 2018. From transcript provided by S&P Capital IQ, accessed November 2018.
[6] Hayley Peterson. “Costco now sells Blue Apron — and the meal kits are 30% off.” Business Insider, May 3, 2018, https://www.businessinsider.com/costco-sells-blue-apron-meal-kits-2018-5, accessed November 2018.
[7] Jenny Splitter. “Long-Suffering Blue Apron’s Latest Strategy: Partnering With Jet.com To Offer Single Meal Kits.” Forbes, October 29, 2018, https://www.forbes.com/sites/jennysplitter/2018/10/29/long-suffering-blue-aprons-latest-strategy-offering-single-meal-kits-for-same-day-delivery/#411a0a73353b, accessed November 2018.
Dear CrimsonCookies, this is a very interesting topic. While I agree with you that achieving a holistic understanding of the user journey across different distribution and marketing channels is fundamental, we should remind ourselves that machine learning and data are means to an end rather than the end goal itself. I don’t think a company like Blue Apron or any other digitally native company should avoid placement channels such as retail just because of the lack of data they would receive from the wholesalers. That would obviously preclude such companies from pursuing potentially great growth opportunities. And if companies required full transparency and data in order to invest in a growth channel, they would stop investing in television, print, influencer marketing (at some extent) etc. as those are also non fully trackable and measurable channels. I also believe waiting is not an option, especially when competition is as fierce as it is in the meal kit industry. Check for example this article, showing that HelloFresh, Blue Apron’s main competitor has also expanded into retail: http://fortune.com/2018/06/04/hellofresh-grocery-stores/. Assuming the relationships with these wholesalers are exclusive, then the first mover has a real advantage and leaving this money on the table doesn’t even seem to be an option even in the scenario in which it turns out that retail cannibalizes some of the online revenues (thinking of game theory principles).
Having said that, even if data is not complete, I think that there are still tremendous benefits for the company to invest in machine learning for product development and to better understand and predict at least the digital journey of customers. And some of those benefits can also be translated into competitive advantage in retail. For example, recipe development based on customer choices online can also help predicting the demand for those recipes in store.