A Better Brain: Machine Learning in Guided Meditation
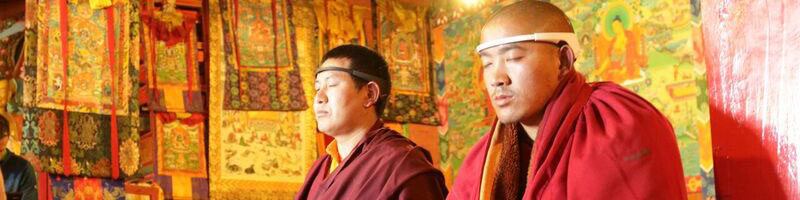
Meditation, with its many mental benefits, has remained an elusive skill to master. While there has been a surge in the number of guided meditation mobile apps available, none offer any real insight to the most common question that beginner meditators have – “Am I doing it right?”.
Meditation, with its many mental benefits, has remained an elusive skill to master. While there has been a surge in the number of guided meditation mobile apps available, none offer any real insight to the most common question that beginner meditators have – “Am I doing it right?”.
Muse’s flagship product, the Muse headband, is a consumer-grade electroencephalogram (EEG) device that provides real-time neurofeedback during meditation. The headband houses seven electrodes that sits on the forehead and behind the ears. When used, Muse senses electrical activity in the brain and records brain wave patterns. This data is then sent via Bluetooth to a smartphone, where it is translated into weather soundscapes. When the user’s mind is calm, the user will hear serene weather soundscapes, such as gentle rain in a tropical rainforest. Conversely, when the brain wave patterns reflect a busy mind, the user will hear chaotic sounds, such as that of rush hour traffic. By providing real-time feedback, Muse is designed to takes the guesswork out of meditation.
A big challenge that Muse faces is to be able to accurately classify the user’s brain states as representative of either focused attention or distraction[1]. Arguably, the most straight-forward way to do this is to run the input EEG data through a statistical model to generate an attention-distraction score. However, this statistical approach yields inaccurate results because there is a significant amount of variation in brainwave patterns across individuals for any given brain state[2]. Comparing the user’s brainwaves to a normative benchmark of what “calm” brainwaves look like tends to lend itself to inaccuracies. Thus, the way forward for product development at Muse is to create individualized brainwave signatures for each user through machine learning.
In order to make the classification of brain states more accurate through machine learning in the short term, Muse has introduced more dimensions of data collection with their newest headband, the Muse 2. The new headband includes photoplethysmography (PPG) sensors that use optical-based technology to detect the user’s heart rate and breathing patterns. Muse 2 also features an accelerometer, which allows Muse to omit brainwave data points that have been affected by the user’s head movements.
In the medium-term time horizon, Muse has started to move away from its positioning as a retail consumer-grade device to an economical alternative to medical-grade EEG devices used in research. Muse has released a software development kit to allow users to download the raw EEG data. The company also created a free visualization tool called MuseLab that lets users plot customized EEG data as the device is being used. By opening up the backend functionalities of the Muse, research institutions are able test the efficacy of machine learning methods on the recognition of mental states. One notable example has been a recent study[3] done by researchers at the University of Memphis and IBM Watson Research Center on discriminating between logical and emotional mental states by applying machine learning to data collected with Muse.
However, despite adding more dimensions for collecting data and opening up the backend to developers, there is still a fundamental aspect of the Muse that needs to be changed to address the growing importance of machine learning. The Muse headband requires an interface that allows users to self-report mental states to construct a training dataset for the machine learning algorithms to work with. This opens the doors to the deployment of a self-calibrating protocol[4] that is user-specific in its ability to distinguish between a calm, settled mind and a busy, wandering one.
In addition, there are serious privacy issues that need to be considered if Muse were to collect the brainwave data of its users to draw insights on the differences between brain morphologies. While perhaps justified in its intentions to use the data to create a more effective meditation learning tool, consumers will undoubtedly protest the collection of the distillation of their very thoughts, the sort of data that is far more intimate than age, race or gender. As such, Muse should avoid this ethical pitfall altogether by keeping brainwave data stored locally on the users’ smartphones.
There remains several key open questions about the use of machine learning in guided meditation:
- Will the use of technological aids such as Muse stunt people’s potential in meditation? Perhaps the traditional method of learning meditation through trial and error is much slower, but could actually be a better path to take in the long run.
- Can the use of EEG-derived brainwave data be applied in other fields such as consumer insights?
- What other potential applications of using machine learning to identify individualized brainwave signatures are there? Mind-controlled wheelchairs for paraplegics?
(775 words)
Very interesting product that Muse has released. As far as stunting the growth of meditators, I hardly think the Muse headband would be any sort of hindrance to serious practitioners; I do not believe they would use it in the first place. It takes years and years of training to even come close to mastering the art of meditation, and these people would be very skeptical of such an invention. This seems like more of a product for novice meditators who could use it in the beginning stages of their meditation practice to augment their normal meditation routine.
It the future if a technology similar to the Muse headband could be implanted or otherwise worn continuously without annoyance, it would be able to send real time mental data to a central location. AI could analyze this data and perhaps predict events and avert things such as national security threats before they occur. A bit big brother-ish, but possible.
I agree with Scipio’s post above – I don’t see this product gaining much traction with real practitioners of meditation. I’m also skeptical that beginners will be eager to buy it as a training tool. There are several meditation apps that provide guided meditations for free, and do so in a surprisingly organic and non-intrusive way. In my opinion, this product defeats one of the main purposes of meditation – to practice mindfulness by exercising our ability to self-identify when our minds wander.
The technology could be applied to a host of other applications, especially those in the medical field.
I agreed with both posts above – I am very skeptical with the commercial value of this product based on its functionality.
Even if machine learning technology enables Muse headband to correctly identify each user’s state of focused attention or distraction based on his/her individual brainwaves pattern, one big question remains unresolved: just the mere fact of being in the state of focused attention, does that mean the person perform meditation correctly and gain all the benefits associated with it? One can argue that when a HBS student puts focused attention in classroom to understand difficult TOM concept, it is certainly not the same state as “the higher state of consciousness” that well-performed meditation can generate.