Billionaire Robots: Machine Learning at Renaissance Technologies
Human traders may be a thing of the past – will machine learning and automation finally lead to the rise of a perfectly efficient market?
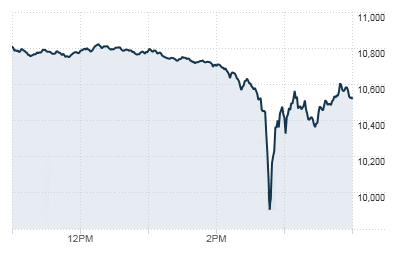
Michael Lewis chronicles the need for speed in his book “Flash Boys”, tracing the rise of high-frequency trading (“HFT”) as one of the most profitable public equity investment methodologies of the 21st century. Increasingly, however, trading speed is no longer sufficient to yield differentiated return – transaction time that was once measured in seconds is now monitored in nanoseconds. As more companies have built the technological infrastructure to compete in the HFT space and competitors have begun to scale, industry leaders have turned to predictive programming and, specifically, machine learning to enhance their ability to forecast stock movements to buy and sell accordingly. Renaissance Technologies (“RenTec”) is one of the most successful hedge funds to dominate the inception of quantitative trading, producing unrivaled returns of 71.8% annually from 1994 through mid-2014.1
Success in high-frequency trading was once solely based on geographic proximity to the NYSE and Nasdaq data centers in northern New Jersey; in fact, hedge funds would pay maintenance staff to place their servers slightly closer than their competitors’ (the staff later dubbed this “proximity services” or “colocation”).2 The arms race for speed intensified throughout the early 2010s, culminating in the construction of Spread Networks’ fiber-optics tunnel, which bridged Chicago and New York using low latency cable.3 By the mid-2010s, HFTs comprised approximately 50-60% of public equity trading by dollar volume.4 Trading speed became necessary but not sufficient to produce differentiated returns. Funds turned to algorithmic trading, which uses machine learning to “train” computer models on past price data in order to predict future price movements. Machine learning and artificial intelligence are particularly suited to trading due to the volume of information available for analysis; pricing models can be trained on extensive stock market data with signals ranging from news articles to historical price movements.5,6 Machine learning is being applied to liquid trading in two ways: first, using “reinforcement learning and neural networks” to increase the predictive power of the computer models, and second, to determine optimal portfolio allocation to different types of securities.7,8
RenTec has long been at the forefront of the deployment of machine and deep learning tools in trading. Its founder, James Simon, has focused on differentiating the firm by hiring investment professionals with doctoral degrees, particularly those with a science or mathematics background.9 Co-founder Howard Morgan explains, “[RenTec doesn’t] want people to have experience with trading; they want people who have math, stats, physics, biochemistry, or computer science backgrounds.”10 The firm has also focused on creating a work environment conducive to the open exchange of ideas, much more like a research lab than a traditional hedge fund. Simon himself explains, “no compartmentalization … you don’t get paid just on your work.”11 Recognizing that employees are the key asset for his firm, Simons has reduced employee turnover to almost zero using equity compensation incentives and lifestyle benefits.12 Unlike most other hedge fund managers, who grow assets indiscriminately due to fee structure incentives (many earn 2% of AUM regardless of fund performance), Simons also realized that there are diminishing returns to taking on capital due to constraints on deployment opportunities; the fund is capped at ~$10bn and is mostly restricted to employees.13
Although RenTec has been an undisputed leader in the emergence of quantitative trading, other funds are not far behind. As traditional barriers to entry have diminished due to cheaper trading infrastructure, new entrants have flooded the industry in search of outsized profits. Many have begun to mimic RenTec’s strategy of hiring non-finance PhDs. In order to preserve its competitive advantage, RenTec has begun and should continue to look at other asset classes and strategies – for example, they were one of the first to begin trading German futures.14 With each new asset class comes a new set of risks, which have often produced losses at other firms that have attempted to diversify. Unlike other strategies, quantitative trading does not require human intervention. Since the computers trade large volumes quickly, this can produce unexpected results. The 2010 “flash crash” sent some stocks plummeting or skyrocketing inexplicably only to bounce back moments later.15 RenTec should focus on honing monitoring systems that would prevent illogical and extreme trading results. In the wake of this catastrophe, regulators shown a spotlight on the previously unknown world of quantitative trading. RenTec should work to understand potential regulation that could significantly reduce the profitability of their strategies.16
- As more hedge funds master the art of machine trading and retail investors become increasingly passive, will we encounter a world in which even the most minor inefficiencies in the market have been arbitraged away? If so, could active trading on the equity markets eventually become obsolete?
- What type of regulation would be most effective to ensure that “flash crashes” do not happen in the future? Europe has considered a transaction tax – is this reasonable?
(Word Count: 800)
Citations
- “How an Exclusive Hedge Fund Turbocharged Its Retirement Plan”. Richard Rubin and Margaret Collins. June 16, 2015. https://www.bloomberg.com/news/articles/2015-06-16/how-an-exclusive-hedge-fund-turbocharged-retirement-plan.
- “Flash Boys: A Wall Street Revolt”, pg. 63. Michael Lewis. 2014.
- “Domeyard: Starting a High-Frequency (HFT) Hedge Fund”, pg. 3. Lauren Cohen, Christopher Maloy, and Matthew Foreman. April 1, 2015. Harvard Business School.
- “Domeyard: Starting a High-Frequency (HFT) Hedge Fund”, pg. 7. Lauren Cohen, Christopher Maloy, and Matthew Foreman. April 1, 2015. Harvard Business School.
- “The Massive Hedge Fund Betting on AI”. Adam Satariano and Nishant Kumar. September 27, 2017. https://www.bloomberg.com/news/features/2017-09-27/the-massive-hedge-fund-betting-on-ai.
- “The Rise of the Artificially Intelligent Hedge Fund”. Cade Metz. January 25, 2016. https://www.wired.com/2016/01/the-rise-of-the-artificially-intelligent-hedge-fund/.
- “When Quantitative Trading Meets Machine Learning: A Pilot Survey”. Yelin Li, Junjie Wu, and Hui Bu. June 24-26, 2016. IEEE Xplore Database.
- “Deep Neural Networks in High Frequency Trading”. Prakhar Ganesh. October 29, 2018. https://arxiv.org/pdf/1809.01506.pdf.
- “The Quiet Quant”. Melanie Rodier. June 2011. Wall Street & Technology, Vol. 29, Issue 3, pg. 22.
- “Renaissance Technologies Co-Founder Reveals Secrets to Quant and VC Career Success”. Dan Butcher. October 12, 2017. https://news.efinancialcareers.com/us-en/298218/renaissance-technologies-secrets-to-quant-hedge-funds-vc-career-success.
- “Jim Simons: My Hedge Fund’s Secrets Are Even Bigger Secrets Than The Work I Did For The Government”. Courtney Comstock. January 25, 2011. https://www.businessinsider.com/jim-simons-renaissance-mit-speech-video?IR=T.
- “The Secret World of Jim Simons”. Hal Lux. January 1, 2000. https://www.institutionalinvestor.com/article/b151340bp779jn/the-secret-world-of-jim-simons.
- “Inside a Moneymaking Machine Like No Other”. Katherine Burton. November 21, 2016. https://www.bloomberg.com/news/articles/2016-11-21/how-renaissance-s-medallion-fund-became-finance-s-blackest-box.
- “The Secret World of Jim Simons”. Hal Lux. January 1, 2000. https://www.institutionalinvestor.com/article/b151340bp779jn/the-secret-world-of-jim-simons.
- “The Massive Hedge Fund Betting on AI”. Adam Satariano and Nishant Kumar. September 27, 2017. https://www.bloomberg.com/news/features/2017-09-27/the-massive-hedge-fund-betting-on-ai.
- “Into the Breech: The Increasing Gap between Algorithmic Trading and Securities Regulation”. Karen Kunz and Jena Martin. October 31, 2013.
Great article, and always fun to hear about a firm that is able to “beat” the market.
I actually have a question in response to one of your questions. You ask what type of regulation would be most effective at preventing flash crashes in the future. My question back to you is: why do we care about flash crashes? Presumably the algorithms will adapt (organically or through human intervention) to profit off of, and prevent, future flash crashes. And even if the algorithms don’t adapt, and we keep having these crashes – what’s wrong with that? The crashes happen very infrequently and self-correct very quickly, so it’s not as if they destroy investor confidence in the stock market. And regulation rarely has no negative side effects…