BenevolentAI: Can artificial intelligence drive the next generation of drug development?
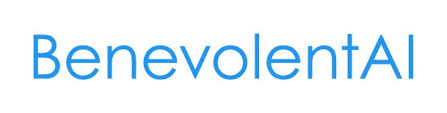
BenevolentAI is a UK-based biotechnology company betting on machine learning and artificial intelligence as their competitive advantage at all stages of the drug development process. Will this bet pay off or will BenevolentAI fail to create meaningful innovation in the biotech space?
The average cost of developing a prescription drug is 2.6 billion USD and often takes over a decade [1]. These prohibitively large numbers translate into real morbidity and mortality for patients. A McKinsey Global Institute study estimated that applying big data strategically in healthcare can save up to 100 billion USD every year in the US healthcare system [2].
BenevolentAI is a small biotechnology company based out of London that uses biomedical data, artificial intelligence (AI) and machine learning to improve all levels of drug development: basic science, target identification, molecular design, and clinical outcomes [3]. While pharmaceutical companies have attempted to integrate AI into their drug delivery pipeline at various steps, BenevolentAI is unique for betting on AI as their core competitive advantage in all stages drug discovery.
The first stage of drug discovery is dependent on basic science and an understanding of the scientific foundations of disease. BenevolentAI use a “comprehensive bioscience knowledge graph” that through machine learning is able to find patterns in the scientific literature. The company’s hypothesis is that scientific leads found through machine learning are generated faster and are higher quality [3].
The second stage of drug discovery is finding the compound or technology to tackle the pathology responsible for disease. BenevolentAI leverages its core technology to select and design molecules to fit both the specific target and the patient. This method should allow computational scientists to recommend a small subset of candidate molecules for laboratory scientists to test with higher efficiency, leading to “fast, iterative experimental cycles” [3].
The final stage of drug discovery is clinical testing. BenevolentAI is using its machine learning methods to input different characteristics about patients to try to predict clinical response and side effects. In this way, the company hopes to lead the revolution for personalized medicine by making never before seen connections between a patient’s genotype and other characteristics and reactions to new pharmaceuticals. Their platform analyzes previous trials to help inform which patients may respond best to a drug and to choose the best clinical trial candidates.
BenevolentAI is currently prioritizing ALS, amyotrophic lateral sclerosis, and has claimed that its AI techniques yielded a breast cancer drug delayed disease onset in an animal model [4]. The drug is currently being optimized using EvoChem, a tool that generates and test novel compounds in silico to decrease the number of costly and slow laboratory.
Other projects include age-related macular degeneration and a broader look at the drug development space through ClinicalTrials.gov [5,6]. They found that the design and dissemination of trials are different depending on the disease area and whether the funding is public or private. These results are not only academically interesting, they also offer a way to more efficiently and fairly identify unmet needs in drug development.
Although these technologies offer huge potential in drug discovery, there are important caveats and considerations. As the scientists at BenevolentAI write, such a complex process as drug discovery cannot be solved by one AI platform [7]. Rather it takes many different algorithms at different steps in the pipeline to create even a single new drug. The company runs the risk of playing it safe after creating one functional pipeline. Human disease is complex and exceedingly diverse. Different organ systems and types of pathology will require different technological solutions. Therefore, BenevolentAI should focus on developing a broad range of AI solutions that are specific to each disease area they are attempting to tackle.
In addition, BenevolentAI claims that their platform is “designed to counter bias,” a controversial claim. Computational algorithms are only as good as the data that is fed into it. One could argue that peer-reviewed scientific literature is as close as one can get to an unbiased database, but clinical trial data on the other hand is highly biased. Not only is there underrepresentation of certain ethnic minorities and pregnant women, the presentation and characteristics of disease in trials are often highly biased by design. Therefore, it would be my strong recommendation that BenevolentAI invest in obtaining more natural history disease data from all patients, especially underrepresented minorities. It is only with a more complete dataset that they can accomplish their mission of using AI to truly personalize medicine.
Even as companies like BenevolentAI lean full-force into AI for drug development, questions remain. Will the AI and machine learning technology they are using allow for truly innovative discovery of molecules and biological technology or will its main purpose be repurposing old drugs for new uses, such as the breast cancer drug for ALS? And more importantly, in a field so deeply related to people as medicine and drug development, is it possible to develop AI to create personalized medicine that doesn’t carry the biases of their human creators?
(790 words)
[1] Rick Mullin. “Cost to Develop New Pharmaceutical Drug Now Exceeds $2.5B.” Scientific American: Chemical & Engineering News. 24 November 2014.
[2] Jamie Cattell, Sastry Chilukuri, and Michael Levy. “How big data can revolutionize pharmaceutical R&D.” McKinsey and Company. April 2013 https://www.mckinsey.com/industries/pharmaceuticals-and-medical-products/our-insights/how-big-data-can-revolutionize-pharmaceutical-r-and-d.
[3] “About us.” BenevolentAI https://benevolent.ai/about-us.
[4] “Why it matters.” BenevolentAI https://benevolent.ai/why-it-matters/.
[5] “BenevolentAI and group of UK charities partner to find a cure for blindness.” Benevolent AI https://benevolent.ai/news/announcements/benevolentai-and-uk-charities-partner-to-find-a-cure-for-blindness.
[6] Magdalena Zwierzyna, Mark Davies, Aroon Hingorani, and Jackie Hunter. “Clinical trial design and dissemination: comprehensive analysis of clinicaltrials.gov and PubMed data since 2005.” The British Medical Journal. 19 April 2018. 361:k2130.
[7] Matthew A Sellwood, Mohamed Ahmed, Marwin HS Segler & Nathan brown, “Artifical intelligence in drug discovery” Future Medicinal Chemistry. 13 August 2018. 10(17): 2025 – 2028.
Hi JP, I think the innovation in drug discovery using AI is extremely challenging, given the current data available for drug development. In addition to selection bias of trial data you already mentioned, a key issue in current BenevolentAI approach is the lack of negative results. The fact that scientists/clinical trials rarely if ever report negative results leads to massive survivorship bias that hypothetically at best would produce drugs with similar/same mechanisms of action and at worst waste precious resources by leading people into a search field of molecules that have already been proven not to work. I’m really curious to see how this field will develop in the future as it becomes less historic data dependent and more simulation driven. I think that a lot of exciting possibilities will emerge facilitated by the exponential growth in processing power and the possible arrival of quantum computing.
Thanks for the thoughtful post, JP. It seems Benevolent AI’s decision to use machine learning as a competitive advantage throughout all stages of the drug development lifecycle is certainly unique – and brings with it certain questions as to whether the AI technology will become “good enough” that it can create personalized medicine absent the biases of human creators. I think your suggestion – to invest in obtaining more natural history disease data from all patients, especially underrepresented minorities – is a good one, and importantly one that can be addressed over time and in parallel (i.e., while the company continues to build out its AI algorithms for known population sets). Awareness of the biases inherent in the available data sets is an important first step in making sure BenevolentAI is able to create personalized medicine for all people, and I would hope the company is vigilant in combating other biases – for instance, making sure there are sufficient results in “unbiased” peer-reviewed scientific literature that tackle under-represented populations – inherent in the data sets it is collecting.