A Use Case for Machine Learning: How Facebook Uses Machine Learning to Combat Fake News
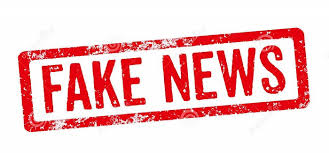
This paper will focus on Facebook’s use of machine learning to manage political content on its site. Today, with platforms like Facebook, content is being generated by a wider range of sources, which has eroded the credibility of the political information on Facebook. Recently, we have seen this occur with the proliferation of “fake news”, specifically falsified political information. This development has significant implications for Facebook and risks alienating its user based which can impact its bottom line and user base. It is Facebook’s mission to create a constructive community that brings people together to create positive experiences. False news is “harmful to [their] community” and “makes the world less informed” which inherently “erodes trust” with its users. In this context, using machine learning and other statistical tools to identify inaccurate and manipulated information is paramount to Facebook’s efforts to combat the spread of such information.
Machine learning enables companies to organize and analyze an enormous scale and complexity of data. Facebook has 2.27 billion active users generating an incredible amount of data.[1] Therefore, machine learning is particularly relevant to Facebook because its gives Facebook the tools to analyze the large volume of posts and determine how relevant they are to individual users. With that information, Facebook can rank relevancy and order of posts that show in a user’s feed, thus creating the most valuable and engaging user experience. More recently, it has become an increasingly important platform for political campaigning. This paper will focus on Facebook’s use of machine learning to manage political content on its site. Today, with platforms like Facebook, content is being generated by a wider range of sources, which has eroded the credibility of the political information on Facebook. Recently, we have seen this occur with the proliferation of “fake news”, specifically falsified political information. This development has significant implications for Facebook and risks alienating its user based which can impact its bottom line and user base. It is Facebook’s mission to create a constructive community that brings people together to create positive experiences. False news is “harmful to [their] community” and “makes the world less informed” which inherently “erodes trust” with its users.[2] In this context, using machine learning and other statistical tools to identify inaccurate and manipulated information is paramount to Facebook’s efforts to combat the spread of such information.
In the near term, Facebook has expanded its fact checking capabilities in order to identify fake news. Facebook uses a combination of machine learning and human labor in order to do this. There is limited information on how exactly Facebook structures its models, but its algorithms likely “analyze the way a [post] is written, and tell you if it’s similar to an article written with little to no biased words, strong adjectives, opinion, or colorful language”.[3] This is a complex task because it is very difficult to characterize what defines news as “fake”, as this process entails a high degree of subjectivity. Facebook uses previously debunked stories to further inform its algorithms, and allows its users to report fake news as well.[4] Once Facebook identifies a potential piece of fake news, it sends it to its network of fact checking partners, such as Schema.org, who then verify it.[5] If flagged as fake, Facebook will show that post lower in its users’ news feeds to limit its impact. Facebook will also use machine learning to identify the domains spreading that news and limit their distribution.[6] In July of 2018, Facebook acquired Bloomsbury AI whose natural language processing capabilities, it is believed, will be put towards the challenges detailed above.[7] In addition, Facebook is partnering with academic institutions to further improve their algorithms.[8]
Politically charged content can come from many different sources. It can come from organic user content and from paid sources like true political campaigns. While the former is particularly difficult to manage, I think one area Facebook can improve in the near-to-medium term is increasing transparency among paid political content. In May of 2018, Facebook instituted a mandatory “Paid For” disclosure for any ad relating to politics.[9] While this is a step in the right direction, it is clear that Facebook needs to improve their process for approval for these paid ads. Recently, media outlet Vice News conducted an experiment in which it successfully created and ran ads that falsely claimed to be “Paid For” by ISIS, Vice President Mike Pence, and Democratic National Committee Chairman Tom Perez.[10] This is a clear indicator that the review and approval process of paid ads needs to be thoroughly audited and improved by Facebook.
Is the public sector ultimately responsible for regulating political messaging online?
Word Count: 794
[1] Facebook Newsroom, [https://newsroom.fb.com/company-info], accessed November 2018.
[2] Adam Mosseri, “Working To Stop Misinformation and False News,” Facebook for Media (blog), Facebook, April 7, 2017, [https://www.facebook.com/facebookmedia/blog/working-to-stop-misinformation-and-false-news], accessed November 2018.
[3] Aaron Edell, “I trained fake news detection AI with >95% accuracy, and I almost went crazy,” Towards Data Science, January 11, 2018, [https://towardsdatascience.com/i-trained-fake-news-detection-ai-with-95-accuracy-and-almost-went-crazy-d10589aa57c], accessed November 2018.
[4] Tessa Lyons, “Increasing Our Efforts to Fight False News”, Facebook Newsroom (blog), June 21, 2018, [https://newsroom.fb.com/news/2018/06/increasing-our-efforts-to-fight-false-news/], accessed November 2018.
[5] ibid
[6] ibid
[7] Patrick Kulp, “Facebook Hires Team Behind AI Startup in Battle Against Fake News”, Adweek, July 3, 2018, [https://www.adweek.com/digital/facebook-hires-team-behind-ai-startup-in-battle-against-fake-news/], accessed November 2018.
[8] Tessa Lyons, “Increasing Our Efforts to Fight False News”, Facebook Newsroom (blog), June 21, 2018, [https://newsroom.fb.com/news/2018/06/increasing-our-efforts-to-fight-false-news/], accessed November 2018.
[9] Sean Wolfe, “Facebook approved 100 fake ad disclosures that were allegedly ‘paid for’ by every United States senator,” Business Insider, October 30, 2018, [https://www.businessinsider.com/facebok-fake-ads-election-senators-2018-10], accessed November 2018.
[10] Sean Wolfe, “Facebook approved fake political ads that claimed to be paid for by ISIS and Mike Pence,” Business Insider, October 26, 2018, [https://www.businessinsider.com/facebook-approved-fake-political-ads-isis-mike-pence-report-2018-10], accessed November 2018.
It was interesting to learn more about how Facebook uses machine learning to identify fake news. I like your idea that Facebook be more transparent about what political content is paid. I do think the public sector has a responsibility to provide some guide rails about political messaging online, but I do think it should not be unnecessarily prescriptive.
This is a very relevant and interesting issue. Machine learning works well in improving when there are measures by which the algorithm can learn and improve by. This case presents a challenge as articles written as speculation or opinion will be hard to differentiate from factual news articles, and may even be subjective. It will be interesting to see how this nuance is handled as the algorithms further mature.
It’s interesting in the current context to think about the implementation of machine learning at facebook to identify fake news. However I doubt about the potential success of this application since there is a degree of subjectivity inherent to any media. French news media has already sprung into action by opening a fact checking service to stop fake news items in their tracks and I believe that as of today human control is the only viable solution. Of course as the volume of data grows bigger with time the chance of handling misinformation will become challenging for humans and hopefully machine learning will keep improving and prove itself efficient.
It’s a good article to show one of the various applications of machine learning that is not necessarily obvious to many people. As mentioned in previous comments, I think the challenge here is whether it’s easy and clear enough for a machine to learn how to tell fake news. Given inherent subjectivity of telling politically fake news, I am not sure how quickly Facebook will be able to develop a mechanism to tell fake news using machine learning. Also, when we think about scalability of the application (i.e., rolling out the application to different languages), it may take longer to fully apply the application to all the news posted and shared in different languages. But, I personally look forward to seeing one day when Facebook tells me that I’d better take a caution with reading fake news.