An Apple a Day Keeps Premiums at Bay: AI at Discovery Ltd.
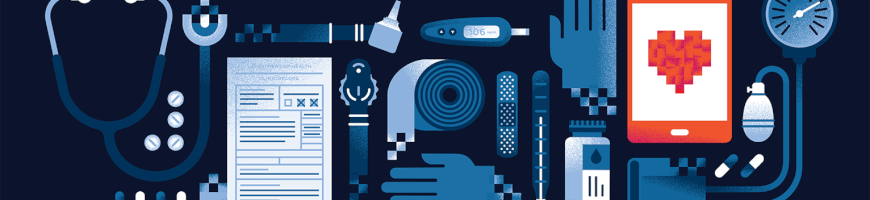
Exploring the trajectory and potential of machine learning in incentive-based insurance at Discovery Ltd.
Discovery Ltd. (JSE/DSY) is a pioneer in shared value, incentive-based health and other personal insurance. The innovative South Africa-based insurance provider has a market cap of approximately USD 7 billion and nearly 10 million clients worldwide [1], [2]. It differentiates through unique access to client data via patented platforms, personalized insurance offerings and noteworthy fintech partnerships [3]. Discovery is a global leader in using data from wearables to incentivize healthy behavior, lowering member costs through lower premiums and financial rewards [4].
Machine learning has significant potential in insurance as the industry is fundamentally data-intensive [5]. A key concern in the industry is the sheer volume of data; insurers increasingly look to machine learning to process data and extract insights [6]. Machine learning is important for insurance companies, and Discovery in particular, due to its potential to achieve higher efficiency, larger scale and enhanced client cost/benefit ratios. Three crucial applications in insurance are: i) claims processing and fraud prevention – processing data faster while minimizing error; ii) customer service – serving a broader base of clients more cost effectively; and iii) developing policies and pricing – personalizing offerings at scale using behavioral risk analysis and incentive-based pricing [5]. While process improvement applications are critical, this study largely focuses on product development application (iii), given the context of Discovery’s behavioral, incentive-based insurance.
Discovery’s patented data platforms are well-positioned for machine learning. Discovery’s Vitality program in South Africa has over 1.8 million clients uploading data on physical activity and health [1]. Discovery’s Insure program has 172,000 auto insurance policies with over 1.6 billion kilometers of driving data from real-time smartphone tracking [7]. Discovery’s management recently introduced machine learning to improve customer service, affirming the importance of AI in operations. The DrConnect app and Ask Discovery chatbot, launched in 2017, learn progressively from client interactions to provide better solutions/diagnoses. Figure 1 displays three of the interactive data platforms described above.
Figure 1: Discovery’s Vitality, Insure and DrConnect platforms [8], [9], [10]
In the short term, Discovery is forging partnerships with fintech/tech companies that have machine learning capabilities. In 2014, Discovery partnered with Cambridge Mobile Telematics (CMT), a U.S. company with core machine learning capabilities, to develop an application that uses driver performance to define appropriate auto insurance policies [11]. This year (2018), Discovery and CMT partnered with SqwidNet, a South African company providing nationwide IoT coverage, to roll out this application further [12]. In 2018, Discovery partnered with Shapa, a company focused on behavioral health technology. The partnership will introduce a U.S. weight-management solution, linked to health insurance, using machine learning to propose personalized weight goals [13]. By partnering with fintech/tech firms, Discovery’s management can rapidly leverage products developed using machine learning, cementing Discovery’s competitive position in key markets.
Discovery’s management has medium-term plans to integrate machine learning into business operations. Discovery Business Insurance was launched this year to provide an insurance product for small business in South Africa, with the premise of using advanced technology to monitor business risks [14]. This technology includes thermal-imaging drones for applications such as building aging analysis and smart 3D glasses to be used by on-site engineers. In the medium-term, Discovery intends to combine machine learning with this technology to improve forecasting and risk management.
A key issue with machine learning in healthcare applications is the phenomenon of strong mistrust among clients, typically attributed to known instances of failure, lack of familiarity and an instinctive preference for human judgement [15]. This could pose challenges to AI adoption, if customers are reluctant to share personal data. In the short term, it is recommended that Discovery’s management undertake a detailed public information campaign to combat trust issues. This should create awareness of back-end processes and data confidentiality, and inform customers of the benefits of machine learning. The campaign should provide transparency on data use with the clear goal of customer well-being.
Over the short- to medium-term, it is recommended that Discovery: i) invest in machine learning capabilities for process improvement, such as in claims processing and fraud detection, to improve cost-effectiveness; ii) integrate data/knowledge across products, creating an organization-wide information base to better tailor individual policies; and iii) develop a platform to share lessons from machine learning across geographies.
While Discovery has made important progress in machine learning, there is still a long road ahead to succeed in this space. Should Discovery invest in in-house machine learning capabilities, or continue to partner going forward? Discovery’s path will partly be shaped by its primary South African market, where it aims to offer lowest cost, accessible insurance for “all South Africans” [1]. Despite this, in South Africa about 50% healthcare spend represents only 16% of the population who can afford private health insurance [16]. This begs the question: is machine learning going to improve accessibility or simply worsen the gap to insurance for low-income groups?
(800)
References
[1] Discovery Ltd. (2017). Integrated Annual Report. Available at: [https://www.discovery.co.za/marketing/integrated-annual-report/?page=1]. Accessed November 2018
[2] Financial Times. (2018). Equities: Discovery Ltd. Available at: [https://markets.ft.com/data/equities/tearsheet/profile?s=DSY:JNB]. Accessed November 2018
[3] Adrian Gore – Discovery Holdings Limited. (2018). SA Entrepreneurs. Available at: [http://saentrepreneurs.co.za/adrian-gore-discovery-holdings-limited/]. Accessed November 2018
[4] Institute of International Finance. (2016). Innovation in Insurance: How Technology is Changing the Industry: pg. 8. Available at: [https://www.iif.com/system/files/32370132_insurance_innovation_report_2016.pdf]. Accessed November 2018
[5] Marr, B. (2017). How AI And Machine Learning Are Used To Transform The Insurance Industry. Forbes. Available at: [https://www.forbes.com/sites/bernardmarr/2017/10/24/how-ai-and-machine-learning-are-used-to-transform-the-insurance-industry/#67345be913a1]. Accessed November 2018
[6] Cheatham, C. (2018). Machine Learning From the Insurance Trenches. Wells Media Group, Inc. San Diego. United States. Available at: [https://search-proquest-com.ezp-prod1.hul.harvard.edu/docview/2022522310?accountid=11311]. Accessed November 2018
[7] Infographic: Discovery Insure and Cambridge Mobile Telematics – Insights into driver behavior. (2014). Available at: [http://www.mynewsdesk.com/za/discovery-holdings-ltd/documents/infographic-discovery-insure-and-cambridge-mobile-telematics-insights-into-driver-behaviour-38222]. Accessed November 2018
[8] Discovery Ltd. (2014). Discovery Insure App Screenshots. Available at: [http://www.mynewsdesk.com/za/discovery-holdings-ltd/images/discovery-insure-app-screenshots-297784]. Accessed November 2018
[9] DrConnect by Discovery Ltd. (2018). Available at: [https://appadvice.com/app/drconnect/1233996971]. Accessed November 2018
[10] LTC. (2016). Where to next for Discovery Vitality Active Rewards? Available at: [http://leavethecouch.com/where-to-next-for-discovery-vitality-active-rewards/]. Accessed November 2018
[11] Discovery Ltd. (2014). Leading global insurer expands partnership with innovative telematics technology provider. Dow Jones Institutional News. New York. Available at: [http://search.proquest.com.ezpprod1.hul.harvard.edu/docview/2074777307?accountid=11311]. Accessed November 2018
[12] Budholiya, A. (2018). Discovery Insure Teams up with SqwidNet for a Wider Portfolio of Technology & Telematics Offerings. Available at: [https://www.technorhythms.com/discovery-insure-teams-up-with-sqwidnet-for-a-wider-portfolio-of-technology-telematics-offerings/]. Accessed November 2018
[13] Vitality Group. (2018). Leading Behavioral Economist Dan Ariely’s Shapa Teams Up with Vitality to take on US Healthcare Challenges. Available at: [https://www.marketwatch.com/press-release/leading-behavioral-economist-dan-arielys-shapa-teams-up-with-vitality-to-take-on-us-healthcare-challenges-2018-08-15]. Accessed November 2018
[14] de Wet, P. (2018). Discovery’s new business insurance unit will assess risk using thermal-imaging drones and remote advisors. Business Insider SA. Available at: [https://www.businessinsider.co.za/discovery-business-insurance-will-use-drones-thermal-cameras-and-virtual-reality-to-check-risks-2018-5]. Accessed November 2018
[15] Polonski, Dr. V. (2018). Humans Don’t Trust AI Predictions – Here’s How to Fix It. OECD. Available at: [https://www.oecd-forum.org/users/80891-dr-vyacheslav-polonski/posts/29988-humans-don-t-trust-artificial-intelligence-predictions-here-s-how-to-fix-it]. Accessed November 2018
[16] Barbera, S. L., Kumarb, A., Roubalc, T., Colombod, F., Lorenzonida, L. (2018). Harnessing the private health sector by using prices as a policy instrument: Lessons learned from South Africa. Elsevier. 122: 558–564. Available at ScienceDirect, accessed November 2018
[17] (reference for cover picture) The Medical Futurist. (2018). What To Expect From Digital Health In 2018? Available at: [https://www.google.com/url?sa=i&rct=j&q=&esrc=s&source=images&cd=&ved=2ahUKEwjNkNL0zNLeAhWxmOAKHUUsCm4Qjhx6BAgBEAM&url=https%3A%2F%2Fmedicalfuturist.com%2Fwhat-to-expect-from-digital-health-2018&psig=AOvVaw1nNmr0UIOvCsdkfRvmf3oZ&ust=1542240821894275]. Accessed November 2018
Found this article about insurance in South Africa very fascinating! It was interesting to see all of the parallels between the insurance system in the US and in S. Africa – machine learning is used for similar reasons and fight a similar problem wherein a small percentage of the cared-for population contributes to the majority of healthcare spending. Because of this, I feel like Discovery definitely needs to begin to invest in their own in-house machine learning / AI capabilities. If healthcare trends are similar in SA (rising healthcare costs and an aim to be more accessible) then it is all the more reason to utilize this technology to target and intervene in the right populations efficiently.
A report by Accenture says that most insurance companies use 10-15% of the data they have access to (https://www.accenture.com/t20180822T093440Z__w__/us-en/_acnmedia/PDF-84/Accenture-Machine-Leaning-Insurance.pdf). Machine learning / AI can allow these companies to better target patients, design personalized marketing and interventions, and have more strategic pricing and risk assessments. If Discovery can leverage this megatrend, then this means that they should be able to better serve low-income groups, so long as the low-risk groups continue to be a part of the risk pool.
You addressed my biggest concern as soon as it came to mind, namely, privacy and trust. I love the idea of using AI to lower insurance premiums through behavioral incentives, making it more accessible and affordable. The two examples that come to mind that have succeeded here in the U.S. (not using AI, but with similar goals) were Progressive’s SnapShot program in car insurance (https://www.progressive.com/auto/discounts/snapshot/), and Safeway’s in-house health insurance plan (https://www.wsj.com/articles/SB124476804026308603). In the first, drivers can opt into the program to have their driving monitored. If they drive well while monitored, their rates go down. In the second, employees pay lower premiums for good results on health tests. The benefits are clear. The insurance companies incentivize good behavior through lowered premiums. As a result, people get in less accidents or live healthier, lowering the costs for the consumer and the provider. If the companies can convince people that their data is secure, or use opt-in programs that give them the choice to use their data or not, this will be a significant step in the right direction for healthcare and other types of insurance.
Interesting application! I think the advantage of using ML for insurance claims and fraud detection are can be very impactful and effective in both reducing the cost structure of insurance companies and reduce the risk exposure of their funds. If the data set and ML processes are designed effectively to increase the customer satisfaction with the service, this could be a significant competitive advantage for Discovery.